Abstract
Purpose
This study assesses the capability of artificial intelligence integrated customer relationship management (AI-CRM) technology for sustaining family businesses in times of crisis, such as the COVID-19 pandemic. The study also investigates the moderating role of strategic intent in sustaining family businesses in times of crisis.
Design/methodology/approach
The authors used dynamic capability view theory and related literature on family business and technology adoption to develop a conceptual model. This model has been validated using the structural equation modeling technique considering 332 usable responses from people of India involved in family businesses and technology adoption. The study also uses multigroup analysis to examine the moderating role of strategic intent.
Findings
The study finds that adoption of AI-CRM technology significantly and positively impacts dynamic capabilities of the family businesses, such as sensing, seizing and transforming capabilities, which in turn positively and significantly influences their sustainability during crises. The study also highlights the significant moderating impact of strategic intent for sustaining family business firms in uncertain times.
Practical implications
This study has highlighted the importance for family businesses to adopt AI-CRM technology and its influence on their dynamic capabilities. The study also provides important inputs to the management of family businesses regarding adoption of new technologies and their significance during crises. The study also documents that strategic intent could help family businesses to survive during such times. The study is conducted in India and thus cannot be generalized.
Originality/value
This study table is unique in that it investigates the influence of AI-CRM technology and the moderating role of strategic intent on family business sustainability in times of crisis. Moreover, the proposed theoretical model is a unique model with explanative power of 71%.
Keywords
Citation
Chaudhuri, R., Chatterjee, S., Kraus, S. and Vrontis, D. (2023), "Assessing the AI-CRM technology capability for sustaining family businesses in times of crisis: the moderating role of strategic intent", Journal of Family Business Management, Vol. 13 No. 1, pp. 46-67. https://doi.org/10.1108/JFBM-12-2021-0153
Publisher
:Emerald Publishing Limited
Copyright © 2022, Ranjan Chaudhuri, Sheshadri Chatterjee, Sascha Kraus and Demetris Vrontis
License
Published by Emerald Publishing Limited. This article is published under the Creative Commons Attribution (CC BY 4.0) licence. Anyone may reproduce, distribute, translate and create derivative works of this article (for both commercial and non-commercial purposes), subject to full attribution to the original publication and authors. The full terms of this licence may be seen at http://creativecommons.org/licences/by/4.0/legalcode
1. Introduction
During the apocalyptic outbreak of the COVID-19 pandemic, demand in the healthcare industry has skyrocketed, but demands in other industries like air transportation, restaurants and tourism have evaporated. Also, general consumption in private households and consumers’ buying power have been severely affected (del Rio-Chanona et al., 2020; Muellbauer, 2020). In such a crisis, businesses have had to contend with several entangled challenges, including implementing essential and strict health protection measures, reducing production and managing with severe supply chain disruptions (Kraus et al., 2020).
This situation has warranted advanced research to provide firms with valid and executable strategies on how to cope with the challenges during and after COVID-19 pandemic. In such a scenario, this study has holistically explored how family firms could sustain themselves with the help of AI-CRM technology capability during the COVID-19 pandemic (Nguyen et al., 2020; Chatterjee et al., 2021a).
In such a crisis, family businesses have faced new challenges, depending on the social backgrounds and cognitive capability of the decision makers/owners of the firms (Mazzelli et al., 2020). In this scenario, family businesses need to adopt suitable business models to address the changed consumption patterns and behaviors (Kraus et al., 2020; Kim, 2020; Zwanka and Buff, 2020). Given their ubiquity in the business environment, family firms play significant roles in the economy, as wealth creators, employers and innovators. This is especially so during a crisis, since increased family ownership considerably reduces the need to strictly follow formal crisis procedures (Faghfouri et al., 2015). The family’s emotional attachment to the firm also affects its overall performance during a crisis (Arrondo-García et al., 2016).
It is a well-established norm that a firm can survive if it can successfully increase the number of customers, as a business’ success principally depends on customer-centric activities (Rosado-Pinto and Loureiro, 2020; Sharif et al., 2021). Even in crisis, family businesses can survive if they successfully develop their customer relationship management (CRM) system (Graca et al., 2015; Ghosh et al., 2021; Tamilmani et al., 2021).
A CRM system involves “identifying a company’s best customers and maximizing the value from them by satisfying and retaining them” (Kennedy, 2006, p. 58). The CRM ability of a firm could be assessed by how well the firm is able to accurately analyze customer data (Li and Nguyen, 2016). But analyzing huge amounts of customer data is difficult for humans, and, in such context, it is argued for artificial intelligence (AI) to be applied in CRM systems, which is commonly known as an AI-CRM system (Molinillo and Japutra, 2017; Chatterjee et al., 2019; Chaudhuri et al., 2021).
Applying AI-CRM technology in family firms, it is believed that they can address the dynamic market changes from the abrupt outbreak of the COVID-19 pandemic (Chatterjee et al., 2021b). After adopting AI-CRM technology in family firms to address the changing business environment due to like COVID-19 pandemic, it is argued that the sensing, seizing and transforming abilities of family businesses (Teece, 2014) would help them to achieve sustainability by using AI-CRM technology.
To explain the situation, dynamic capability view (DCV) theory (Teece et al., 1997) and situational crisis communication theory (Coombs, 2007) have been used in this study. Barring some studies (Herbane, 2013; Kraus et al., 2013, 2020; Faghfouri et al., 2015; Teixeira et al., 2020; Ferreira et al., 2021), no others were found to have exhaustively investigated how family business firms could manage to overcome a crisis using modern technology to achieve sustainability. Family firms must intend to strategize and properly plan to sustain their competitiveness and success to survive a crisis (Weismeier-Sammer, 2011). Family firms should possess strategic intent (SI) to effectively utilize their resources to adopt AI-CRM (Sciascia et al., 2015).
The imposition of non-pharmaceutical interference has had a considerable negative impact on the world economy during the COVID-19 pandemic (Anderson et al., 2020). The world has had to face a tremendous shock to supply and demand, and family firms have suffered greatly. In such a situation, this study contributes to understanding how family firms could enhance their sustainability by improving their CRM activities with the help of AI. For this purpose, this study has developed a theoretical model which provides guidance on how a family firm can survive a crisis by changing its business style so that it can pass a “clean and sustainable company onto subsequent generations” (Bauweraerts, 2013, p. 92). Against this background, this study aims to address the following research questions (RQs):
Does AI-CRM technology capability help to sustain family businesses in times of crisis?
Does strategic intent (SI) have a moderating role of sustaining family business firms during crises?
To answer these research questions, a few theoretical streams have been integrated. It is argued that the sustainability of family businesses is reflected in their exercising their dynamic abilities in turbulent situations. This conceptualization is perceived to be rooted in the concept of situational crisis communication theory and DCV theory. To address the research questions, a conceptual nomological model is proposed, which has been validated by analyzing the inputs of 332 respondents of Indian family firms by applying the technique of partial least squares structural equation modeling.
2. Literature review
Advanced technology helps firms in their day-to-day marketing-based operations (Rust, 2020; Vlačić et al., 2021). However, despite such advantages, family businesses have been facing challenges in the context of adopting, improving and executing modern technologies in times of crisis (Kumar et al., 2020). Firms can use modern technology to make rapid and accurate decisions, but the unprecedented COVID-19 situation appears to have widened the gap between current actual performances and expected performances (Singh and Thirumoorthi, 2019; Cuomo et al., 2020; Singh, 2022).
The COVID-19 pandemic has introduced some new conditions to which firms need to adhere, influencing the reasonings of the decision makers and compelling them to reframe the mechanisms of their marketing operations for their survival by ensuring customers’ welfare (Syam and Sharma, 2018; Singh and Tiwari, 2019; Silva et al., 2021). In this crisis, family businesses, comprising approximately 90% of all firms (Xi et al., 2015), have suffered greatly. They are particularly vulnerable because of their autonomy as well as their family-based standing (Lee, 2006). Several researchers (Runyan, 2006; Kraus et al., 2020; Kubíček et al., 2021; Liew and Devi, 2021) have observed that a crisis typically affects owners of family businesses twice – first because they are private citizens and second because they are business owners (Nordqvist and Melin, 2010).
In defining entrepreneurial family business, Nordqvist and Melin (2010) said it “refers to the family business as a type of organization […] with certain characteristics that can facilitate or constrain entrepreneurial activities, processes, and outcomes” (p. 214). Family businesses usually possess a long-term horizon (Miller and Le Breton–Miller, 2005) and intend to pass a “clean and sustainable company onto subsequent generations” (Bauweraerts, 2013, p. 92). As a result, family members of the business tend to be aligned to sacrifice short-term financial gains for long-term survival of their family legacy (Minichilli et al., 2016; Salehi et al., 2020).
Thus, to sustain the family business during a crisis, the owners need to improve their CRM activities by accurately analyzing various types of customer data (Kupp et al., 2019; Kiwia et al., 2020). As humans cannot quickly and easily analyze the huge volume of customer data, family firm managers need to adopt an AI-integrated CRM system to achieve sustainability during and after the COVID-19 pandemic (Deb et al., 2018; Ghosh et al., 2019; Chatterjee et al., 2019; Galati et al., 2021; Singh, 2022).
However, merely adopting new technology cannot guarantee that a firm will enjoy the color of success unless it can choose which available opportunities will sustain it, assimilate those opportunities and then transform those opportunities for the betterment of the firm during a crisis (Teece, 2014). Thus, in a crisis, when the business scenario undergoes changes, firms, especially family firms, must possess dynamic abilities, comprising sensing, seizing and reconfiguring abilities, to survive by adopting and using new technology like AI-CRM to address the apocalyptic situation.
This concept corroborates the essence of situational crisis communication theory and DCV theory. To achieve success in a turbulent situation, family businesses must possess SI which drives them to take up specific change initiatives within a portfolio structure (Lois et al., 2020). These initiatives are perceived to act as positive catalysts that facilitate the relationship between customers of the family businesses in crisis with the dynamic capability predictors (Żukowska et al., 2021).
3. Theoretical underpinning and development of conceptual model
3.1 Theoretical underpinning
When a firm faces an abrupt crisis, like COVID-19, it needs to understand the situation and estimate whatever threats it might have to face. Firms need to assess how much damage the crisis could inflict if they do not take any protective and remedial actions in response to the threat. In such a scenario, the treatments that firms need to adopt can be better identified by the concept of situational crisis communication theory (Coombs, 2007). Although this theory principally deals with how a firm can survive a threat to its reputation, the measures prescribed in this theory are perceived to help any firm to survive a crisis.
Situational crisis communication theory posits that the actions the firms need to take to address the crisis depend on the gravity of the crisis. It posits a roadmap for how the key facets of the crisis could impact some of its attributes. This theory classified three types of crises: the victim cluster, the intellectual cluster and the accidental cluster (Coombs, 2016). The crisis associated with the sudden outbreak of the COVID-19 pandemic comes under the category of victim cluster when firms become the victims of the situation. This theory prescribes three treatments to combat the crisis: deny, diminish and rebuild.
It is argued that in the context of the emerging crisis from the COVID-19 pandemic, firms need to take up a “rebuild strategy”, where it is perceived that there is necessity to adopt the latest technology to revamp the CRM activities. It is also argued that adoption of CRM integrated with AI could manage the situation. However, it will not suffice for firms to only adopt AI-CRM. The steps enumerated above are common to all types of firms, including family businesses, which is the subject of this study.
After adopting AI-CRM technology to harness its full benefits in the dynamic market situation of the COVID-19 pandemic, firms must possess the dynamic capability to combat the crisis, which is in consonance with DCV theory (Teece et al., 1997). Eckstein et al. (2015) also called dynamic capability a higher order capability of the firms, which could help them address any highly dynamic and changing business environment. Teece (2012) said that dynamic capability is the “ability to integrate, build, and reconfigure internal and external resources/competencies to address, and possibly shape, rapidly changing business environments” (p. 1395). Teece (2014) also conceptualized dynamic capability as having three dimensions, which are sensing, seizing and reconfiguring capabilities.
Sensing capability is the ability of a firm to identify or develop, or codevelop, and measure technical opportunities to meet the needs of customers and to improve business value. Seizing capability is conceptualized as the ability of a firm to mobilize the necessary resources to fulfill customers’ need in the dynamic market, which helps to improve the overall value of the firm’s business. The reconfiguring, or transforming, capability of a firm is interpreted as the activities which could “recombine bundles of resources and ordinary capabilities” (Fainshmidt et al., 2016, p. 2) to “innovate and respond to (or bring about) changes in the market and in the business environment more generally” (Teece, 2014, p. 332).
In terms of DCV theory, all three capabilities (sensing, seizing and transforming abilities) are perceived to allow firms to utilize the adopted AI-CRM technology for ensuring their sustainability in times of crisis. Family businesses are perceived to be especially impacted by their SI that enables the firm to successfully implement different transformational projects.
3.2 Development of hypotheses and conceptual model
The inputs of the literature review and the theories helped the researchers to identify that family businesses can address crises, like the COVID-19 pandemic, by adopting an AI-integrated CRM system. By using AI-CRM technology, owners could sustain their family businesses during uncertain times by appropriately developing their dynamic capabilities of sensing, seizing and transforming, with the support of SI. Here, all these determinants will be discussed, and hypotheses will be formulated to develop a theoretical model.
3.2.1 Adoption of AI-CRM technology in family businesses (ACF)
Due to the abrupt global crisis caused by the outbreak of the COVID-19 pandemic, family firms have experienced new challenges, and they have had to rely on their decision makers’ cognitive thinking (Mazzelli et al., 2020). The crisis that emerged has drastically changed the patterns of consumer buying behavior (Kim, 2020; Zwanka and Buff, 2020). The new apocalyptic environment necessitated family businesses to change and adjust their business models by adopting, developing and implementing new technology (Kumar et al., 2020; Rust, 2020; Vlačić et al., 2021). In such a situation, success is perceived to have been actuated had the family businesses efficiently, timely and accurately analyzed the huge volume of customer data to meet customers’ needs (Singh and Tiwari, 2019; Silva et al., 2021). As such, it is argued that CRM activities must be considerably developed, which can be achieved by integrating AI-technology with CRM systems of family businesses (Schultz and Pick, 2012; Graca et al., 2015).
The mere adoption of technology in a firm cannot bring it success unless the firm can duly integrate the technology with the available opportunities in the dynamic market. For this, the firms’ dynamic capabilities are to be developed with the help of modern technology, like AI integrated with their CRM system. As opined by Teece (2014), the dynamic capability of a firm is comprised of sensing, seizing and transforming capabilities. Accordingly, it is hypothesized as follows:
Adoption of AI-CRM technology in a family business (ACF) positively impacts sensing capability (SEN) of the firm.
Adoption of AI-CRM technology in a family business (ACF) positively impacts seizing capability (SEI) of the firm.
Adoption of AI-CRM technology in a family business (ACF) positively impacts the transforming capability (TRA) of the firm.
3.2.2 Sensing capability (SEN)
Rapid technology developments along with market changes have caused problems to firms in predicting future business development. New knowledge and updated information are considered a laudable source of innovation. From this perspective, when family firms face entangled challenges during a crisis, they need to explore appropriate opportunities in technologies and markets, due to the changing business environment (Zhou et al., 2017).
Therefore, sensing capability (SEN) of the firm is perceived to be fundamental to deal with the situation. SEN is concerned with realizing what changes are required in the existing operations or what are the potential opportunities for developing new services (Zitkiene et al., 2015). SEN effectively influences the innovation of products and services (Kodama, 2018). It is considered to encompass the generation and dissemination of, and responsiveness to, market intelligence (Pavlou and El Sawy, 2011; Teece, 2017). Wamba et al. (2019) explained SEN as “the ability of a given organization to identify, develop, codevelop, and assess technological opportunities that can meet customers’ needs and business opportunities” (p. 3).
In the context of adopting AI-CRM in family businesses during a crisis, we perceive that they can successfully operate and survive in a crisis provided they possess the capability to sense potential and appropriate opportunities. Accordingly, the following hypothesis is developed.
Sensing capability (SEN) of the family businesses positively impacts the sustainability of family businesses in times of crisis (SFB).
3.2.3 Seizing capability (SEI)
DCV theory considers that seizing capability (SEI) is considered a dynamic capability of a firm (Teece et al., 1997). It is considered the ability of a firm to effectively seize opportunities by mobilizing resources (Pezeshkan et al., 2016). According to Teece (2007), the ability to seize opportunities includes addressing marketing opportunities with new products, services or processes and appropriately adopting new technology. With SEI, a firm can select an appropriate business design or model to define its investment and commercialization strategies for deciding when, where and how to invest in the dynamic market environment (Teece, 2007).
In an uncertain environment, it is important to capture new opportunities consequent upon the quick obsolescence of the existing business model (Jansen et al., 2006). This is very relevant for small and medium enterprises and for family businesses, which frequently possess limited resources and depend on appropriate opportunities to survive in a crisis (Guo et al., 2016). In the apocalyptic environment, when business flow suffers interruptions for multifarious reasons, family businesses must undertake market-development related activities to properly seize opportunities and eventually create financial value (Conboy et al., 2020). The focal point of SEI is concerned with resource readiness for adaptation (Barreto, 2010). SEI is interpreted as “mobilization of resources to address needs and opportunities” (Teece et al., 2016, p. 13). During the COVID-19 pandemic, it is perceived that family businesses must develop their seizing abilities to trap appropriate opportunities to effectively use AI-CRM to achieve sustainability. Accordingly, the following hypothesis is formulated:
Seizing capability (SEI) of the family business firms positively impacts the sustenance of family business firms in times of crisis (SFB).
3.2.4 Transforming capability (TRA) and sustaining family businesses in times of crisis (SFB)
A firm is required to transform its resources which are considered necessary for its sustainability, and which appropriately react and respond to environmental changes. A firm’s transforming capability (TRA) is the ability to integrate, reconstruct, renovate, create and, in some cases, dispose of some of the existing resources to ensure better innovation (Teece, 2017).
The ability to coordinate, manage and control activities with various firms is also needed for sourcing appropriate external knowledge, which is associated with the ability to innovate (Becker and Dietz, 2004). As an example, Apple, GE, IBM and P&G have already established systems which are dedicated to collaborating with outside firms, and they have developed a knowledge management system for improving the efficiency of solving problems by using internal and external information to obtain essential inputs in time (Prajogo and Ahmed, 2006). In Wamba et al. (2019), the transforming capability “encompasses all activities that ‘recombine bundles of resources and ordinary capabilities’ to ‘innovate and respond to (or bring about) changes in the market and in the business environment more generally’” (p. 3).
TRA is one of the dynamic capabilities envisaged in DCV theory (Teece et al., 1997). In the context of this study, after adoption of AI-CRM technology by family firms to sustain themselves by addressing the COVID-19 pandemic crisis, the firms are perceived to enjoy success if, along with other dynamic capabilities, they possess transforming ability. Accordingly, it is hypothesized as follows:
Transforming capability (TRA) of a family business positively impacts the sustainability of the firm in times of crisis (SFB).
3.2.5 Moderating role of strategic intent (SI)
SI is conceptualized as a firm’s aspirational plans, and an overarching purpose of approach that is necessary for reaching the firm’s goal or vision (Dvir et al., 2004). SI drives firms to ensure competitive advantage and to even sustain themselves during crises. SI leads a firm to take initiatives to change the strategy to combat any crisis (Żukowska et al., 2021). Firms select proper initiatives that are supported by their SI to address a crisis like the COVID-19 pandemic. These initiatives should be based on the available resources and capabilities which can be safely deployed. In any situation, the SI of a firm supplements the firm for its survival (Weismeier-Sammer, 2011).
To address a crisis like COVID-19, family businesses need to manage the existing resources and planning for the future so that they can effectively utilize the benefits of AI-CRM (Singh and Thirumoorthi, 2019; Chatterjee et al., 2021a). Therefore, family businesses should strategically plan to use AI-CRM technology in the best possible way (Sciascia et al., 2015). They need to utilize the dynamic capabilities by articulating effective strategic planning with proper intent so that they can extract the benefits of dynamic capabilities to achieve better sustainability, even in times of crisis. Accordingly, the following hypotheses are developed.
Strategic intent (SI) moderates the relationships between sensing capability (SEN) of a family business and its sustainability in times of crisis (SFB).
Strategic intent (SI) moderates the relationships between seizing capability (SEI) of a family business firm and sustenance of family business firms in times of crisis (SFB).
Strategic intent (SI) moderates the relationships between transforming capability (TRA) of a family business firm and sustenance of family business firms in times of crisis (SFB).
With all these inputs, a conceptual model has been developed, which is shown in Figure 1.
4. Research methodology
The hypotheses have been tested and the conceptual model has been validated by using the partial least squares structural equation modeling technique. This technique was preferred because it can easily analyze an exploratory study like this (Peng and Lai, 2012). This technique also allows data which are not normally distributed to be analyzed and which are not permissible with the covariance-based structural equation modeling approach (Rigdon et al., 2017; Kock and Hadaya, 2018). Another advantage is that this method does not restrict the sample size (Willbay et al., 2015; Hair et al., 2018). The technique analyzes the feedback from usable respondents against a structured questionnaire through survey. The responses are quantified using a standard scale, which in this study is a five-point Likert scale with anchors on “Strongly Disagree”, marked as 1, and “Strongly Agree”, marked as 5.
4.1 Measurement instruments
From the inputs of the existing literature and theories, instruments to measure the constructs have been developed to confirm content validity. With a series of corrective steps, as recommended by Carpenter (2018), some instruments could be prepared initially that were appropriate for the context of this study. Then, a pretest was conducted with a small sample. The outcomes of the pretest helped to rectify the defects in the readability of some instruments and to simplify the formats of some of them. After the pretest stage, a pilot test was conducted to assess the contemplated response rate. Then, from the inputs of the pilot test, the questions were corrected so that the prospective respondents would not have any problem understanding them. It was also expected that this step would improve the quality of responses. From the results of the pilot test, some of the instruments were dropped, as the researchers felt they did not explain the constructs properly. Finally, the remaining instruments were verified by some experts with knowledge in the domain of this study. From their advice, the instruments were fine tuned. In this way, 28 questions were prepared.
4.2 Collection of data
For targeting usable respondents, the authors of this study selected Indian family businesses, because there are various successful family business firms in India, some of which have been functioning for more than 100 years. Most well-known Indian family firms have expanded their businesses throughout the world. Some of them, whose employees were respondents in the recent study, have become internationally esteemed multinational firms. As such, consideration of the input of these firms’ employees is deemed to yield a result which is expected to have an international dimension. This concept has been taken from the knowledge of different research studies (Eisenhardt and Graebner, 2007; Yin, 2009). As stated before, this study investigates how adoption of AI-CRM in family firms could help sustain the business even during a crisis, such as the COVID-19 pandemic.
The data were collected from respondents who have direct or indirect knowledge about the subject of this study. As such, purposive sampling, otherwise known as judgmental or subjective sampling, has been used (Apostolopoulos and Liargovas, 2016). In this method, the researchers used their own judgment to target the respondents. In this study, most of the authors are based in India, and they felt it was more convenient to select respondents from Indian family firms. In this context, convenience sampling methodology has been used (Garg, 2019). Thus, this study employed a dual sampling methodology (purposive and convenience sampling) to target the potential respondents. In particular, this study targeted employees of eight family firms, whose details are provided in Table 1.
We made several attempts to contact the top executives of these eight family businesses of India to request them to allow their managers of different ranks to participate in this survey. Eventually, a consolidated list of 689 employees holding different managerial ranks was procured. All 689 employees were informed that their anonymity and confidentiality would be strictly preserved and that the aim of this study is purely academic. A response sheet was prepared which contained 28 questions with five options each. A guideline was also provided with each response sheet telling the respondents to put a tick mark in one out of five options against each question. Upon providing the prospective respondents with the response sheets, they were requested to respond within two months (March and April 2021). Within the specific period, 346 responses were obtained, which is a response rate of 50.2%. Of the responses, 346 were scrutinized, and it was found that 14 responses were incomplete. Therefore, we analyzed the inputs of 332 respondents against 28 questions. The details of the demographic statistics are provided in Table 2.
5. Data analysis and results
5.1 Measurement properties and discriminant validity test
Content validity of all the items with reference to their constructs has been assessed by estimating the loading factors (LFs) of all the items. It has been observed that all the item loadings are less than 0.70 (Chin, 2010). To assess the reliability and validity of the constructs, composite reliability (CR) and average variance extracted (AVE) of all the constructs have been estimated. The CR values are found to be greater than 0.80, and the AVE values are found to be greater than 0.50 (Hair et al., 2017). For estimating the internal consistency of the constructs, Cronbach’s alpha (α) of all the constructs have been estimated. The results are shown in Table 3.
It has also been observed that the square roots of the AVEs are greater than the respective bifactor correlation coefficients, which confirms discriminant validity (Fornell and Larcker, 1981). The results are shown in Table 4.
5.2 Moderator analysis (multigroup analysis)
In this study, SI has been considered the moderating variable acting on the relationships covering H2a (SEN→SFB), H2b (SEI→SFB) and H2c (TRA→SFB). In this study, the effects of SI have been examined by considering its two groups, which are Strong SI and Weak SI. For this, multigroup analysis (MGA) has been used with a bootstrapping procedure considering 5,000 resamples. The p-value differences in the effects of the two categories of SI on each relationship have been found to be less than 0.05, thus satisfying the condition laid down by Hair et al. (2016). Hence, the moderating effects of SI on these three linkages are significant. The results are shown in Table 5.
5.3 Common method variance (CMV)
Since the results of this study are based on survey data, there is a chance of getting biased replies. To mitigate it, some preemptive procedural remedies have been adopted. In the survey stage, CMV might come from different sources. The most important source seems to be implicit social responsibility in which the respondents answer questions in a specific way, which causes the indicators to share a certain amount of common variance (Podsakoff et al., 2012). To minimize the effects of CMV, during the pretest stage of the survey, some questions and some formats of the questions were corrected to enhance their readability. Also, the prospective respondents were assured that their confidentiality and anonymity would be protected. These procedural remedies were taken with an expectation that the prospective respondents would reply in an unbiased way. Also, to examine the severity of CMV, statistical methods were used, such as Harman’s Single Factor Test (SFT). The results highlighted that the first factor accounted only for 20.3% of the variance, which is less than the highest allowable value of 50% (Podsakoff et al., 2003). However, some scholars have observed that Harman’s SFT does not yield robust results (Ketokivi and Schroeder, 2004). For this, a further marker variable test has been performed (Lindell and Whitney, 2001), which showed that the differences between the original correlations and CMV-adjusted correlations were all very small (≤0.06) (Mishra et al., 2018) for all the constructs. The results helped to infer that the CMV did not distort the prediction of this study.
5.4 Hypotheses testing (structural equation modeling)
To test the hypotheses, we used cross-validated redundancy measures and considered a separation distance of 7 for each dependent construct. The value of Q2 (Predictive relevance) was found to be 0.073 (positive), indicating that the model possesses predictive relevance (Mishra et al., 2018). To assess the overall model fit, we used standardized root mean square residual as a standard index, and found its values to be 0.062 for PLS (Partial Least Squares) and 0.033 for PLSc (Consistent Partial Least Squares). Both are less than the threshold value of 0.08 (Hu and Bentler, 1999), confirming that the model is in order. This process helps to estimate the path coefficients of all the linkages along with other necessary parameters. The results are provided in Table 6.
With all these inputs, the validated model is provided in Figure 2.
5.5 Results
This study has formulated nine hypotheses out of which three belong to the moderating effects of SI on the linkages H2a, H2b and H2c. All the relationships have been statistically validated. The results demonstrate that ACF could impact SEN, SEI and TRA (H1a, H1b and H1c) significantly and positively, as the concerned path coefficients are 0.21 (p < 0.001, ***), 0.26 (p < 0.01, **) and 0.17 (p < 0.05, *) respectively. The results also demonstrate that SEN, SEI and TRA impact SFB significantly and positively (H2a, H2b and H2c), since the concerned path coefficients are 0.35 (p < 0.001, ***), 0.41 (p < 0.01, **) and 0.38 (p < 0.01, **). The impacts of the moderator SI on H2a, H2b and H2c are all significant and positive, since the relevant path coefficients are 0.15 (p < 0.05, *), 0.18 (p < 0.01, **) and 0.22 (p < 0.01, **). ACF could explain SEN, SEI and TRA to the extent of 31%, 32% and 39%, respectively, whereas SEN, SEI and TRA could simultaneously explain SFB as much as 71%, which is the explanative power of the proposed theoretical model.
6. Discussion
Within a limited time, the apocalypse of COVID-19 has claimed numerous lives. It has also caused awful limitations to people’s daily lives and dramatic changes to business activities. Every firm, regardless of its nature or size, has been affected in one way or other. Against such a backdrop, this study has investigated how adoption of an AI-integrated CRM system could sustain family businesses in such a crisis with moderating influence of the firms’ SI. In this context, this study has demonstrated that family businesses can address a rapidly changing business scenario by successfully integrating, building and reconfiguring their capabilities, which are otherwise known as dynamic capabilities, according to DCV theory (Teece et al., 1997). This has received support from a study by Baía and Ferreira (2019), who documented that the dynamic capability of a firm has a direct impact on its performance. This study has highlighted that with the help of dynamic capability, which includes abilities of sensing, seizing and transforming, it is possible to sustain family businesses by adopting an AI-integrated CRM systems. This has also received support from another study (van Lieshout et al., 2021).
This study has also indicated that SI of a family business acts as a positive catalyst to facilitate the adoption of AI-CRM to address the crisis. Our findings also highlight that SI leads to the development of specific change initiatives within a firm’s portfolio structure. This study also shows the moderating effects of SI on H2a, H2b and H2c. The effects of SI have been categorized into two groups, which are Strong SI and Weak SI. Here, we analyze the moderating effects on the three linkages, H2a, H2b and H2c, through graphical representations in Figures 3–5, respectively.
In these three figures, the continuous and the dotted lines represent the effects of Strong SI and the effects of Weak SI on H2a, H2b and H2c, respectively. In all these figures, it appears that with increases of SEN (for H2a in Figure 3), SEI (for H2b in Figure 4) and TRA (for H2c in Figure 5), the rate of increase of SFB is more from the effects of Strong SI compared to the effects of Weak SI, since, in all the three figures, the gradients of the continuous lines are more than the corresponding gradients of the dotted lines.
6.1 Theoretical contributions
This study makes several theoretical contributions. It has shown that family businesses that adopt an AI-integrated CRM system can sustain their firms even during crises, provided they successfully utilize their dynamic capabilities with their SI. No extant literature is known to have investigated these salient issues simultaneously. This is claimed to be a special theoretical contribution of this study. In the context of such a sudden global crisis, due to COVID-19 apocalypse, this study has shown that family businesses felt the urgent necessity to adopt and adapt a new business model (Kraus et al., 2020) by improving their CRM activities with contributions from AI. The study also has demonstrated that family firms have faced significant impediments toward adoption, development and execution of state-of-the-art technology (AI integrated CRM) in such turbulent times.
Again, this study has been able to extend the applicability of situational crisis communication theory (Coombs, 2007) to suggest that family firms can achieve sustainability by adopting an AI-integrated CRM system during a crisis like the COVID-19 pandemic. This theory posits that at the outbreak of the crisis, the managers concerned of the firm need to respond strategically to overcome the threats, which can help to maintain the firm’s reputation. This concept has been extended in this study to show that during a crisis, family businesses should not only focus on protecting their reputation but also on their sustainability, which AI-CRM tool can complement.
This study has also used DCV theory (Teece et al., 1997). This theory explains that for a firm to perform better in a dynamic business environment, it must possess sensing, seizing and transforming capabilities to react and respond to the changes (Teece, 2012). This idea has been extended in this study by arguing that, during the crisis when the business environment undergoes rapid change, family businesses must successfully utilize their dynamic capabilities by articulating appropriate policy with their SI to achieve sustainability with the help of an AI-integrated CRM system. This is also claimed to be another theoretical contribution of this study.
Kraus et al. (2020) investigated how family businesses in five European countries had to cope with the COVID-19 crisis. We extended their research in this study to demonstrate how adoption of modern technology, like AI-CRM, could sustain family businesses during a crisis by using their dynamic capabilities of sensing, seizing, as well as transforming with the moderating effects of SI. This study is mainly concerned with adoption of an AI-integrated CRM system to combat any crisis. In doing so, this study might have used a standard adoption model, but the researchers chose not to. Instead, the researchers chose some better-suited antecedents to develop the theoretical model, which achieved a high explanative power (71%). This is claimed to be a novel theoretical contribution of this study.
6.2 Implication to practice
The findings of this study have provided timely and important implications to owners and managers of the family firms. The theoretical model proposed in this study may be followed by family businesses for effectively responding to a crisis and to come out successful. The aim of developing a model is not only to help businesses to survive the crisis but it also provides a pragmatic prescription for how to emerge from a crisis in a stronger position.
Firms try to know their customers more methodically by “identifying a company’s best customers and maximizing the value from them by satisfying and retaining them” (Kennedy, 2006, p. 58). At this juncture, a CRM tool is deemed to help a firm the most, although the huge volume of customer data needs to be analyzed. This can be done effectively, easily and more accurately at less cost by integrating AI with CRM, especially during the crisis when businesses face multifarious constraints (Chatterjee et al., 2019).
This study highlights that sensing capability helps managers to sustain their family businesses during crises, by identifying and developing appropriate technological and other opportunities. Family business managers need to sense creative product innovation opportunities to satisfy customers’ rapidly changing needs, which will help the firm to achieve sustainability in a turbulent situation.
The study also documents that seizing ability significantly and positively impacts family firms to successfully sustain their businesses during crisis. This implies that to efficiently utilize CRM during a crisis, family business managers need to seize as well as assimilate the sensed opportunities. To accomplish this, they must design the best business model so that they can extract maximum potentials from the AI-CRM applications.
Our research then documents that transforming ability significantly and positively impacts the sustainability of family firms during crisis by adopting AI integrated CRM system. This implies that family business managers must motivate their employees to exchange information with each other to make them uniformly knowledgeable, which could make the best use of AI-CRM applications to achieve sustainability even during a crisis. Though adoption of AI-CRM is expected to help sustain family firms during the COVID-19 pandemic, the unprecedented circumstances exacerbated the gap between the current performance and the expected and desired performance of family firms (Singh and Thirumoorthi, 2019). In such a precarious situation, the managers or owners of the family firms had to reframe their marketing operations, focus on marketing accountability and indulge in activities which could ensure customer welfare as well as the survival of their businesses (Silva et al., 2021).
Finally, this study shows that SI moderates family firms by facilitating their sustainability during a crisis. Therefore, our research suggests that family business owners must articulate effective strategic plans for appropriately using AI-CRM applications during a crisis so that they can sustain their businesses successfully.
6.3 Limitations and future scope of research
This study has provided several theoretical and practical implications, but it is still not free from all limitations. The findings of this study depend on the cross-sectional data, which invites causality defects between the relationships of the constructs. It also causes the problem of endogeneity. It is suggested that future researchers should conduct a longitudinal study to eliminate its occurrence.
The results depend on the input of the respondents based in India. The culture of Indians differs from the cultures of other countries, but the research did not consider cross-cultural aspects. As such, the results of this study lack generalizability. In this context, it is suggested that future researchers may collect input from respondents across the globe. The results derived from such inputs could provide more generalizable results.
With the survey method, we collected inputs from 332 usable respondents. This does not represent a generalizable sample; therefore, it is suggested that future researchers should collect data from more respondents.
This study has used DCV theory (Teece et al., 1997), which Ling Yee (2007) opined suffers from the defect of context insensitivity. This theory is considered unable to accurately identify the conditions under which the ability of family businesses will be most valuable (Dubey et al., 2019). It is suggested to study the optimum condition in which adoption of AI-CRM could sustain family firms during any apocalyptic situation.
The explanative power of the proposed theoretical model is 71%. Future researchers may consider other boundary conditions and other constructs to examine if, by including them, the explanative power of the proposed theoretical model could be enhanced.
Figures
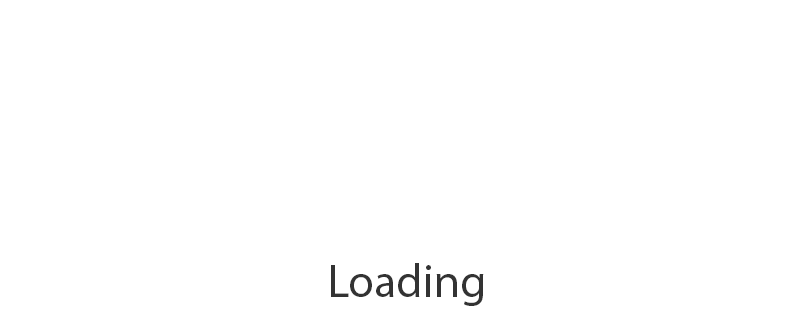
Figure 3
Effects of SI on H2a
Details of family firms
Name of the firm | Founder’s name | Year of establishment | Headquarters |
---|---|---|---|
Aditya Birla group | Seth Shivnarayan Birla | 1857 | Mumbai |
Godrej group | Ardeshir Godrej and Pirojsha Burijorji Godrej | 1897 | Mumbai |
Khoday group | Khoday Eshwara | 1906 | Bengaluru |
Kirloskar group | Laxmanrao Kirloskar | 1911 | Pune |
Murugappa group | A.M. Murugappa Chattair | 1900 | Chennai |
Shapoorji Pallonji | Pallonji Mistry | 1865 | Mumbai |
Tata group | Jamshedji Tata | 1868 | Mumbai |
TVS group | T.V. Sundaramiyengar | 1911 | Madurai |
Demographic statistics (N = 332)
Category | Particulars | Number | Percentage (%) |
---|---|---|---|
Gender | Male | 198 | 59.6 |
Female | 134 | 40.4 | |
Age | Below 40 years | 221 | 66.6 |
Above 40 years | 111 | 33.4 | |
Education | Undergraduate (school level) | 82 | 24.7 |
Graduate | 202 | 60.8 | |
Professional degree holder | 48 | 14.5 | |
Employee hierarchy | Senior manager | 42 | 12.6 |
Mid-level manager | 119 | 35.8 | |
Junior-level manager | 171 | 51.6 |
Measurement properties
Constructs/Items | LF | CR | AVE | t-values | α |
---|---|---|---|---|---|
ACF | 0.90 | 0.87 | 0.94 | ||
ACF1 | 0.95 | 26.12 | |||
ACF2 | 0.97 | 37.17 | |||
ACF3 | 0.89 | 38.13 | |||
ACF4 | 0.94 | 30.11 | |||
ACF5 | 0.95 | 35.14 | |||
ACF6 | 0.90 | 27.29 | |||
SEN | 0.84 | 0.81 | 0.89 | ||
SEN1 | 0.89 | 38.17 | |||
SEN2 | 0.82 | 29.11 | |||
SEN3 | 0.92 | 22.25 | |||
SEN4 | 0.95 | 27.19 | |||
SEN5 | 0.86 | 24.79 | |||
SEN6 | 0.96 | 39.11 | |||
SEI | 0.89 | 0.85 | 0.94 | ||
SEI1 | 0.94 | 29.92 | |||
SEI2 | 0.96 | 22.91 | |||
SEI3 | 0.87 | 26.02 | |||
SEI4 | 0.90 | 27.19 | |||
SEI5 | 0.89 | 31.17 | |||
SEI6 | 0.95 | 28.39 | |||
TRA | 0.91 | 0.84 | 0.90 | ||
TRA1 | 0.92 | 24.26 | |||
TRA2 | 0.89 | 27.29 | |||
TRA3 | 0.96 | 28.13 | |||
TRA4 | 0.87 | 27.36 | |||
TRA5 | 0.90 | 25.09 | |||
TRA6 | 0.91 | 26.17 | |||
SFB | 0.92 | 0.87 | 0.95 | ||
SFB1 | 0.95 | 25.12 | |||
SFB2 | 0.90 | 24.16 | |||
SFB3 | 0.92 | 29.09 | |||
SFB4 | 0.95 | 32.16 |
Note(s): ACF, Adoption of AI-CRM technology in a family business; AVE, average variance extracted; CR, composite reliability; LF, loading factor; SEI, Seizing capability; SEN, Sensing capability; SFB, Sustenance of family business firms in times of crisis; TRA, Transforming capability
Discriminant validity test (Fornell and Larcker criteria)
Constructs | ACF | SEN | SEI | TRA | SFB |
---|---|---|---|---|---|
ACF | 0.93 | ||||
SEN | 0.26 | 0.90 | |||
SEI | 0.19 | 0.26 | 0.92 | ||
TRA | 0.32 | 0.37 | 0.24 | 0.91 | |
SFB | 0.33 | 0.29 | 0.31 | 0.30 | 0.93 |
Note(s): ACF, Adoption of AI-CRM technology in a family business; SEI, Seizing capability; SEN, Sensing capability; SFB, Sustenance of family business firms in times of crisis; TRA, Transforming capability
Moderating effects (MGA)
Linkages | Moderator | Hypotheses | p-value differences | Remarks |
---|---|---|---|---|
(SEN→SFB) × SI | SI | H3a | 0.04 | Significant |
(SEI→SFB) × SI | SI | H3b | 0.01 | Significant |
(TRA→SFB) × SI | SI | H3c | 0.03 | Significant |
Note(s): ACF, Adoption of AI-CRM technology in a family business; SEI, Seizing capability; SEN, Sensing capability; SFB, Sustenance of family business firms in times of crisis; TRA, Transforming capability
Structural equation modeling
Linkages | Hypotheses | R2(Coefficient of determination)/Path coefficients | p-values | Remarks |
---|---|---|---|---|
Effects on SEN | R2 = 0.37 | |||
By ACF | H1a | 0.21 | p < 0.001(***) | Supported |
Effects on SEI | R2 = 0.32 | |||
By ACF | H1b | 0.26 | p < 0.01(**) | Supported |
Effects on TRA | R2 = 0.39 | |||
By ACF | 0.17 | p < 0.05(*) | Supported | |
Effects on SFB | R2 = 0.71 | |||
By SEN | H2a | 0.35 | p < 0.001(***) | Supported |
By SEI | H2b | 0.41 | p < 0.01(**) | Supported |
By TRA | H2c | 0.38 | p < 0.01(**) | Supported |
(SEN→SFB) × SI | H3a | 0.15 | p < 0.05(*) | Supported |
(SEI→SFB) × SI | H3b | 0.18 | p < 0.01(**) | Supported |
(TRA→SFB) × SI | H3c | 0.22 | p < 0.01(**) | Supported |
Note(s): ACF, Adoption of AI-CRM technology in a family business; SEI, Seizing capability; SEN, Sensing capability; SFB, Sustenance of family business firms in times of crisis; TRA, Transforming capability. p < 0.05(*); p < 0.01(**); p < 0.001(***)
References
Anderson, R.M., Heesterbeek, H., Klinkenberg, D. and Hollingsworth, T.D. (2020), “How will country-based mitigation measures influence the course of the COVID-19 epidemic?”, The Lancet, Vol. 395 No. 10228, pp. 931-934.
Apostolopoulos, N. and Liargovas, P. (2016), “Regional parameters and solar energy enterprises: purposive sampling and group AHP approach”, International Journal of Energy Sector Management, Vol. 10 No. 1, pp. 19-37.
Arrondo-García, R., Fernández-Méndez, C. and Menéndez-Requejo, S. (2016), “The growth and performance of family businesses during the global financial crisis: the role of the generation in control”, Journal of Family Business Strategy, Vol. 7 No. 4, pp. 227-237.
Baía, E. and Ferreira, J. (2019), “Dynamic capabilities and performance: how has the relationship been assessed?”, Journal of Management and Organization, Vol. 17 No. 2, pp. 1-30.
Barreto, I. (2010), “Dynamic capabilities: a review of past research and an agenda for the future”, Journal of Management, Vol. 36 No. 1, pp. 256-280.
Bauweraerts, J. (2013), “How do private family firms face the crisis? Empirical evidence from Belgium”, International Business Research, Vol. 6 No. 8, pp. 91-111.
Becker, W. and Dietz, J. (2004), “R&D cooperation and innovation activities of firms—evidence for the German manufacturing industry”, Research Policy, Vol. 33 No. 2, pp. 209-223.
Carpenter, S. (2018), “Ten steps in scale development and reporting: a guide for researchers”, Communication Methods and Measures, Vol. 12 No. 1, pp. 25-44.
Chatterjee, S., Ghosh, S.K., Chaudhuri, R. and Nguyen, B. (2019), “Are CRM systems ready for AI integration? A conceptual framework of organizational readiness for effective AI-CRM integration”, The Bottom Line, Vol. 32 No. 2, pp. 144-157.
Chatterjee, S., Chaudhuri, R., Vrontis, D., Thrassou, A. and Ghosh, S.K. (2021a), “ICT-enabled CRM system adoption: a dual Indian qualitative case study and conceptual framework development”, Journal of Asia Business Studies, Vol. 15 No. 2, pp. 257-277.
Chatterjee, S., Rana, N.P., Khorana, S., Mikalef, P. and Sharma, A. (2021b), “Assessing organizational users’ intentions and behavior to AI-integrated CRM systems: a Meta-UTAUT approach”, Information Systems Frontiers, Press, doi: 10.1007/s10796-021-10181-1.
Chaudhuri, R., Vrontis, D., Thrassou, A. and Ghosh, S.K. (2021), “Adoption of artificial intelligence-integrated CRM systems in agile organizations in India”, Technological Forecasting and Social Change, Vol. 168 No. 120783, pp. 1-17.
Chin, W.W. (2010), “How to write up and report PLS analyses”, in Esposito Vinzi, V., Chin, W., Henseler, J. and Wang, H. (Eds), Handbook of Partial Least Squares, Springer, Berlin, Heidelberg, pp. 655-690.
Conboy, K., Mikalef, P., Dennehy, D. and Krogstie, J. (2020), “Using business analytics to enhance dynamic capabilities in operations research: a case analysis and research agenda”, European Journal of Operational Research, Vol. 281 No. 3, pp. 656-672.
Coombs, W.T. (2007), “Protecting organization reputations during a crisis: the development and application of situational crisis communication theory”, Corporate Reputation Review, Vol. 10 No. 3, pp. 163-176.
Coombs, W.T. (2016), “Reflections on a meta-analysis: crystallizing thinking about SCCT”, Journal of Public Relations Research, Vol. 28 No. 2, pp. 120-122.
Cuomo, M.T., Tortora, D., Festa, G., Ceruti, F. and Metallo, G. (2020), “Managing omni-customer brand experience via augmented reality. A qualitative investigation in the Italian fashion retailing system”, Qualitative Market Research Journal, Vol. 23 No. 3, pp. 427-445.
Deb, S.K., Jain, R. and Deb, V. (2018), “Artificial intelligence ― creating automated insights for customer relationship management”, 2018 8th International Conference on Cloud Computing, Data Science and Engineering (Confluence), pp. 758-764.
del Rio-Chanona, R.M., Mealy, P., Pichler, A., Lafond, F. and Farmer, D. (2020), Supply and Demand Shocks in the COVID-19 Pandemic: An Industry and Occupation Perspective, Oxford University Press, Vol. 36, pp. 94-137.
Dubey, R., Gunasekaran, A., Childe, S.J., Blome, C. and Papadopoulos, T. (2019), “Big data and predictive analytics and manufacturing performance: integrating institutional theory and resource based view”, British Journal of Management, Vol. 30 No. 2, pp. 341-361.
Dvir, T., Kass, N. and Shamir, B. (2004), “The emotional bond: vision and organizational commitment among high-tech employees”, Journal of Organizational Change Management, Vol. 17 No. 2, pp. 126-143.
Eckstein, D., Goellner, M., Blome, C. and Henke, M. (2015), “The performance impact of supply chain agility and supply chain adaptability: the moderating effect of product complexity”, International Journal of Production Research, Vol. 53 No. 10, pp. 3028-3046.
Eisenhardt, K.M. and Graebner, M.E. (2007), “Theory building from cases: opportunities and challenges”, Academy of Management Journal, Vol. 50 No. 1, pp. 25-32.
Faghfouri, P., Kraiczy, N.D., Hack, A. and Kellermanns, F.W. (2015), “Ready for a crisis? How supervisory boards affect the formalized crisis procedures of small and medium-sized family firms in Germany”, Review of Managerial Science, Vol. 9 No. 2, pp. 317-338.
Fainshmidt, S., Pezeshkan, A., Lance Frazier, M., Nair, A. and Markowski, E. (2016), “Dynamic capabilities and organizational performance: a meta-analytic evaluation and extension”, Journal of Management Studies, Vol. 53 No. 8, pp. 1348-1380.
Ferreira, J., Fernandes, C., Schiavone, F. and Mahto, R. (2021), “Sustainability in family business – a bibliometric study and a research agenda”, Technological Forecasting and Social Change, Vol. 173, p. 121077, doi: 10.1016/j.techfore.2021.121077.
Fornell, C. and Larcker, D.F. (1981), “Evaluating structural equation models with unobservable variables and measurement error”, Journal of Marketing Research, Vol. 18 No. 1, pp. 39-50.
Galati, A., Chatterjee, S., Chaudhuri, R. and Vrontis, D. (2021), “Adoption of ubiquitous CRM for operational sustainability of the firms: moderating role of technology turbulence”, Sustainability, Vol. 13 No. 18, pp. 10358-10372.
Garg, S. (2019), “Research methodology”, in HR Initiatives in Building Inclusive and Accessible Workplaces, Emerald Publishing, Bingley, pp. 61-83.
Ghosh, S.K., Chatterjee, S. and Chaudhuri, R. (2019), “Adoption of ubiquitous customer relationship management (uCRM) in enterprise: leadership support and technological competence as moderators”, Journal of Relationship Marketing, Vol. 19 No. 2, pp. 75-92.
Ghosh, S.K., Chatterjee, S. and Chaudhuri, R. (2021), “Knowledge management in improving business process: an interpretative framework for successful implementation of AI–CRM–KM system in organizations”, Business Process Management Journal, Vol. 26 No. 6, pp. 1261-1281.
Graca, S.S., Barry, J.M. and Doney, P.M. (2015), “Performance outcomes of behavioral attributes in buyer supplier relationships”, Journal of Business and Industrial Marketing, Vol. 30 No. 7, pp. 805-816.
Guo, H., Su, Z. and Ahlstrom, D. (2016), “Business model innovation: the effects of exploratory orientation, opportunity recognition, and entrepreneurial bricolage in an emerging economy”, Asia Pacific Journal of Management, Vol. 33 No. 2, pp. 533-549.
Hair, J.F., Jr, Hult, G.T.M., Ringle, C.M. and Sarstedt, M. (2016), A Primer on Partial Least Squares Structural Equation Modeling (PLS-SEM), Sage Publications, Thousand Oaks, CA.
Hair, J.F., Hollingsworth, C.L., Randolph, A.B. and Chong, A.Y.L. (2017), “An updated and expanded assessment of PLS-SEM in information systems research”, Industrial Management and Data Systems, Vol. 117 No. 3, pp. 442-458.
Hair, J.F., Jr, Sarstedt, M., Ringle, C.M. and Gudergan, S.P. (2018), Advanced Issues in Partial Least Squares Structural Equation Modeling (PLS-SEM), Sage Publications, Thousand Oaks, CA.
Herbane, B. (2013), “Exploring crisis management in UK small-and medium-sized enterprises”, Journal of Contingencies and Crisis Management, Vol. 21 No. 2, pp. 82-95.
Hu, L. and Bentler, P.M. (1999), “Fit indices in covariance structure modeling: sensitivity to under parameterized model misspecification”, Psychological Methods, Vol. 3 No. 4, pp. 424-453.
Jansen, J.J., Van Den Bosch, F.A. and Volberda, H.W. (2006), “Exploratory innovation, exploitative innovation, and performance: effects of organizational antecedents and environmental moderators”, Management Science, Vol. 52 No. 11, pp. 1661-1674.
Kennedy, A. (2006), “Electronic customer relationship management (eCRM): opportunities and challenges”, Irish Marketing Review, Vol. 18 Nos 1/2, pp. 58-69.
Ketokivi, M.A. and Schroeder, R.G. (2004), “Perceptual measures of performance: fact or fiction?”, Journal of Operations Management, Vol. 22 No. 3, pp. 247-264.
Kim, R.Y. (2020), “The impact of COVID-19 on consumers: preparing for digital sales”, IEEE Engineering Management Review, Vol. 48 No. 3, pp. 212-218.
Kiwia, R.H., Bengesi, K.M.K. and Ndyetabula, D.W. (2020), “Succession planning and performance of family-owned small and medium enterprises in Arusha City – Tanzania”, Journal of Family Business Management, Vol. 10 No. 3, pp. 213-230.
Kock, N. and Hadaya, P. (2018), “Minimum sample size estimation in PLS-SEM: the inverse square root and gamma-exponential methods”, Information Systems Journal, Vol. 28 No. 1, pp. 227-261.
Kodama, M. (2018), Collaborative Dynamic Capabilities for Service Innovation: Creating a New Healthcare Ecosystem, Springer International Publishing AG, Chams.
Kraus, S., Moog, P., Schlepphorst, S. and Raich, M. (2013), “Crisis and turnaround management in SMEs: a qualitative-empirical investigation of 30 companies”, International Journal of Entrepreneurial Venturing, Vol. 5 No. 4, pp. 406-430.
Kraus, S., Clauss, T., Breier, M., Gast, J., Zardini, A. and Tiberius, V. (2020), “The economics of COVID-19: initial empirical evidence on how family firms in five European countries cope with the corona crisis”, International Journal of Entrepreneurial Behavior and Research, Vol. 26 No. 5, pp. 1067-1092.
Kubíček, A., Dofkova, L. and Machek, O. (2021), “Advice-seeking process in family businesses: a qualitative study”, Journal of Family Business Management, Vol. 11 No. 1, pp. 19-31.
Kumar, V., Ramachandran, D. and Kumar, B. (2020), “Influence of new-age technologies on marketing: a research agenda”, Journal of Business Research, Vol. 125 No. 3, pp. 864-877.
Kupp, M., Schmitz, B. and Habel, J. (2019), “When do family firms consider issuing external equity? Understanding the contingent role of families’ need for control”, Journal of Family Business Management, Vol. 9 No. 3, pp. 271-296.
Lee, J. (2006), “Family firm performance: further evidence”, Family Business Review, Vol. 19 No. 2, pp. 103-114.
Li, M. and Nguyen, B. (2016), “When will firms share information and collaborate to achieve innovation? A review of collaboration strategies”, The Bottom Line, Vol. 30 No. 1, pp. 65-86.
Liew, C.Y. and Devi, S.S. (2021), “Family firms, banks and firm value: evidence from Malaysia”, Journal of Family Business Management, Vol. 11 No. 1, pp. 51-85.
Lindell, M.K. and Whitney, D.J. (2001), “Accounting for common method variance in cross-sectional research designs”, Journal of Applied Psychology, Vol. 86 No. 1, pp. 114-121.
Ling-Yee, L. (2007), “Marketing resources and performance of exhibitor firms in trade shows: a contingent resource perspective”, Industrial Marketing Management, Vol. 36 No. 3, pp. 360-370.
Lois, P., Drogalas, G., Karagiorgos, A. and Tsikalakis, K. (2020), “Internal audits in the digital era: opportunities risks and challenges”, EuroMed Journal of Business, Vol. 15 No. 2, pp. 205-217.
Mazzelli, A., De Massis, A., Petruzzelli, A.M., Del Giudice, M. and Khan, Z. (2020), “Behind ambidextrous search: the micro foundations of search in family and non-family firms”, Long Range Planning, Vol. 53 No. 6, p. 101882.
Miller, D. and Le Breton-Miller, I. (2005), Managing for the Long Run: Lessons in Competitive Advantage from Great Family Businesses, Harvard Business Press, Boston, MA.
Minichilli, A., Brogi, M. and Calabro, A. (2016), “Weathering the storm: family ownership, governance, and performance through the financial and economic crisis”, Corporate Governance: An International Review, Vol. 24 No. 6, pp. 552-568.
Mishra, A., Maheswarappa, S.S., Maity, M. and Samu, S. (2018), “Adolescent’s eWOM intentions: an investigation into the roles of peers, the Internet and gender”, Journal of Business Research, Vol. 86, pp. 394-405.
Molinillo, S. and Japutra, A. (2017), “Organizational adoption of digital information and technology: a theoretical review”, The Bottom Line, Vol. 30 No. 1, pp. 33-46.
Muellbauer, J. (2020), “The coronavirus pandemic and US consumption”, VOX CERP Policy Portal, Vol. 1 No. 11, pp. 1-17.
Nguyen, B., Chatterjee, S., Ghosh, S.K., Bhattacharjee, K.K. and Chaudhuri, S. (2020), “Adoption of artificial intelligence integrated CRM system: an empirical study of Indian organizations”, The Bottom Line, Vol. 33 No. 4, pp. 359-375.
Nordqvist, M. and Melin, L. (2010), “Entrepreneurial families and family firms”, Entrepreneurship and Regional Development, Vol. 22 Nos 3/4, pp. 211-239.
Pavlou, P.A. and El Sawy, O.A. (2011), “Understanding the elusive black box of dynamic capabilities”, Decision Sciences, Vol. 42 No. 1, pp. 239-273.
Peng, D.X. and Lai, F. (2012), “Using partial least squares in operations management research: a practical guideline and summary of past research”, Journal of Operations Management, Vol. 30 No. 6, pp. 467-480.
Pezeshkan, A., Fainshmidt, S., Nair, A., Frazier, M.L. and Markowski, E. (2016), “An empirical assessment of the dynamic capabilities performance relationship”, Journal of Business Research, Vol. 69 No. 8, pp. 2950-2956.
Podsakoff, P.M., MacKenzie, S.B., Lee, J.Y. and Podsakoff, N.P. (2003), “Common method biases in behavioral research: a critical review of the literature and recommended remedies”, Journal of Applied Psychology, Vol. 88 No. 5, pp. 879-903.
Podsakoff, P.M., MacKenzie, S.B. and Podsakoff, N.P. (2012), “Sources of method bias in social science research and recommendations on how to control it”, Annual Review of Psychology, Vol. 63, pp. 539-569.
Prajogo, D.I. and Ahmed, P.K. (2006), “Relationships between innovation stimulus, innovation capacity, and innovation performance”, R&D Management, Vol. 36 No. 5, pp. 499-515.
Rigdon, E.E., Sarstedt, M. and Ringle, M. (2017), “On comparing results from CB-SEM and PLS-SEM: five perspectives and five recommendations”, Marketing ZFP, Vol. 39 No. 3, pp. 4-16.
Rosado-Pinto, F. and Loureiro, S.M.C. (2020), “The growing complexity of customer engagement: a systematic review”, EuroMed Journal of Business, Vol. 15 No. 2, pp. 167-203.
Runyan, R.C. (2006), “Small business in the face of crisis: identifying barriers to recovery from a natural disaster 1”, Journal of Contingencies and Crisis Management, Vol. 14 No. 1, pp. 12-26.
Rust, T. (2020), “The future of marketing”, International Journal of Research in Marketing, Vol. 37 No. 1, pp. 15-26.
Salehi, M., Hoshmand, M. and Rezaei Ranjbar, H. (2020), “The effect of earnings management on the reputation of family and non-family firms”, Journal of Family Business Management, Vol. 10 No. 2, pp. 128-143.
Schultz, M. and Pick, D. (2012), “From CM to CRM to CN2: a research agenda for the marketing communications transition”, Advances in Advertising Research, Vol. 3, pp. 421-432.
Sciascia, S., Nordqvist, M., Mazzola, P. and De Massis, A. (2015), “Family ownership and R&D intensity in small- and medium-sized firms”, Journal of Product Innovation Management, Vol. 32 No. 3, pp. 349-360.
Sharif, K., Kassim, N., Faisal, M.N. and Zain, M. (2021), “Impact of skill on bi-dimensional trust within small-to-medium sized enterprises upstream relationships”, EuroMed Journal of Business, Vol. 16 No. 1, pp. 39-68.
Silva, S.C., Corbo, L., Vlačić, B. and Fernandes, M. (2021), “Marketing accountability and marketing automation: evidence from Portugal”, EuroMed Journal of Business, In Press, doi: 10.1108/EMJB-11-2020-0117.
Singh, S. (2022), Adoption and Implementation of AI in Customer Relationship Management, IGI-Global, Hershey, PA.
Singh, A.K. and Thirumoorthi, P. (2019), “The impact of digital disruption technologies on customer preferences: the case of retail commerce”, International Journal of Recent Technology and Engineering, Vol. 8 No. 3, pp. 1255-1261.
Singh, B. and Tiwari, A.A. (2019), “Customer stewardship behavior and stewardship fatigue: a conceptual framework”, Marketing Intelligence and Planning, Vol. 38 No. 3, pp. 386-399.
Syam, N. and Sharma, A. (2018), “Waiting for a sales renaissance in the fourth industrial revolution: machine learning and artificial intelligence in sales research and practice”, Industrial Marketing Management, Vol. 69, pp. 135-146.
Tamilmani, K., Chatterjee, S., Rana, N.P. and Sharma, A. (2021), “The effect of AI-based CRM on organization performance and competitive advantage: an empirical analysis in the B2B context”, Industrial Marketing Management, Vol. 97 No. 8, pp. 205-219.
Teece, D.J. (2007), “Explicating dynamic capabilities: the nature and micro foundations of (sustainable) enterprise performance”, Strategic Management Journal, Vol. 28 No. 13, pp. 1319-1350.
Teece, D.J. (2012), “Dynamic capabilities: routines versus entrepreneurial action”, Journal of Management Studies, Vol. 49 No. 8, pp. 1395-1401.
Teece, D.J. (2014), “The foundations of enterprise performance: dynamic and ordinary capabilities in an (economic) theory of firms”, Academy of Management Perspectives, Vol. 28 No. 4, pp. 328-352.
Teece, D.J. (2017), “Towards a capability theory of (innovating) firms: implications for management and policy”, Cambridge Journal of Economics, Vol. 41, pp. 693-720.
Teece, D.J., Pisano, G. and Shuen, A. (1997), “Dynamic capabilities and strategic management”, Strategic Management Journal, Vol. 18 No. 7, pp. 509-533.
Teece, D., Peteraf, M. and Leih, S. (2016), “Dynamic capabilities and organizational agility: risk, uncertainty, and strategy in the innovation economy”, California Management Review, Vol. 58 No. 4, pp. 13-35.
Teixeira, S., Veiga, P., Figueiredo, R., Fernandes, C., Ferreira, J. and Raposo, M. (2020), “A systematic literature review on family business: insights from an Asian context”, Journal of Family Business Management, Vol. 10 No. 4, pp. 329-348.
van Lieshout, J.W.F.C., van der Velden, J.M., Blomme, R.J. and Peters, P. (2021), “The interrelatedness of organizational ambidexterity, dynamic capabilities and open innovation: a conceptual model towards a competitive advantage”, European Journal of Management Studies, In Press, doi: 10.1108/EJMS-01-2021-0007.
Vlačić, B., Corbo, L., Costa e Silva, S. and Dabić, M. (2021), “The evolving role of artificial intelligence in marketing: a review and research agenda”, Journal of Business Research, Vol. 128 No. 5, pp. 187-203.
Wamba, S.F., Gunasekaran, A., Akter, S. and Dubey, R. (2019), “The performance effects of big data analytics and supply chain ambidexterity: the moderating effect of environmental dynamism”, International Journal of Production Economics, Vol. 222 No. 107498, pp. 107-132.
Weismeier-Sammer, D. (2011), “Entrepreneurial behavior in family firms: a replication study”, Journal of Family Business Strategy, Vol. 2 No. 3, pp. 128-138.
Willaby, H.W., Costa, D.S.J., Burns, B.D., MacCann, C. and Roberts, R.D. (2015), “Testing complex models with small sample sizes: a historical overview and empirical demonstration of what partial least squares (PLS) can offer differential psychology”, Personality and Individual Differences, Vol. 84 No. 1, pp. 73-78.
Xi, J.M., Kraus, S., Kellermanns, F. and Filser, M. (2015), “Mapping the field of family business research: past trends and future directions”, International Entrepreneurship and Management Journal, Vol. 11 No. 1, pp. 113-132.
Yin, R.K. (2009), Case Study Research, 4th ed., Sage, Thousand Oaks.
Zhou, S.S., Zhou, A.J., Feng, J. and Jiang, S. (2017), “Dynamic capabilities and organizational performance: the mediating role of innovation”, Journal of Management and Organization, Vol. 25 No. 5, pp. 731-747.
Zitkiene, R., Kazlauskiene, E. and Deksnys, M. (2015), “Dynamic capabilities for service innovation”, in Gomezelj Omerzel, D. and Laporšek, S. (Eds), MIC 2015: Managing Sustainable Growth, University of Primorska, Koper, pp. 269-278, available at: https://www.researchgate.net/publication/330081446_Dynamic_Capabilities_for_Service_Innovation (accessed 30 November 2021).
Żukowska, B.A., Martyniuk, O.A. and Zajkowski, R. (2021), “Mobilisation of survivability capital – family firm response to the coronavirus crisis”, International Journal of Entrepreneurial Behavior and Research, Vol. 27 No. 9, pp. 48-81.
Zwanka, R.J. and Buff, C. (2020), “COVID-19 generation: a conceptual framework of the consumer behavioral shifts to be caused by the COVID-19 pandemic”, Journal of International Consumer Marketing, Vol. 33 No. 1, pp. 58-67.