Abstract
Purpose
Following the imperative for human-centric digital innovation brought by the paradigm of Industry 5.0, the article aims to integrate the dispersed and multi-disciplinary literature on individual risks for workers to define, explain and predict individual risks related to Industry 4.0 technologies.
Design/methodology/approach
The paper follows the question, “What is the current knowledge and evidence base concerning risks related to Industry 4.0 technologies, and how can this inform digital innovation management in the manufacturing sector through the lens of the Industry 5.0 paradigm?” and uses the method of systematic literature review to identify and discuss potential risks for individuals associated with digital innovation. N = 51 contributions met the inclusion criteria.
Findings
The literature review indicates dominant trends and significant gaps in understanding risks from a human-centric perspective. The paper identifies individual risks, their interplay with different technologies and their antecedents at the social, organizational and individual levels. Despite this, the paper shows how the literature concentrates in studying risks on only a limited number of categories and/or concepts. Moreover, there is a lack of consensus in the theoretical and conceptual frameworks. The paper concludes by illustrating an initial understanding of digital innovation via a human-centered perspective on psychological risks.
Practical implications
Findings yield practical implications. In investing in the adoption, generation or recombination of new digital technologies in organizations, the paper recommends managers ensure to prevent risks at the individual level. Accordingly, the study’s findings can be used as a common starting point for extending the repertoire of managerial practices and interventions and realizing human-centric innovation.
Originality/value
Following the paradigm of Industry 5.0, the paper offers a holistic view of risks that incorporates the central role of the worker as crucial to the success of digital innovation. This human-centric perspective serves to inform the managerial field about important factors in risk management that can result in more effective targeted interventions in risk mitigation approaches. Lastly, it can serve to reinterpret digital innovation management and propose future avenues of research on risk.
Keywords
Citation
Du Plooy, H., Tommasi, F., Furlan, A., Nenna, F., Gamberini, L., Ceschi, A. and Sartori, R. (2024), "A human-centered perspective on individual risks for digital innovation management: an integrative conceptual review", European Journal of Innovation Management, Vol. ahead-of-print No. ahead-of-print. https://doi.org/10.1108/EJIM-09-2023-0821
Publisher
:Emerald Publishing Limited
Copyright © 2024, Hilda Du Plooy, Francesco Tommasi, Andrea Furlan, Federica Nenna, Luciano Gamberini, Andrea Ceschi and Riccardo Sartori
License
Published by Emerald Publishing Limited. This article is published under the Creative Commons Attribution (CC BY 4.0) licence. Anyone may reproduce, distribute, translate and create derivative works of this article (for both commercial and non-commercial purposes), subject to full attribution to the original publication and authors. The full terms of this licence may be seen at http://creativecommons.org/licences/by/4.0/legalcode
1. Introduction
The exponential technological advancements within the rapid burgeoning of Industry 4.0 (I4.0) underlay the revolution of production and operation processes of the manufacturing sector. Technology-backed manufacturing is on the rise with over 90% of companies worldwide that are using or transitioning to digital manufacturing technology (Fictiv and Dimensional Research, 2022). Internet of Things (IoT), robotics, artificial intelligence (AI), Big data, augmented reality (AR) and Cloud Computing are examples of largely adopted technologies in manufacturing processes (Costantino et al., 2021; Marinescu et al., 2023). The transformation of the manufacturing sector influences and boosts demands in other sectors, prompting businesses and organizations to rapidly invest in digital innovation (Spieske and Birkel, 2021), that is, to invest in the adoption, generation, or recombination of new digital technologies in organizations (see Felicetti et al., 2023; Shukla and Singh, 2023; Troisi et al., 2023). While this vogue for economic growth is welcome, I4.0 is the object of attention of policymakers and scholars for understanding also the individual being potentials (Bianco et al., 2023; European Commission (EC), 2021; Spieske and Birkel, 2021).
It is in this context that policymakers have called for a novel revolution, namely Industry 5.0 (I5.0), by marking I4.0 with a more value-oriented approach (EC, 2021). On the surface, Industry 5.0 implies a shift of the paradigm of technological innovations from economic growth to a human-oriented approach that places the well-being of the employee at the center of the production process (Leng et al., 2022). For example, I4.0 technologies may increase the complexity of skills needed among workers such as problem-solving and decision-making resulting in cognitive overload and fatigue (Wixted et al., 2018). This can be the case of exoskeletons where the lack of skills of the workers may result in physical discomfort or lack of human flexibility (Fox et al., 2020; Thorvald et al., 2021). Likewise, while virtual and AR-based technologies can improve work procedures and enhance workers’ cognitive abilities, they can also impair psychological well-being with a sense of isolation (Vanneste et al., 2020).
The values of industry 5.0 stress the importance of acknowledging the presence of possible individual level risks associated with digital innovation. Accordingly, recent reviews of the literature on I4.0 and manufacturing sector have placed particular concerns around potential risks to the individual (Costantino et al., 2021; Meissner et al., 2020). If not properly designed, the massive implementation of I4.0 technologies can result in physical risks for the employee such as physical discomfort, pain, injury, illness or diseases brought on by their interaction with specific technologies (Lowe et al., 2022; Papetti et al., 2021; Wixted et al., 2018). Likewise, technologies can increase psychological risks of cognitive (knowledge-and skills), emotional and mental health (Lordan and Stringer, 2022; Panchetti et al., 2023). Lastly, human-technological interactions can result in routinary jobs or lead employees to develop habits that could be harmful for themselves in medium-to-long terms (Brougham and Haar, 2018; Hopko and Mehta, 2022; Meissner et al., 2020).
Against this background, there is a dearth of knowledge on how and what risks I4.0 technologies can bring to the individual (Costantino et al., 2021). Prior works have not considered a precise definition of risk and so we lack understanding on the relationship between digital innovation and individual level risks. It remains unanswered what does it mean and take to minimize risks in developing I5.0 in the manufacturing sector. The vision of I5.0 “recognises the power of industry to achieve societal goals beyond jobs and growth to become a resilient provider of prosperity, by making production respect the boundaries of our planet and placing the wellbeing of the industry worker at the center of the production process” (EC, 2021, p. 14) and this dearth of knowledge is troublesome. The introduction of digital innovations requires the awareness of the potential risks at the individual level. Such a knowledge can inform theory and practice of digital innovation management, i.e., “the practices, processes, and principles that underlie the effective orchestration of digital innovation” (Nambisan et al., 2017, p. 224). Understanding risk becomes a significant element for organizations to realize a resilient and human-centered industry that achieves not just economic goals but also the well-being of the employees. Ultimately, understanding risks at the individual level is an important element to realize digital innovation while following the paradigm of I5.0.
In this paper, we report the results of a systematic review we undertook to provide a clear and complete analysis of the different types of risks to the individual worker associated with the I4.0 technologies implemented in the manufacturing sector. Following the I5.0 vision, we aimed at addressing the unanswered questions about how manufacturing organizations can successfully realize the core value of human-centricity proposed by I5.0. Accordingly, the overarching aim of our study is to address the question, “What is the current knowledge and evidence base concerning risks related to I4.0 technologies, and how can this inform digital innovation management in the manufacturing sector through the lens of the I5.0 paradigm?”. Examining individual risks allows us to understand how to manage digital innovation. Studying individual risks related to I4.0 technologies responds to the call on the understanding of realizing the paradigm of I5.0 and particularly it has implications on how to ensure that digital innovation can be realized while caring for the centrality of the individual. This work can expand the conceptualization of risks by realizing I5.0 paradigm and distinguishing practices, processes and principles for the orchestration of digital innovation which can ultimately contribute to optimal conditions of individuals and organizations.
In the rest of the paper, we proceed as follows. First, we delineate the method used to search for and extract data from relevant articles and the decisions made about inclusion criteria. Second, we provide an overview of the extracted contributions following our research questions. In this section, we synthetize the contributions on individual level risk related to digital innovation of I4.0. Lastly, we discuss our results highlighting our contribution to the literature. Inspired by the paradigm of I5.0, we set out a unique framework to understand risks related to I4.0 technologies for research and managerial implications.
2. Methodology
In the systematic review of the literature, we refer to the methodological lines proposed by Briner and Denyer (2012). Systematic literature review aims to “report as accurately as possible what is known and not known about the research questions addressed in the review” ( Briner et al., 2009, p. 27). Briner and Denyer approach is regarded as an effective approach in the area of organization and management studies where different disciplines and perspectives are interwoven. This approach allows to collect multiple data giving researchers the flexibility to understand themes with coherence. Briner and Denyer propose to adhere to four main principles rather than strict criteria in order to guarantee a rigorous approach, namely, a) organization, b) transparency, c) replicability and d) quality. Firstly, the review has to be conducted according to a system or method specifically designed to address the research questions of the review. For purposes of transparency the method followed has to be clearly stated so that this will ensure other researchers to effectively replicate the review. Finally, through synthesis, the results in relation to the research question/s can be summarized in a structured and organized manner for replicability, credibility and relevance (Briner et al., 2009; Winchester and Salji, 2016; Xiao and Watson, 2019).
Briner and Denyer (2012) principles translate into concrete research stages which constitute the steps that must be undertaken to conduct the systematic literature review. Researchers are involved in the (1) planning of the review (i.e. research question/s and pilot literature search) which follows (2) the realization of a structured search. Then, researchers (3) evaluate and shift through material. Lastly, by (4) analyzing and synthesizing information, they (5) present the findings.
2.1 Data collection
In the planning stage, we developed and refined our research questions and piloted the search of databases using the key terms (see Figure 1). We conducted a title, abstract and keyword search on Scopus and Web of Science which resulted in N = 100,785 on Scopus and N = 6,793,373 on Web of Science.
We continued with the structured research using a detailed structure that combines a set of keywords relating to industry 4.0 and the different set of technologies associated with I4.0 (Costantino et al., 2021) and one set of keywords referring to risk (see Appendix 1 for a full list of search terms). To determine the date range, we considered that Industry 4.0 only commenced in 2011. We confined our search to studies published in English between 2011–May 2023 since the first mention of Industry 4.0 appeared in 2011 at the Hanover industry technology fair in Germany. As the digital transformation and its impact on workers extends across multiple disciplinary fields, we included research from business, economics, engineering, psychology and health. A majority of articles were in the engineering field with a focus on the implementation of a specific technology with limited research on the specific impact on workers. Therefore, the size of compatible literature reduced exponentially. Lastly, we only included peer-reviewed articles, other reviews and book chapters. We decided to exclude working papers, dissertations and conference proceedings (i.e., gray literature) giving concerns around academic rigor and to uphold transparency for reproducibility (Briner and Denyer, 2012; Podsakoff et al., 2005). This structured research resulted in a reliable amount of contributions with n = 259 on Scopus and n = 780 on Web of Science.
2.2 Data extraction and initial analysis
In the third phase of evaluating/shifting material, we evaluated the search results by initially applying the inclusion and exclusion criteria based on abstracts. Abstracts of the extracted contributions were viewed in light of the scope of our research questions considering I4.0 technology in the manufacturing sector with specific mention of individual risks to workers. Two reviewers independently read through the abstracts to fully understand concepts presented and screened for studies related to only I4.0 technologies and risks to workers in the manufacturing sectors. Any conflicting opinions by the two reviewers were discussed with a third reviewer.
After the selection of relevant abstracts (n = 47), the fourth phase, i.e., analysis/thematic coding, involved reviewers in reading the extracted contributions. To do so, a structured form to evaluate each included contribution was used. This was uploaded on an Excel sheet for direct input of the quantitative data and headings based on the research questions, namely a) the definitions and theories of risk, b) type of technologies utilized in the manufacturing sector, c) antecedents of risks and d) the type of risks, e) significant results and f) recommendations regarding practical implications in the form of prevention and intervention programs. Two reviewers conducted their analysis and thematic coding individually and compared their results with two additional researchers. At the end of the analysis and thematic coding stage, a total of n = 12 contributions were not compatible with the research questions. Fifty-one articles composed the final amount of extracted contributions since n = 16 additional contributions were included via cross-referencing (Briner and Denyer, 2012). While the data reduction activity and analysis enabled for a rigorous and careful analysis during phase 4, the use of the form helped for the realization of the synthesis of the results, i.e., phase 5.
3. Results
3.1 Overview of the contributions
The final selection of articles (N = 51) consisted of n = 29 empirical studies, n = 16 reviews of the literature, n = 5 conceptual contributions and n = 1 book chapter (see Tables A1 and A2 in Appendix 2). The majority (n = 33) of the contributions focused on one type of digital technology in the manufacturing sector, whilst others grouped the technologies together under the scope of Industry 4.0. With respect to the research studies, a majority took place in Europe (n = 14), followed by the United States of America (n = 6) with small representation of studies from countries in Asia (China, Japan), Australia, Ireland, Latin America, the Middle East, New Zealand and the United Kingdom. Fifteen of the empirical studies were conducted with workers/operators in the manufacturing sectors, whereas the other majority of samples consisted of academics and students in the engineering or technical fields (n = 7), other professionals/specialists in the manufacturing industry (n = 3) and lastly, working adults in the general population (n = 4).
3.2 Definitions and theories about risk
There was no consensus over definitions and theories about risks in I4.0 across all the contributions that we collected. This aspect resounds in similar works such as Birkel et al. (2019)’s attempt to develop a risk framework with respect to I4.0. Notably, a significant minority of the contributions did not report any reference to theoretical aspects and definitions, and they simply considered risks in general regarding the introduction of I4.0 technologies. For example, Gangwar and Date (2016) defined risk as the potential harm that may arise from some current process or from some future event and proceeded to explain cybersecurity risks of IoT. Conversely, empirical studies have followed different theoretical backgrounds which appeared evident in the manuscripts.
According to the thematic analysis, the majority of the collected contributions refer to a traditional risk assessment for mostly physical risks and safety to workers. For example, empirical contributions examined risks in terms of the type of technology implemented in a specific workplace area (e.g., Chao et al., 2017; Gerdenitsch et al., 2022; Papetti et al., 2020). Several studies looked at risk factors via a multi-level framework (e.g., Bárcia De Mattos et al., 2021; Kumar et al., 2022; Marques et al., 2022; Palumbo et al., 2022; Thylén et al., 2023). Others have combined physiological and neurological responses in human–robot collaboration processes to evaluate specific risks to workers (Hopko et al., 2023; Hopko and Mehta, 2022). Other authors used the term hazards and risks interchangeably or broadly referred to the terms impacts, barriers or challenges in determining the risks to workers and 4.0 technologies (Rymarczyk, 2020; Sheridan, 2016; Thylén et al., 2023).
The analysis of definitions and theories yielded four categories of theoretical models to which scholars refer to evaluate risks in I4.0.
Models within management approach. A number of contributions referred to management models to determine the impact of automation on employment. This is the case of Bárcia De Mattos et al. (2021) who followed such models for evaluations aimed at reshoring from a supply chain management perspective. Others (e.g., Egana-delSol et al., 2022; Fornino and Manera, 2022) focused on changes in tasks and labor flexibility to predict potential risks associated with in order to combat employees' job insecurity. Similarly, more precise evaluations have focused on changes of tasks and job quality by using the job characteristics model (JCM). Gerdenitsch et al. (2022) applied the JCM to assess employee’s experience of autonomy considered as an antecedent of individual well-being.
Another group of scholars have extended the focus on tasks by including organizational aspects, e.g., organizational dynamics, management practices. Brougham and Haar (2018) focused on the employee’s experience and perception of digital technologies as potential predictor of organizational commitment, job satisfaction, turnover intention and affective components (i.e cynicism and depression). This model offers a basis on which to evaluate risks at the level of the employee’s work experience. Similarly, Palumbo et al. (2022) approached the topic of risk via the organizational excellence theory (OET). The authors have questioned the emphasis on the need for improvement of organizational dynamics in the introduction and effects of digital technologies in the workplace. The diagnosis revealed that the OET appears as an effective approach for distinguishing risk factors and different impacts of technologies. Lastly, Birkel et al. (2019) extended task and organizational level models by following the principles of risk assessment theory (RAT) to realize a multi-level risk perspective on workers in the employment of digital technologies such as AI, cobots and smart wearables.
Models within cognitive psychology approach. Another way through which scholars have taken into account risks associated with I4.0 derives from the use of psychological and occupational health classical models. Several authors have concentrated on cognitive theories as framework to evaluate cognitive load in the interaction with technology (Chao et al., 2017). Nakanishi and Sato (2015) referred to information-processing theory (IPT) to focus on the employee’s cognitive capacity. In their study, they evaluated the behavioral, physiological and psychological effects on workers in application of digital manuals with a retinal imaging display in manufacturing. Similarly, Kumar et al. (2022) used the social cognition theory (SCT) to identify and map the potential impact of technologies 4.0 on personal and social behavior of workers. Lastly, Meissner et al. (2020) extended cognitive-based models by interweaving behavioral aspects. They did so by referring to the theory of reasoned action which follows the theory of planned behavior (TPB) and the SCT. Considering behavioral-cognitive factors, Meissner et al. (2020) evaluated risk via the dyad acceptance towards technology and intention to use it among workers.
Models within organizational psychology and ergonomics. Several authors have used theories and models within organizational psychology. They used such an approach in order to incorporate both mental health aspects and their correspondence with work and organizational aspects. For example, Lordan and Stringer (2022) approached risks related to I4.0 technologies by looking at the presence of mental-ill health symptoms. Lowe et al. (2022) used an occupation-specific approach relating to the mental health of workers. Lastly, Wixted et al. (2018) followed the Job Demand Control model to determine the psychosocial demands of digitalization to musculoskeletal complaints and job control through stress states (such as distress and worry) in the manufacturing context.
Under these auspices, scholars have also considered models within ergonomics and human factors literature. This is the case of a number of studies exploring the direct effects and psychosocial risks of digital technology on workers (Marinescu et al., 2023; Papetti et al., 2020; Papetti et al., 2021; Thylén et al., 2023). Some occupation-specific studies looked at the physical effects of digital technologies on body posture of body techniques and occurrence of musculoskeletal disorders (Cunha et al., 2022; Wixted et al., 2018). Within such a broad area of ergonomics, scholars have proposed specific approaches such as cognitive ergonomics and neuroergonomics. With respect to the first, Panchetti et al. (2023) investigated the interaction of human and robot on the cognitive abilities of workers (e.g., cognitive workload), perceived stress and robot user acceptance by employing eye-tracking technology. Others, termed neuroergonomics in an attempt to use neurological measures to quantify aspects such as acceptance and trust towards the adoption of digital technology (Hopko et al., 2023; Hopko and Mehta, 2022).
Models within technological perspectives. A small number of scholars concentrated on technological aspects. This is the case of the Technology acceptance model (TAM) which falls within the information systems theory and illustrates how workers come to accept and use digital technology based on their perceived ease of use and perceived usefulness (Gangwar and Date, 2016; Panchetti et al., 2023). Likewise, information systems theory pertains to the transformation of information and is considered to be a theoretical foundation of information and communication technology (ICT) (Gangwar and Date, 2016). Such an approach offers a framework to identify risk by evaluating the acceptance of technology. Similarly, Thylén et al. (2023) used the Human, Technology and Organization (HTO) model by extending socio-technical systems theory and including three separate and interactive subsystems namely; human, technical and organizational to be considered when determining challenges relating to Automated Guided Vehicles in the manufacturing sector.
3.3 Antecedents of risks
While considering definitions, assessment tools and theories within the literature, the analysis led to identify a small amount of aspects, i.e., antecedents, which may interact to influence the severity of risk to the individual worker. Following the knowledge and evidence within our collected data, these aspects were categorized via three levels, namely individual, organizational and societal.
Individual aspects. Age, gender and level of education as well as dispositional characteristics represent potential antecedents of risks. Brougham and Haar (2018) showed that younger workers with higher awareness of digital technologies have more negative views towards their future career prospects than their older counterparts. Such a negative view can lead to potential perception of harm with technologies and the avoidance of their use. Cunha et al. (2022) and Egana-delSol et al. (2022). reported how female workers feel more at risk due to gender inequalities in access to education and skills in science, technology, engineering and mathematics (STEM) fields. Similarly, lower skilled workers reported to be more at risk to fear job loss and replacement by industrial digitalization (Ghadimi et al., 2022). Lastly, individual personality differences (e.g., motivation) increase resistance to use technology with workers feeling to be at risk of control and lack of autonomy (Gerdenitsch et al., 2022). Several researchers view lack of trust, reluctance to accept and resistance to change as core interrelated determinants extending across the digital technologies, i.e. interaction with Cobots and automated guided vehicles (AGVs) (Ghadimi et al., 2022; Hopko and Metha, 2022; Hopko et al., 2023; Meissner et al., 2020; Marinescu et al., 2023; Panchetti et al., 2023); AI, Cloud computing and Big data (Birkel et al., 2019; Gangwar and Date, 2016; Ghadimi et al., 2022); AR/VR technologies and smart wearables (Gong et al., 2019).
Organizational aspects. Organizational culture, lack of training and communication in the organization represent the main antecedents of risks. For example, lack of communication can increase the perception of risks with workers showing resistance to digital innovation in the workplace. Likewise, lack of training can increase the actual risks for workers who are not able to deal with new technologies (e.g., Cobot) (e.g., Bárcia De Mattos et al., 2021; Birkel et al., 2019; Egana-delSol et al., 2022). Palumbo et al. (2022) showed how preparing workers for digital innovation represents a timely and effective organizational intervention to prevent risk significantly.
Beyond organizational aspects such as culture and communication, the literature informs that the mere act of organizing and managing workers’ tasks can also represent a driver of risks. This implies that specific organizing practices should be involved in digital innovation. For example, Chao et al. (2017) showed that the use of VR in complex maintenance tasks led to longer operation time and errors among the participants. It appears that whether a technological device is associated with simple or complex tasks can greatly influence the cognitive demand required of the worker which in turn can lead to risks for the individual. In this respect, when the digital innovation is not tailored on worker’s previous experience with technology (Lowe et al., 2022), their perception of usefulness and ease of usability (Gangwar and Date, 2016; Panchetti et al., 2023) as well as the design and quality of user-machine interfaces (Nakanishi and Sato, 2015; Panchetti et al., 2023) can either simplify the worker’s interaction with the technology or lead to an increase of risks.
Societal aspects. A small number of contributions reported how social acceptability (Kumar et al., 2022) and people’s view of technology as utopian or dystopian can determine the probability to accept technologies (Marinescu et al., 2023). Lastly, the extent to which governments support and develop policies to safely regulate the implementation of technologies is regarded as an important driver for the future success of industrial digitalization (Meissner et al., 2020).
3.4 Industry 4.0 technologies and risks
Given the range of definitions, theories and antecedents of risk, it is not surprising that there is a lack of an extensive overview of the specific individual risks related to the adoption of I4.0 technologies in the manufacturing sectors. Accordingly, this also resounds in the absence of a classification of the disparate amount of technologies under the umbrella of I4.0. To approach the fragmented status of the literature, we undertook an analysis of types of risks and types of technologies that yielded a) different categories of technologies based on their main application b) and three domains of individual risks (see Table 1).
3.4.1 Types of technologies
To categorize each type of technology identified, the traditional definition of the term technology as a tool with a specific application was used. This led to the identification of four main categories of I4.0 technologies based on their main application (Slack et al., 2022).
Material processing technologies. These types of technologies assist workers with the transformation and processing of raw materials into useful products or in the transport of such materials, e.g. AGV, Robots, Cobots, computerized numerical control (CNC) and digital manufacturing. The majority of the empirical studies in this review focus on collaborative robots or cobots, that are increasingly common in the manufacturing sectors and share the workspace very closely with the worker (e.g., Cunha et al., 2022; Egana-delSol et al., 2022; Wixted et al., 2018).
Information-processing technologies. In order to collect and process massive amounts of data information from different sources to increase effectiveness of decisions in real time, technologies such as AI, Big data, Cloud computing, Digital twin and Smart wearables are adopted in the manufacturing sectors. For example, big data allows for great streams of data to be produced and communicated on a global scale in order to optimize the sustainable industrial value chain (Leng et al., 2022). Cloud Computing is the process of storing and managing data resources on distant servers and infrastructures, usually by a third party, to permit manufacturers remote access to crucial operational information (Gangwar and Date, 2016).
Worker processing technologies. Digital technologies that are implemented in the service of workers’ activities to boost individual productivity, team performances and decision-making processes, e.g. augmented reality (AR), virtual reality (VR), extended reality (XR), human-systems integration and exoskeletons. For instance, AR integrates virtual and real world settings and is implemented with workers in the manufacturing sectors to increase efficiency in the production chain (Marques et al., 2022). In comparison, VR is an advanced computer-generated virtual environment with which a person can interact. In the manufacturing sectors VR are used in training by allowing workers to test and operate new equipment prior to actual installation (Matsas and Vosniakos, 2017). Exoskeletons, or mechanical wearable structures, assist workers to increase strength and endurance in performing hard manual tasks on the production floor (Fox et al., 2020).
Other integrated technologies. Finally, the IoT encompasses broader integrated and connected technologies (i.e., software) that facilitate communication between the various devices to seek to optimize decision-making and respond in real time to the complexity of manufacturing processes (Papetti et al., 2020).
3.4.2 Type of risks
Our analysis of the contributions showed that the different aspects considered can be grouped into three main domains which are at the a) physical, b) psychological and c) behavioral (see Table 1).
Physical risks. On the surface, research has examined the number of physical risks associated with technological inclusion. As more likely to appear evident in the organizational setting, physical risks stand at the nexus between human and technological devices. Given their interaction with workers, material-related and for human potential technologies such as robot and cobot aims to have the combined benefit of automating routine repetitive tasks whilst maintaining the flexibility of the worker (Lowe et al., 2022). Nevertheless, such an interaction can compromise physical safety with risks to the workers with potential falls, injuries, collisions, increased noise exposure and potential buildup of static electricity and subsequent discharge (Lasota and Shah, 2015; Lowe et al., 2022; Matsas and Vosniakos, 2017; Thylén et al., 2023). Similarly, musculoskeletal complaints and disorders are connected to upper body strain due to increased vigilance needed among workers to maintain safety protocols (Papetti et al., 2021; Wixted et al., 2018). Automated Guided Vehicles (AGV) which are used as modes of transport between production lines (Thylén et al., 2023) can lead to risks for safety as workers have demonstrated to ignore signals of AGV that causes potential for fatal accidents. Additionally, workers showed poor working postures while working with AGV’s increasing risk of musculoskeletal problems (Thylén et al., 2023).
Information- and operational-related technologies such as digital manufacturing, refers to the application of computer systems to the manufacturing products, processes and supply chains (Marinescu et al., 2023). These advanced systems however, can be breached and cause malfunctioning leading to accidents and deaths if not appropriately secured (Kumar et al., 2022). For example, smart wearables are body-worn devices (such as smart watches, smart glasses, rings and bio-sensors) that provide biological data in real time for the purposes of ergonomics in the manufacturing sector. Papetti et al. (2020) reported how when employing bio-sensors, workers suffer physical discomfort.
Lastly, integrated technologies for immersive experiences in working such as AR (a cognitive-assistive technology) that are used in assembly tasks may produce discomfort and physical harm. In a recent laboratory experiment, participants reported physical discomfort when wearing the technology due to the weight of the device and limited field of vision (Gerdenitsch et al., 2022).
Psychological risks. The psychological risks of workers include cognitive-and affective components concerning their perceptions of work and interactions with the digital technologies. Lack of skills are closely linked with increased levels of job insecurity among workers, resulting in fear and anxiety about specifically replaced by robotics (Egana-delSol et al., 2022; Fornino and Manera, 2022; Lordan and Stringer, 2022; Meissner et al., 2020; Palumbo et al., 2022). In studies with cobots, several researchers report cognitive overload and resulting fatigue to be a major cognitive risk factor to workers (Hopko et al., 2023; Panchetti et al., 2023; Wixted et al., 2018). Interestingly, Meissner et al. (2020) with manufacturing workers collaborating with cobots, workers reported mental underload (i.e low level of task demand that leads to boredom and fatigue). In the same study, workers using AI and other smart technology experience separation anxiety when away from devices (Meissner et al., 2020). Studies on the use of extended reality technologies (VR/AR) and smart wearables in the working context, indicate risks of information-processing overload, problem-solving difficulties and cognitive overload leading to operation errors (Chao et al., 2017; Marques et al., 2022; Nakanishi and Sato, 2015). Moreover, Brougham and Haar (2018) showed that workers show higher levels of job insecurity and lower levels of job satisfaction and organizational commitment (i.e., psychological engagement and dedication to their work) when they are aware of technological changes occurring in their workplace. These aspects in turn, have a bi-directional relationship with stress (i.e., technostress) and mental health problems such as depression and anxiety (Birkel et al., 2019; Brougham and Haar, 2018; Lordan and Stringer, 2022; Meissner et al., 2020; Palumbo et al., 2022; Panchetti et al., 2023; Wixted et al., 2018). In a large population study, Lordan and Stringer (2022) find mental health and life satisfaction to be significantly affected by digital technologies in manufacturing. Lastly, Cloud computing and IoT that allows for the sharing of private human data and information increase fear among workers in respect of privacy violations and confidentiality (Birkel et al., 2019; Gangwar and Date, 2016; Kumar et al., 2022).
Behavioral risks. Lastly, technology can impact individual behavior and social dimensions. According to Birkel et al. (2019) digital technologies impact the social dimensions of the workforce the most. More specifically, the increased human–robot collaboration reduces workers' contact with their coworkers and influences their perceived social isolation and available support (Meissner et al., 2020; Wixted et al., 2018). This is also observed in cases of other technologies (such as AI, Big data and Cloud computing) where an overreliance on technology has the risk of increasing social isolation (Birkel et al., 2019; Meissner et al., 2020).
Several studies demonstrated similar behavioral risks affecting workers in respect of their work productivity and performance. For instance, workers report a loss of autonomy reflected by an increased dependence on cobots, perceived workload amplifications, decreased work performance, higher turnover intentions and more likelihood towards absenteeism (Brougham and Haar, 2018; Hopko and Mehta, 2022; Meissner et al., 2020; Wixted et al., 2018). The implementation of AI, Cloud computing and Big data is connected to a sense of loss of autonomy and human control Birkel et al. (2019), addiction and dependence on technology (Meissner et al., 2020) and increased absenteeism (Brougham and Haar, 2018). In the adoption of AR/VR technologies for assembly tasks, Gerdenitsch et al. (2022) indicated a lack of autonomy is linked with passive work roles.
4. Discussion
In the present work, we aimed to identify the current knowledge and evidence base concerning risk related to I4.0 technologies through a systematic literature review, with the purpose of informing innovation management in the manufacturing sector. Following the paradigm of I5.0, we interrogated peer-reviewed scientific publications to reinterpret digital innovation considering individual risks associated with I4.0 technologies. Based on the principles of Briner and Denyer (2012), the analysis and synthesis of a total of 51 articles situated within the manufacturing sector has been conducted. Results show that the academic publications dealing with the specific risks to workers in the physical, psychological and behavioral domains are various and fragmented. Remarkably, they build on different and stand-alone theoretical approaches, lacking a comprehensive view that truly accounts for the interrelatedness between individual risks. In addition, extant literature is limited to identify relevant antecedents and their potential effects on the level and severity of risk perceived among industrial workers in the adoption of 4.0 technologies.
We maintain that the existing literature is not sufficient to inform and reinterpret innovation management following the advent of I5.0. Given the ever-changing nature of industrial technologies, the increasingly smart and fence-less machines and especially the strongly fragmented perspective previously adopted when assessing risk, the literature appears to lack a holistic perspective that can a) include the new risks that might derive from the new types of technologies, while b) considering the individual risk as a multifaceted construct rather than a one-layer factor. Such a holistic perspective is specifically relevant as the shift towards I5.0 implies a focus on person-centered values in contrast to the technological-driven approach that signified previous industrial approaches. For this reason, organizations need to give attention to the potential risks for workers in order to realize a resilient and human-centered industry that allows achievement of economic goals whilst placing the human worker’s well-being central to the success of digital transformation.
4.1 Theoretical implications
As an interdisciplinary endeavor, the topic of individual risks in industry remains a complex phenomenon that obstacles possible concrete theoretical contributions. This is also due to the purpose to renovate industry by marking it with a human-centric approach following the paradigm of I5.0. It is crucial to take a step forward and cross disciplinary boundaries to bring new possibilities and stir up new paradigms for the era of digital innovation management. Ultimately, the present literature review moves from the mere synthesis of the interdisciplinary data collected and proposes a revisitation of the current literature by framing a broad and inclusive illustration of how individual risks can be managed in digital innovation. Specifically, digital innovation in the context of the manufacturing sector implies innovations in product promotion, distribution and pricing but also requires a managerial revisitation of the repertoire of interventions and practices for managing digital innovation if managers want to ensure to prevent risks while investing in the adoption, generation, or recombination of new digital technologies in organizations (see Felicetti et al., 2023; Shukla and Singh, 2023; Troisi et al., 2023).
Then, it is perhaps worth noting that the disparate range of theories, technologies and empirical evidence can be illustrated by considering the interrelatedness of individual level risks, antecedents and types of technologies (see Figure 2). In the absence of a holistic perspective, such an illustration is an attempt to place importance to the centricity of the human in digital innovation which is considered through the lens of the individual risks. This illustration can have a significant influence on the way the manufacturing sector can realize digital innovation with not only an impact on product placement, pricing and marketing but also securing employees and the organizations from potential risks. In line with this, our literature review argues that companies may benefit of a human-centric lens for realizing digital innovation to avoid potential suffering of significant hazards and risks for the individual.
Figure 2 depicts risks as a circuit between physical, psychological and behavioral risks. With the inspiration of the paradigm of industry 5.0, the analysis of the interrelatedness of the types of individual risks indicates that risk is a pragmatic, moral and legal issue that relates to the actual and/or perceived possibility of suffering harm or injury at the physical, psychological and personal levels. First, the breadth of studies on individual risks shows a number of definitions of risks, theories and assessment tools used to evaluate and prevent risks in digital innovation. The number of definitions diverge due to different theoretical backgrounds, yet it appears that such divergence finds a point of contact in the description of risks as physical, psychological or behavioral. Taken together, all the very different contributions suggest potential interrelatedness between different types of risks. Psychological risks such as the fear of losing one’s job can interact with physical discomfort (Gerdenitsch et al., 2022). The relational nature of behavioral risks can affect the way teams cooperate in one organization and increase the potential risks for the individual in general (e.g., Meissner et al., 2020; Wixted et al., 2018). Accordingly, the notion of individual risks can be considered as a circuit of the three different types, i.e., physical, psychological and behavioral.
Second, the collected contributions place risks in two different terms, namely a) as an actual risk with individuals incurring in hazards for their physical, psychological and behavioral health and b) as a perception of risks with individuals experiencing and perceiving the possibility of risks for their physical, psychological and behavioral health. Workers can suffer physically due to the use of specific technologies. Psychologically, they can have different experiences of their interaction with innovative digital systems. Lastly, they can develop habits that can be harmful both for themselves, colleagues and the organization itself. Moreover, risks are treated as pragmatic, moral and legal aspects associated with the technology used. Pragmatic aspects refer to the daily work of employees and their interaction with technology. This is the case of the organization of work resulting from the inclusion of technologies such as cobots which require workers to redefine their tasks and work experience. Moral and legal aspects appear in the implications of technological innovation with employees exposed to ethical issues and legal risks (e.g., Kumar et al., 2022). For example, smart wearables provide data of the individual that must be protected by the organization but also can be perceived by the individual as a violation of their privacy.
Such a view of risks as the intersection of physical, psychological and behavioral represents the core of our human-centric perspective of digital innovation represented in Figure 2. From the figure we show that risk is impacted by the antecedents and the types of technology. However, individual risks cannot be considered as an element totally reducible to them but rather as a single element that represents the ideal of human-centricity for realizing digital innovation in I5.0 in the manufacturing sector.
Figure 2 depicts individual, organizational and societal factors which can directly affect risk, namely antecedents of risks. The antecedents represent include a) the individual characteristics interacting with technological innovation, b) the organizational context in which digital innovation takes place and c) the societal account of technological innovation characterized by implicit and explicit representations of the new technological eras. As noted in our analysis, the antecedents can be directly or indirectly related to the presence of the processes behind risks. For example, age, gender and education of the workers are individual level factors that influence the experience or perception of risks (e.g., Brougham and Haar, 2018; Cunha et al., 2022). Similarly, organizational level factors represent the immediate context in which individuals connect with digital innovation which can have heterogeneous effects (e.g., the lack of an organizational culture devoted to technological innovation can lead workers’ resistance to technological adoption) Lowe et al. (2022), Palumbo et al. (2022).
Finally, Figure 2 shows how digital innovation is represented by a plethora of different and disparate technological tools. In our review, we analyzed digital innovations by grouping them based on the type of applications of technologies. However, these technologies maintain a certain level of interdependence. Different types of technologies can be better understood as overlapping domains and not as fully independent spheres. It becomes relevant to consider how these elements exert joint effects in leading to the experience and perception of risks at the individual level. Moreover, the overlapping of these elements depicted in Figure 2 illustrates how we can better imagine the value of human-centricity in digital innovation. One main implication of this illustration for the manufacturing sector stands in the contribution to understand digital innovation beyond business models. Digital innovation is not only in service of the organizations as it can have a significant divergent impact for the individual in the organization (e.g., individual risk related to I4.0 technologies). Considering a human-centric lens (i.e., following the paradigm of I5.0), digital innovation in the manufacturing sector appears as an alternative to the mere innovation of products development, pricing and distribution that can be also in service of the individual by securing their safety and well-being.
4.2 Managerial implications
The present literature review has a number of managerial implications. Following the impetus of industry 5.0, managerial practices should not remain at the level of the type of technology involved in digital innovation but should be informed by our holistic view of risk as depicted in Figure 2. First, human resource management can tailor risk-specific assessments and develop approaches to prevent and/or mitigate potential risks to the employees. Second, prior to the adoption of digital innovation, organizations should cultivate a culture of transparency providing clear information about the adoption of technologies and their associated risks. As reported in our literature review, communicating the adoption of technological innovations can significantly reduce the occurrence of individual risks (Palumbo et al., 2022). Third, the evidence of psychological harm in digital innovation indicates that organizations should also prioritize mental health support. Taken together these elements inform that organizations may invest in training interventions for upskilling the employees which can directly affect the level of acceptance of digital innovation as well as improving safety conditions of the workers (Trenerry et al., 2021). In parallel, the concept of digital innovation as a mere investment in technological innovations should be reinterpreted by including investment in the workforce. This can be the case of recruitment practices of additional working figures with a specific emphasis on matching the workers’ levels of expertise with the jobs’ requirements.
To conclude, following the ideal of human-centricity in digital innovation, organizations could invest in job design practices supporting the quality of the job of the workers (Zorzenon et al., 2022) as well as in the promotion of psychological safety (Edmondson, 1999). First, our literature review indicates that workers might feel limited autonomy or struggle to interact with new technologies (Birkel et al., 2019; Gerdenitsch et al., 2022). Job design practices could focus on the tasks of the workers and their responsibility. By increasing autonomy, workers could have more freedom, independence and discretion in defining their own way of interacting with technology.
Second, organizations can follow traditional managerial approaches aimed at promoting psychological safety, broadly considered as workers’ feeling safe in expressing and communicating their views and opinions about working conditions. In the context of digital innovation, facilitating psychological safety can nurture an environment in which workers can share their concerns about potential risks. In parallel, psychological safety can also facilitate creativity and innovation among workers on individual and team level. The promotion of psychological safety can benefit organizational competencies, fostering innovation and productivity.
4.3 Limitations and future research
In our literature review, we followed the principles of Briner and Denyer (2012)’s approach with rigor but there are a number of potentially limiting factors. First, in order to stay in line within the scope of our research questions, by searching for specific key terms and combinations we are aware that certain applications might have been overlooked. For instance, we solely included English and Italian publications. This could have led to the probability of excluding relevant scientific literature. Nevertheless, a key contribution of this review was to provide an integrative approach to establish links across the different discipline fields on the impact (i.e., risks) digital transformation have on the worker. Similarly, a second limit is represented by terminology used. Industry 4.0 is generally used to describe the current digital innovation in industry but there might be other terminologies and words which are used in management practices. As for the language of the publications, we limited it to academic publications to identify evidence on the topic.
We took these decisions for practical reasons and we decided to not extend the scope of our search by including contributions from additional contexts. Future research could refer to specific types of contributions (e.g., national reports) to offer a particularistic view of risks in industry 4.0 within specific contexts. Moreover, future related studies can benefit from our interdisciplinary lens on risks and advance theory and solution-focused applications in the spirit of industry 5.0. The lack of in vivo research combining experimental and qualitative designs to explore the interaction of workers with digital technologies remains another priority for future research to deepen the understanding of the potential pitfalls and opportunities that may arise. Ultimately, this research can improve knowledge in the transition towards I5.0, which is meant to place more value on the human worker within the industrial-technological evolution.
5. Conclusion
The extant research on risks connected with digital innovation has yielded various insights where and when individual risks can appear, are generated and to what they can lead for the individual and the organization, yet there remains a plethora of possibilities for scholars and practitioners interested in digital innovation. This is the case of the offer for renovating the mere concept of digital innovation via the paradigm of Industry 5.0. In the present literature review, we have sought to improve our imagination of digital innovation by explicitly following the ideal of human-centricity bringing coherence on the risks for the individual risks and the well-being of the workers. We uncovered the centrality of the human in digital innovation by considering types of technologies, antecedents of risks and types of individual risks expliciting a definition of risks as well as illustrating a possible depiction of the interrelatedness of the very disparate factors. We hope that our review can represent a chance of imagining possibilities about Industry 5.0 to broaden our perspectives on the constant exponential digital evolution.
Figures
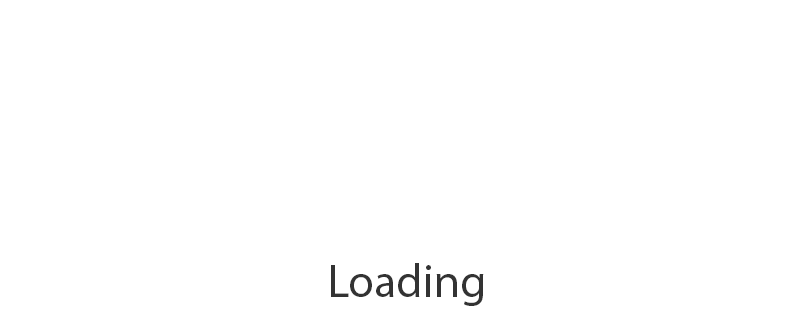
Figure 1
Phases of systematic literature review (Briner and Deyner, 2012)
Types of technologies 4.0 – Type of risks (physical, psychological and behavioral)
Type of technologies or technology 4.0 | Types of risk to workers | ||
---|---|---|---|
Physical | Psychological (cognitive, emotional) | Behavioral (social) | |
Material processing Automated guided vehicles (AGV), robots, cobots, computerized numerical control (CNC) and digital manufacturing |
|
|
|
Information processing AI, Big data, cloud computing, digital twin and smart wearables |
|
|
|
Worker processing AR/VR/XR, exoskeletons and human-systems integration |
|
|
|
Other integrated technologies – Internet of Things (IoT) |
|
|
|
Overview of literature reviews and theoretical/conceptual papers extracted
Authors (Year) | Research approach | Purpose | Main implications |
---|---|---|---|
Adriaensen et al. (2022) | Narrative literature review | Systemic risk analysis to explore risks associated with industrial cobots | More effective safety based risk assessments to increase safe human-cobot collaboration and to follow a socio-technical research perspective to deal with issues referred to modern and future cobot systems |
Alberola and Fassi (2022) | Systematic literature review | Determine a methodology that potentially helps a proper assessment of performance-based interactions between human and machines | Organizations should adopt the holistic model of performance-based assessment methodology to evaluate all the aspects in a collaborative manufacturing environment |
Bautista et al. (2023) | Systematic literature review | Identify strategies to reduce the changes in attention in instructional/educational materials, which use extended reality (XR) to present information to trainee workers | Propose the use of combined strategies to reduce cognitive overload generated by XR display platforms |
Costantino et al. (2021) | Systematic literature review | Identify new and emerging hazards for workers caused by adoption of 4.0 technologies in production environments | Manufacturing organizations must perform robust risk assessments for workers when introducing specific 4.0 technologies |
Di Pasquale et al. (2022) | Scoping review | To define a taxonomy of factors influencing worker’s performance in terms of human reliability in human–robot collaboration | Researchers and practitioners can improve human reliability analysis methods in the context of Industry 4.0 |
Faccio et al., 2022 | Systematic literature review | Investigate how collaborative robots (cobots), human factors, and modern production systems interact with each other | Human factors and cobot capabilities must be linked to all the modern production systems features in Industry 4.0 in order for the work cell to reach its full potential |
Fox et al. (2020) | Multivocal literature review | The use of exoskeletons to increase worker performance across five phases of manufacturing | The introduction of exoskeletons requires careful health and safety planning without introducing new sources of musculoskeletal disorders and accidents |
Gervasi et al. (2020) | Theoretical/conceptual | Construction of a conceptual framework to evaluate human–robot collaboration | Decision makers in manufacturing can use the results obtained from the human–robot collaboration framework to focus on the improvement of certain aspects of the collaboration |
Hoffman and Hancock (2017) | Theoretical/conceptual | Theoretical contributions in how to measure resilience in human factors and ergonomics (HF/E) concerning human–machine technologies | Robust and validated measures of resilience will enable coherent and rational discussions of complex emergent properties in macro cognitive system science |
Kadir et al. (2019) | Systematic literature review | Provide current research and future perspectives on human factors and ergonomics in Industry 4.0 | Research implications to increase empirical investigations on human factors/ergonomics and Industry 4.0 |
Karwowski (2012) | Systematic literature review | Review the human factors challenges of complex adaptive systems | Success of future human–systems integration requires the fusion of paradigms, knowledge, design principles, and methodologies of human factors and ergonomics with those of the science of complex adaptive systems as well as modern systems engineering |
Kleiner et al. (2015) | Theoretical/conceptual | Present a variety of socio-technical systems perspectives on intersections between social– organizational and technology–work process factors as they impact work system analysis, design and operations | The identification of factors that can reliably distinguish between safe and unsafe work systems is an important concern for ergonomists and other safety professionals |
Kolade and Owaseni (2022) | Systematic literature review | Provide current research on how digital transformation will shape the future of work under industry 4.0 | Policy, scholars and stakeholders need to focus attention on evidence-based interventions to tackle worsening inequality exacerbated by disruptive digital transformation, For practitioners a need for informed, conscious and skilled citizen-workers to shape the future of work |
Leng et al. (2022) | Systematic literature review | Provide state of the art about three leading characteristics of Industry 5.0 namely human-centricity, sustainability, and resiliency | Specific recommendations for scholars and policy makers to obtain three values of I5.0 |
Oravec (2021) | Theoretical/conceptual | Discusses how “death by robot” narratives are employed in efforts to characterize workplace and infrastructure automation issues, including the prospects for subsequent anti-robot sabotage or destruction on the part of workers | Organizational transparency needed to assist in acceptance and trust of workers collaborating with cobots/robots |
Reiman et al. (2021) | Systematic literature review | Describe the state-of-the-art of human factors/ergonomics (HF/E) research related to industry 4.0 in manufacturing context | Formulation of an organization level maturity model for organizations to optimize overall socio technical work system performance in the context of rapid technological development |
Ruiz Garcia (2021) | Book chapter: Theoretical/conceptual | Explores role of AI and ML for Human–Robot Cooperation in intelligent and flexible manufacturing | Manufacturing sectors must ensure safe human–robot collaboration |
Rymarczyk (2020) | Theoretical/conceptual | Identify the impact of the Industry 4.0 on the production processes, changes and potential economic, social, and political consequences | The paper contributes to organizations' understanding of 4.0 technologies with regards to stimulating factors, positive effects and benefits, and threats |
Sheridan (2016) | Systematic literature review | Review of the current status of human–robot interaction (HRI) and key current research challenges for the human factors community are described | Research implications in the areas relating to lifestyle, fears, and human values as the most important challenge for understanding human–robot interaction |
Storm et al. (2022) | Scoping review | To identify factors affecting physical and mental health and well-being of workers using collaborative robots (cobots) in manufacturing industries | Introduced the SHELLO model (Software-Hardware-Environment-Liveware-Liveware-Organization) to identify and examine the individual psychological and behavioral elements in complex socio-technical systems. Safety issues related to the physical interaction with the cobot is predominant with implications for occupational health and safety practitioners |
Trenerry et al. (2021) | Systematic literature review | Review on current theory and empirical research into a multi-level theoretical framework (i.e. individual, group, organization) for digital transformation | Organizations should take into consideration and implement specific strategies related to the different multi-level factors that are influenced by digital transformation |
Zorzenon et al. (2022) | Systematic literature review | Identify existing associations between I4.0 technologies and their influences on occupational health and safety (OHS) | Managers should disclose all risks to workers that may arise in implementation of technology and pay attention to adequate training aiming at the continuous development of all workers |
Source(s): Author’s own creation/work
Overview of empirical studies
Author/Year | Research approach | Purpose | Theory/Perspective/Model | Nationality | Sample and size (n = ) |
---|---|---|---|---|---|
Bárcia De Mattos et al. (2021) | Qualitative: Interviews | Effects of automation on employment and reshoring to determine implications for supply chain management and production | Supply chain management perspective | US, Europe & Asia | Workers from 5 factories (n = 8) |
Birkel et al. (2019) | Qualitative: Interviews | Development of a risk framework for Industry 4.0 in the context of sustainability | Risk assessment theory | Germany | Managers in manufacturing sector (n = 14) |
Brougham and Haar (2018) | Mixed methods: Survey and open-ended question | Determined worker’s awareness of Smart Technology, Artificial Intelligence, Robotics, and Algorithms (STARA) and effects on organizational commitment, job satisfaction, turnover intention, cynicism and depression | Organizational commitment theory | New Zealand | General working population (n = 120) |
Chao et al. (2017) | Experimental study (lab controlled) | Explored the effects of three-dimensional virtual reality and traditional training methods on mental workload and training performance in complex maintenance tasks | Cognitive theories | China | Students (n = 48) |
Cunha et al. (2022) | Qualitative: Inductive approach | Effects of automation on body techniques | Physical Ergonomics | Portugal | Workers in Cork manufacturing (n = 12) |
Egana-delSol et al. (2022) | Quantitative comparative analysis | Assessed the risk of automation for female and male workers in four Latin American countries – Bolivia, Chile, Colombia and El Salvador | Task based approach | Latin America | Workers from 4 Latin American countries (n = 6,602) |
Fornino and Manera (2022) | Quantitative modeling | Automation and the future of work: Assessing the role of labor flexibility | Task based approach | US | Various manufacturing industries (not available) |
Gangwar and Date (2016) | Quantitative: Survey | Critical factors of cloud computing adoption in organizations: An empirical study | Technology Acceptance Model (TAM) | India | Workers in various manufacturing companies (n = 280) |
Gerdenitsch et al. (2022) | Mixed methods:Experimental (lab controlled) and semi-structured interviews | Workers' experiences of sense of autonomy with AR-based assistance systems | Job Characteristics model | Austria | General population and students (n = 117) |
Ghadimi et al. (2022) | Quantitative: Survey | An analysis and prioritization of risks in Irish industry with implementation of industry 4.0 in manufacturing | Not identified | Ireland | Professionals in manufacturing industry (n = 12) |
Gong et al. (2019) | Experimental task and questionnaire | Determined effects of virtual reality support to factory layout planning | Not identified | Sweden | Workers (n = 49) |
Hopko and Mehta (2022) | Experimental study (Lab controlled) | Determined trust in shared-space collaborative robots from a neuroscience perspective | Neuroergonomics | US | Engineering students (n = 16) |
Hopko et al. (2023) | Experimental (lab controlled): Physiological/Neurological (HRV & ECG) | Physiological and perceptual consequences of trust in collaborative robots | Neuroergonomics | US | Engineering students (n = 16) |
Keller et al. (2020) | Experimental study (lab controlled) | Determined the effect of digital assistance on process complexity and employee competence in industrial assembly | Not identified | Germany | Students in Technical fields (n = 30) |
Kumar et al. (2022) | Quantitative: Survey | Developed a framework for assessing social acceptability of industry 4.0 technologies for the development of digital manufacturing | Theory of socio-technical transition (TSTT) and social cognition theory (SCT) | India | Experts in manufacturing sector (n = 125) |
Lasota and Shah (2015) | Experimental study (lab controlled) and questionnaires | The effects of human-aware motion planning on team fluency, human satisfaction, and perceived safety and comfort | Not identified | US | Students/Post graduates in Technical fields (n = 20) |
Lordan and Stringer (2022) | Quantitative: Survey | Job automation risk on mental health and life satisfaction | Mental health model | Australia | General working population (n = 41,923) |
Lowe et al. (2022) | Case studies | Looked at influence of industrial robots and automation on type of injuries, productivity and worker acceptance | Occupational Health & Safety | US | Workers (n = 63) |
Marinescu et al. (2023) | Experimental study (lab controlled) and questionnaires | People’s perceptions of digital technologies in manufacturing as positive or negative (utopian vs dystopian) | Human factors and Ergonomics | UK | Adults of general population (varying degrees of knowledge of manufacturing field) (n = 134) |
Marques et al. (2022) | Qualitative: Participatory and focus group | Problem-solving abilities and level of technological literacy in collaboration with Augmented reality | Not identified | Portugal | Experienced specialists in manufacturing field + professor + PhD student (n = 9) |
Matsas and Vosniakos (2017) | Experimental study (lab controlled) and questionnaires | The effects of a virtual reality training system for human–robot collaboration in manufacturing tasks | Not identified | Greece | Mechanical engineering student (n = 30) |
Meissner et al. (2020) | Qualitative: Semi-structured interviews | Looked at assembly workers' level of acceptance of human–robot collaboration | Theory of reasoned action (TRA) and Theory of planned behavior (TPB) | Germany | Workers from 4 manufacturing companies (n = 17) |
Nakanishi and Sato (2015) | Experimental study (lab controlled) and questionnaires | Looked at behavioral, physiological, and psychological effects on workers in application of digital manuals with a retinal imaging display in manufacturing | Information-processing theory | Japan | University students (n = 29) |
Palumbo et al. (2022) | Quantitative: Questionnaires | Psychosocial risks at work with regards to various digital technologies | Organizational Health & Safety, Organizational excellence theory | European countries | Workers/Personnel from service and manufacturing sectors (n = 5,673) |
Panchetti et al. (2023) | Experimental and Questionnaires | The relationship between cognitive workload, workstation design, user acceptance and trust in collaborative robots | Technology acceptance model (TAM), Cognitive ergonomics | Italy | Academic personnel part of Smart Lab (n = 14) |
Papetti et al. (2020) | Experimental, postural and physiological measures | Looked at workers' well-being in human-centered connected factories | Human factors/Ergonomics | Italy | Operators in manufacturing sectors (n = 2) |
Papetti et al. (2021) | Case study | Ergonomic manufacturing equipment through a human-centered methodology | Human factors/Ergonomics The Human, Technology, Organisation (HTO) model | Italy | Operators in manufacturing sectors (not available) |
Thylén et al. (2023) | Case studies | Challenges in introducing automated guided vehicles in a production facility | It builds on socio-technical systems theory and includes three separate subsystems: human, technical, and organizational and it facilitates understanding of the interactions between them | Sweden | Operators, team leaders and engineers (n = 17) |
Wixted et al. (2018) | Quantitative: Questionnaires | Distress and worry as mediators in the relationship between psychosocial risks and upper body musculoskeletal complaints in highly automated manufacturing | Physical Ergonomics, Job demand control theory | Ireland | Workers in manufacturing sectors (n = 235) |
Source(s): Author’s own creation/work
Full list of search terms.
Consistent with the recommendations for systematic literature review to provide one example database search term description (Briner et al., 2009; Winchester and Salji, 2016; Xiao and Watson, 2019), the following terms were input in search string into the advanced search function of Scopus and Web of Science: (“industry 4.0″ OR “I4.0″ OR “fourth industrial revolution” OR “4th industrial revolution” OR “digital technologies” OR “collaborative robots” OR “collaborative robot” or “COBOT” OR “augmented reality” OR “system integration” OR “cloud computing” or “big data” OR “IOT” or “industrial Internet of things” OR “Internet of things” OR “3D printing” OR “cyber security” OR “artificial intelligence” OR “digitalization” OR “digitalisation” or “automation”) AND (“manufacturing” OR “engineering” OR “industries” OR “production” or “operations”) AND (“risk” OR “risk*” OR “hazard” OR “hazard*” OR “danger” OR “threat” OR “safety”).
References
Adriaensen, A., Costantino, F., Di Gravio, G. and Patriarca, R. (2022), “Teaming with industrial cobots: a socio-technical perspective on safety analysis”, Human Factors and Ergonomics in Manufacturing and Service Industries, Vol. 32 No. 2, pp. 173-198, doi: 10.1002/hfm.20939.
Alberola, J.A.M. and Fassi, I. (2022), “Analysis of interactive manufacturing systems: towards a performance-based assessment methodology”, IET Collaborative Intelligent Manufacturing, Vol. 4 No. 4, pp. 286-298, doi: 10.1049/cim2.12063.
Bárcia De Mattos, F., Eisenbraun, J., Kucera, D. and Rossi, A. (2021), “Disruption in the apparel industry? Automation, employment and reshoring”, International Labour Review, Vol. 160 No. 4, pp. 519-536, doi: 10.1111/ilr.12213.
Bautista, L., Maradei, F. and Pedraza, G. (2023), “Strategies to reduce visual attention changes while learning and training in extended reality environments”, International Journal of Interactive Design and Manufacturing, Vol. 17 No. 1, pp. 17-43, doi: 10.1007/s12008-022-01092-9.
Bianco, D., Bueno, A., Godinho Filho, M., Latan, H., Miller, G., Ganga, D., Frank, A.G., Jose, J. and Jabbour, C. (2023), “The role of Industry 4.0 in developing resilience for manufacturing companies during COVID-19”, International Journal of Production Economics, Vol. 256, 108728, doi: 10.1016/j.ijpe.2022.108728.
Birkel, H.S., Veile, J.W., Müller, J.N., Hartmann, E. and Voigt, K.I. (2019), “Development of a risk framework for Industry 4.0 in the context of sustainability for established manufacturers”, Sustainability, Vol. 11 No. 2, pp. 1-27, doi: 10.3390/su11020384.
Briner, R.B. and Denyer, D. (2012), “Systematic review and evidence synthesis as a practice and scholarship tool”, in Rousseau, D.M. (Ed.), The Oxford Handbook of Evidence-Based Management, Oxford, NY, NY, pp. 112-129.
Briner, R.B., Denyer, D. and Rousseau, D.M. (2009), “Evidence-based management: concept clean-up time?”, Academy of Management Perspectives, Vol. 23 No. 4, pp. 19-32, doi: 10.5465/AMP.2009.45590138.
Brougham, D. and Haar, J. (2018), “Smart technology, artificial intelligence, robotics, and algorithms (STARA): employees' perceptions of our future workplace”, Journal of Management and Organization, Vol. 24 No. 2, pp. 239-257, doi: 10.1017/jmo.2016.55.
Chao, C.J., Wu, S.Y., Yau, Y.J., Feng, W.Y. and Tseng, F.Y. (2017), “Effects of three-dimensional virtual reality and traditional training methods on mental workload and training performance”, Human Factors and Ergonomics in Manufacturing and Service Industries, Vol. 27 No. 4, pp. 187-196, doi: 10.1002/hfm.20702.
Costantino, F., Falegnami, A., Fedele, L., Bernabei, M., Stabile, S. and Bentivenga, R. (2021), “New and emerging hazards for health and safety within digitalized manufacturing systems”, Sustainability, Vol. 13 No. 19, 10948, doi: 10.3390/su131910948.
Cunha, L., Silva, D., Macedo, M. and Lacomblez, M. (2022), “‘My whole body is at work’: the silence of gendered body techniques in cork industry in an era of automation”, Ergonomics, Vol. 65 No. 11, pp. 1456-1468, doi: 10.1080/00140139.2022.2066189.
Di Pasquale, V., De Simone, V., Giubileo, V. and Miranda, S. (2022), “A taxonomy of factors influencing worker's performance in human-robot collaboration”, IET Collaborative Intelligent Manufacturing, Vol. e12069 No. 1, doi: 10.1049/cim2.12069.
European Commission, Directorate-General for Research and Innovation Breque, M., De Nul, L. and Petridis, A. (2021), Industry 5.0 : Towards a Sustainable, Human-Centric and Resilient European Industry, Publications Office of the European Union, available at: https://data.europa.eu/doi/10.2777/308407 (accessed 27 August 2023).
Edmondson, A. (1999), “Psychological safety and learning behavior in work teams”, Administrative Science Quarterly, Vol. 44 No. 2, pp. 350-383, doi: 10.2307/2666999.
Egana-delSol, P., Bustelo, M., Ripani, L., Soler, N. and Viollaz, M. (2022), “Automation in Latin America: are women at higher risk of losing their jobs?”, Technological Forecasting and Social Change, Vol. 175, 121333, pp. 1-13, doi: 10.1016/j.techfore.2021.121333.
Faccio, M., Granata, I., Menini, A., Milanese, M., Rossato, C., Bottin, M., Minto, R., Pluchino, P., Gamberini, L., Boschetti, G. and Rosati, G. (2022), “Human factors in cobot era: a review of modern production systems features”, Journal of Intelligent Manufacturing, Vol. 34 No. 1, pp. 85-106, doi: 10.1007/s10845-022-01953-w.
Felicetti, A.M., Corvello, V. and Ammirato, S. (2023), “Digital innovation in entrepreneurial firms: a systematic literature review”, Review of Managerial Science, pp. 1-48, doi: 10.1007/s11846-023-00638-9.
Fictiv and Dimensional Research (2022), “State of manufacturing report”, available at: https://www.fictiv.com/(accessed 27 August 2023).
Fornino, M. and Manera, A. (2022), “Automation and the future of work: assessing the role of labor flexibility”, Review of Economic Dynamics, Vol. 45, pp. 282-321, doi: 10.1016/j.red.2021.07.002.
Fox, S., Aranko, O., Heilala, J. and Vahala, P. (2020), “Exoskeletons: comprehensive, comparative and critical analyses of their potential to improve manufacturing performance”, Journal of Manufacturing Technology Management, Vol. 31 No. 6, pp. 1261-1280, doi: 10.1108/JMTM-01-2019-0023.
Gangwar, H. and Date, H. (2016), “Critical factors of cloud computing adoption in organizations: an empirical study”, Global Business Review, Vol. 17 No. 4, pp. 886-904, doi: 10.1177/0972150916645692.
Gerdenitsch, C., Meneweger, T., Stockreiter, C., Butterer, P., Halbwachs, M. and Scheiblhofer, D. (2022), “Experiencing an augmented-reality assisted assembly task autonomy, passive work attitude, and responsibility”, Journal of Corporate Real Estate, Vol. 24 No. 1, pp. 59-72, doi: 10.1108/JCRE-01-2021-0005.
Gervasi, R., Mastrogiacomo, L. and Franceschini, F.A. (2020), “Conceptual framework to evaluate human-robot collaboration”, International Journal of Advanced Manufacturing Technology, Vol. 108 No. 3, pp. 841-865, doi: 10.1007/s00170-020-05363-1.
Ghadimi, P., Donnelly, O., Sar, K., Wang, C. and Azadnia, A.H. (2022), “The successful implementation of industry 4.0 in manufacturing: an analysis and prioritization of risks in Irish industry”, Technological Forecasting and Social Change, Vol. 175, 121394, doi: 10.1016/j.techfore.2021.121394.
Gong, L., Berglund, J., Fast-Berglund, A., Johansson, B., Wang, Z.P. and Borjesson, T. (2019), “Development of virtual reality support to factory layout planning”, International Journal of Interactive Design and Manufacturing, Vol. 13 No. 3, pp. 935-945, doi: 10.1007/s12008-019-00538-x.
Hoffman, R.R. and Hancock, P.A. (2017), “Measuring resilience”, Human Factors, Vol. 59 No. 4, pp. 564-581, doi: 10.1177/0018720816686248.
Hopko, S.K. and Mehta, R.K. (2022), “Trust in shared-space collaborative robots: shedding light on the human brain”, Journal of the Human Factors and Ergonomics Society, pp. 1-20, doi: 10.1177/00187208221109039.
Hopko, S.K., Mehta, R.K. and Pagilla, P.R. (2023), “Physiological and perceptual consequences of trust in collaborative robots: an empirical investigation of human and robot factors”, Applied Ergonomics, Vol. 106 No. 10, 103863, doi: 10.1016/j.apergo.2022.103863.
Kadir, B.A., Broberg, O. and Souza da Conceição, C. (2019), “Current research and future perspectives on human factors and ergonomics in Industry 4.0”, Computers and Industrial Engineering, Vol. 137, 106004, doi: 10.1016/j.cie.2019.106004.
Karwowski, W. (2012), “A review of human factors challenges of complex adaptive systems: discovering and understanding chaos in human performance”, Journal of the Human Factors and Ergonomics Society, Vol. 54 No. 6, pp. 983-995, doi: 10.1177/0018720812467459.
Keller, T., Behling, M., Stockinger, C., Metternich, J. and Schutzer, K. (2020), “Analysis of the influence of process complexity and employee competence on the effect of digital assistance in industrial assembly”, Production Engineering, Vol. 15 No. 3, pp. 1-8, doi: 10.1007/s11740-020-01005-3.
Kleiner, B.M., Hettinger, L.J., DeJoy, D.M., Huang, Y.H. and Love, P.E.D. (2015), “Sociotechnical attributes of safe and unsafe work systems”, Ergonomics, Vol. 58 No. 4, pp. 635-649, doi: 10.1080/00140139.2015.1009175.
Kolade, O. and Owoseni, A. (2022), “Employment 5.0: the work of the future and the future of work”, Technology in Society, Vol. 71, 102086, doi: 10.1016/j.techsoc.2022.102086.
Kumar, A., Agrawal, R., Wankhede, V.A., Sharma, M. and Mulat-weldemeskel, E. (2022), “A framework for assessing social acceptability of industry 4.0 technologies for the development of digital manufacturing”, Technological Forecasting and Social Change, Vol. 174, 121217, doi: 10.1016/j.techfore.2021.121217.
Lasota, P.A. and Shah, J.A. (2015), “Analyzing the effects of human-aware motion planning on close-proximity human–robot collaboration”, Human Factors, Vol. 57 No. 1, pp. 21-33, doi: 10.1177/0018720814565188.
Leng, J., Sha, W., Wang, B., Zheng, P., Zhuang, C., Liu, Q., Wuest, T., Mourtzis, D. and Wang, L. (2022), “Industry 5.0: prospect and retrospect”, Journal of Manufacturing Systems, Vol. 65, pp. 279-295, doi: 10.1016/j.jmsy.2022.09.017.
Lordan, G. and Stringer, E.J. (2022), “People versus machines: the impact of being in an automatable job on Australian worker's mental health and life satisfaction”, Economics and Human Biology, Vol. 66, 101144, pp. 1-11, doi: 10.1016/j.ehb.2022.101144.
Lowe, B.D., Hayden, M., Albers, J. and Naber, S. (2022), “Case studies of robots and automation as health/safety interventions in small manufacturing enterprises”, Human Factors and Ergonomics in Manufacturing and Service Industries, Vol. 33 No. 1, pp. 69-103, doi: 10.1002/hfm.20971.
Marinescu, A., Argyle, E.M., Duvnjak, J., Wilson, M.L., Lawson, G., Sharples, S., Hubbard, E.M. and Justham, L. (2023), “The future of manufacturing: utopia or dystopia?”, Human Factors and Ergonomics in Manufacturing and Service Industries, Vol. 33 No. 2, pp. 184-200, doi: 10.1002/hfm.20976.
Marques, B., Silva, S., Alves, J., Rocha, A., Dias, P. and Santos, B.S. (2022), “Remote collaboration in maintenance contexts using augmented reality: insights from a participatory process”, International Journal of Interactive Design and Manufacturing, Vol. 16 No. 1, pp. 419-438, doi: 10.1007/s12008-021-00798-6.
Matsas, E. and Vosniakos, G.C. (2017), “Design of a virtual reality training system for human–robot collaboration in manufacturing tasks”, International Journal on Interactive Design and Manufacturing, Vol. 11 No. 2, pp. 139-153, doi: 10.1007/s12008-015-0259-2.
Meissner, A., Trübswetter, A., Conti‐Kufner, A.S. and Schmidtler, J. (2020), “Friend or foe? Understanding assembly workers' acceptance of human‐robot collaboration”, ACM Transactions on Human‐Robot Interaction, Vol. 10 No. 1, pp. 1-30, doi: 10.1145/3399433.
Nakanishi, M. and Sato, T. (2015), “Application of digital manuals with a retinal imaging display in manufacturing: behavioral, physiological, and psychological effects on workers”, Human Factors and Ergonomics in Manufacturing and Service Industries, Vol. 25 No. 2, pp. 228-238, doi: 10.1002/hfm.20535.
Nambisan, S., Lyytinen, K., Majchrzak, A. and Song, M. (2017), “Digital innovation management”, MIS Quarterly, Vol. 41 No. 1, pp. 223-238, doi: 10.25300/misq/2017/41:1.03.
Oravec, J.A. (2021), “Robots as the artificial “other” in the workplace: death by robot and anti-robot backlash”, Change Management: An International Journal, Vol. 21 No. 2, pp. 65-78, doi: 10.18848/2327-798X/CGP/v21i02/65-78.
Palumbo, R., Casprini, E. and Montera, R. (2022), “Making digitalization work: unveiling digitalization's implications on psycho-social risks at work”, Total Quality Management and Business Excellence, pp. 1-21, doi: 10.1080/14783363.2022.2055458.
Panchetti, T., Pietrantoni, L., Puzzo, G., Gualtieri, L. and Fraboni, F. (2023), “Assessing the relationship between cognitive workload, workstation design, user acceptance and trust in collaborative robots”, Applied Sciences, Vol. 13 No. 3, p. 1720, doi: 10.3390/app13031720.
Papetti, A., Gregori, F., Pandolfi, M., Peruzzini, M. and Germani, M. (2020), “A method to improve workers' well-being toward human-centered connected factories”, Journal of Computational Design and Engineering, Vol. 7 No. 5, pp. 1-14, doi: 10.1093/jcde/qwaa047.
Papetti, A., Ciccarelli, M., Brunzini, A. and Germani, M. (2021), “Design of ergonomic manufacturing equipment by a human-centered methodology”, International Journal of Interactive Design and Manufacturing, Vol. 15 No. 1, pp. 107-111, doi: 10.1007/s12008-020-00734-0.
Podsakoff, P.M., MacKenzie, S.B., Bachrach, D.G. and Podsakoff, N.P. (2005), “The influence of management journals in the 1980s and 1990s”, Strategic Management Journal, Vol. 26 No. 5, pp. 473-488, doi: 10.1002/smj.454.
Reiman, A., Kaivooja, J., Parviainen, E., Takala, E.P. and Lauraeus, T. (2021), “Human factors and ergonomics in manufacturing in the industry 4.0 context – a scoping review”, Technology in Society, Vol. 65, pp. 1-9, doi: 10.1016/j.techsoc.2021.101572.
Ruiz Garcia, M.A., Rauch, E., Vidoni, R. and Matt, D.T. (2021), “AI and ML for human-robot cooperation in intelligent and flexible manufacturing”, In Matt, D.T., Modrák, V. and Zsifkovits, H. (Ed. s), Implementing Industry 4.0 in SMEs:concepts, Examples and Applications, Palgrave Macmillan, London. pp. 95-127, doi: 10.1007/978-3-030-70516-9_3.
Rymarczyk, J. (2020), “Technologies, opportunities and challenges of the industrial revolution 4.0: theoretical considerations”, Entrepreneurial Business and Economics Review, Vol. 8 No. 1, pp. 185-198, doi: 10.15678/EBER.2020.080110.
Sheridan, T.B. (2016), “Human–robot interaction: status and challenges”, Human Factors, Vol. 58 No. 4, pp. 525-532, doi: 10.1177/0018720816644364.
Shukla, P. and Singh, S. (2023), “Industry 5.0 and digital innovations: antecedents to sustainable business model”, in Transformation for Sustainable Business and Management Practices: Exploring the Spectrum of Industry 5.0, Emerald Publishing, pp. 17-29.
Slack, N., Brandon-Jones, A. and Burgess, N. (2022), Operations Management, 10th ed., Pearson.
Spieske, A. and Birkel, H. (2021), “Improving supply chain resilience through industry 4.0: a systematic literature review under the impressions of the COVID-19 pandemic”, Computers and Industrial Engineering, Vol. 158, 107452, doi: 10.1016/j.cie.2021.107452.
Storm, F.A., Chiappini, M., Dei, C., Piazza, C., André, E., Reißner, N., Brdar, I., Delle Fave, A., Gebhard, P., Malosio, M., Pena Fernández, A., Štefok, S. and Reni, G. (2022), “Physical and mental well-being of cobot workers: a scoping review using the Software-Hardware-Environment-Liveware-Liveware-Organization model”, Human Factors and Ergonomics in Manufacturing and Service Industries, Vol. 32 No. 5, pp. 419-435, doi: 10.1002/hfm.20952.
Thorvald, P., Fasth Åsa, F. and Romero, D. (2021), “The cognitive operator 4.0”, in Shafik, M. and Case, K. (Ed. s), 18th International Conference on Manufacturing Research: Advances in Manufacturing Technology XXXIV, Derby, UK, Vol. 15, pp. 3-8, doi: 10.3233/ATDE21000.
Thylén, N., Wänström, C. and Hanson, R. (2023), “Challenges in introducing automated guided vehicles in a production facility – interactions between human, technology, and organization”, International Journal of Production Research, Vol. 61 No. 22, pp. 7809-7829, doi: 10.1080/00207543.2023.2175310.
Trenerry, B., Chng, S., Wang, Y., Suhaila, Z.S., Lim, S.S., Lu, H.Y. and Oh, P.H. (2021), “Preparing workplaces for digital Transformation: an integrative review and framework of multi-level factors”, Frontiers in Psychology, Vol. 12, 620766, pp. 1-24, doi: 10.3389/fpsyg.2021.620766.
Troisi, O., Visvizi, A. and Grimaldi, M. (2023), “Rethinking innovation through industry and society 5.0 paradigms: a multileveled approach for management and policy-making”, European Journal of Innovation Management, Vol. 27 No. 9, pp. 22-51, doi: 10.1108/ejim-08-2023-0659.
Vanneste, P., Raes, A., Morton, J., Bombeke, K., Van Acker, B., Larmuseau, C., Depaepe, F. and Van den Noortgate, W. (2020), “Towards measuring cognitive load through multimodal physiological data”, Cognition Technology and Work, Vol. 23 No. 3, pp. 567-585, doi: 10.1007/s10111-020-00641-0.
Winchester, C.L. and Salji, M. (2016), “Writing a literature review”, Journal of Clinical Urology, Vol. 9 No. 5, pp. 308-312, doi: 10.1177/2051415816650133.
Wixted, F., Shevlin, M. and O'Sullivan, L.W. (2018), “Distress and worry as mediators in the relationship between psychosocial risks and upper body musculoskeletal complaints in highly automated manufacturing”, Ergonomics, Vol. 61 No. 8, pp. 1079-1093, doi: 10.1080/00140139.2018.1449253.
Xiao, Y. and Watson, M. (2019), “Guidance on conducting a systematic literature review”, Journal of Planning Education and Research, Vol. 39 No. 1, pp. 93-112, doi: 10.1177/0739456X17723971.
Zorzenon, R., Lizarelli, F.L., de, A. and Moura, D.B.A. (2022), “What is the potential impact of industry 4.0 on health and safety at work?”, Safety Sciences, Vol. 153, e105802, doi: 10.1016/j.ssci.2022.105802.
Further reading
Nahavandi, S. (2019), “Industry 5.0: a human-centric solution”, Sustainability, Vol. 11 No. 16, p. 4371, doi: 10.3390/su11164371.
Acknowledgements
This study was carried out within the PNRR research activities of the consortium iNEST (Interconnected North-Est Innovation Ecosystem) funded by the European Union Next-GenerationEU (Piano Nazionale di Ripresa e Resilienza - PNRR) – Missione 4 Componente 2, Investimento 1.5 – D.D. 1058 23/06/2022, ECS_00000043). This manuscript reflects only the Authors’ views and opinions, neither the European Union nor the European Commission can be considered responsible for them.