Abstract
Purpose
Lean Thinking is an operation management discipline which aims to identify, map and analyse the activities forming a process to detect “value waste” and outline the most effective flow of activities to execute in sequence. Process mapping is often developed in lean projects through the use of the Value Stream Map (VSM). Like many other management tools, the VSM adopts a static and non-systemic perspective in the representation of an organizational process. This may result in the implementation of Lean projects inconsistent with the overall organizational long-term strategy, thus leading to dysfunctional performance. In order to overcome this limit, the paper suggests combining VSM with System Dynamics (SD) modelling.
Design/methodology/approach
The paper is based on a review of the literature on VSM. This review is matched with an analysis of SD modelling principles aimed at explaining the practical and theoretical contribution of this approach to operation and strategic management practices. An illustrative case study is then provided to explore the practical implications of the proposed approach.
Findings
Our results show that SD modelling provides robust methodological support to VSM and Lean Thinking due to its inner characteristics, namely: simulation, systemic view, explicit link between system structure and behaviour and effective visual representation.
Originality/value
This research proposes a novel approach to design VSMs aimed at fostering a strategic perspective in Lean Thinking applications. Such an approach connects two fields of research and practice – i.e. VSM and SD modelling – which have traditionally been kept separated or, at least, partially combined for specific organizational sub-systems, thereby neglecting a broader strategic view of the entire process system.
Keywords
Citation
Noto, G. and Cosenz, F. (2021), "Introducing a strategic perspective in lean thinking applications through system dynamics modelling: the dynamic Value Stream Map", Business Process Management Journal, Vol. 27 No. 1, pp. 306-327. https://doi.org/10.1108/BPMJ-03-2020-0104
Publisher
:Emerald Publishing Limited
Copyright © 2020, Guido Noto and Federico Cosenz
License
Published by Emerald Publishing Limited. This article is published under the Creative Commons Attribution (CC BY 4.0) licence. Anyone may reproduce, distribute, translate and create derivative works of this article (for both commercial and non-commercial purposes), subject to full attribution to the original publication and authors. The full terms of this licence may be seen at http://creativecommons.org/licences/by/4.0/legalcode
1. Introduction and research objectives
Lean Thinking is an Operation Management discipline conceptualized by Womack et al. (1990) through the exploration of the Japanese manufacturing system, being first applied by Toyota Motor Company under the leadership of Taiichi Ohno (see Ohno, 1988; Womack et al., 1990). Lean Thinking aims at enhancing organizational performance and value creation through the reduction (or elimination) of any wasteful activity, whereby waste is defined as “anything does not add value to the customer” (Holden, 2011, p. 265). As argued by Bianchi et al. (2018), the “lean” attribute characterizes an agile, less-structured and more pragmatic approach to apply Thinking methods to operation management, process analysis and performance management.
Early applications of Lean Thinking have been developed in the automotive industry, as well as in other manufacturing sectors. Subsequently, many experiences reported successful results of applying the Lean principles to service sectors, such as healthcare, banks, tourism, public services, etc. (Julien and Tjahjono, 2009; Curatolo et al., 2014; Balocco et al., 2019; Barnabè et al., 2019). Despite the wide diffusion of Lean Thinking worldwide, one of the main issues outlined by the literature with respect to its successful adoption is the difficulty to foster a strategic approach to its effective implementation and usage within organizations (see among others Garnett et al., 1998; Hines et al., 2004; Karim and Arif-Uz-Zaman, 2013; Shamah, 2013; Radnor and Osborne, 2013). As for example, this is the case of complex organizational systems in which multiple processes are interconnected, thereby creating numerous overlaps among their underlying operations and associated organizational units, and Lean applications are exclusively implemented to focus on improving one single process (or operation), thus resulting non-effective. Such pitfalls are not attributable to the Lean Thinking approach itself, rather to its current forms of implementation (Hines et al., 2004; Radnor and Osborne, 2013).
The key momentum of any Lean Thinking application is related to the identification, mapping and analysis of those operations forming the process to detect “value waste” and outline the most effective flow of activities to execute in sequence. Process mapping is often developed in Lean projects through the use of the Value Stream Map (VSM). This is a technique to analyse, (re)design and manage the flow of materials and information required to generate and offer a final product (or service) to customers (Rother and Shook, 1998; Pavnaskar et al., 2003; El-Haik and Al-Aomar, 2006; Serrano Lasa et al., 2008).
The adoption of a VSM implies the “as is” representation of the analysed process, and the consequent identification of those solutions to reduce or eliminate value waste in order to design a new and improved process “to be” implemented. The effects of the emerging solutions are usually assessed once these are effectively implemented. However, implementing these solutions could be quite expensive in terms of time, money and organizational efforts. In addition, although the implemented actions may produce the expected results in terms of efficiency and productivity, not necessarily such results will meet the long-term goals of the organization (Wolstenholme, 2006). This might be the case of the so-called unintended consequences – i.e. when, despite a number of reported short-term improvements, the implemented action leads to an overall dysfunctional performance in the long-run or, similarly, when implemented solutions are only applied to a limited number of organizational units, thus worsening the strategic coordination alongside the overall value creation chain (Meyer and Gupta, 1994; Smith, 1995; Marr, 2006; Melnyk et al., 2013). An example of these unintended consequences can be found in a manufacturing firm whose sales department enhances the process leading to an increase in its market demand (e.g. by introducing remarkable promotional campaigns or discounts). Other conditions being equal, this event can be considered as a successful driver for the business, its competitiveness and viability. However, in case this market demand increase overcomes the actual production capacity of the firm (i.e. new customer orders cannot be fulfilled in due time), this will be likely to cause significant delays in the shipment of products, thus compromising the creation of value for customers, as well as their satisfaction and fidelity in the long term. Another example can be often observed in the healthcare sector whenever the improved productivity of a specific service (e.g. laboratory analysis) fosters its demand even for patients who do not need those treatments, thus worsening the overall outcomes in terms of quality of care produced by the healthcare provider. Both examples describe circumstances that emphasize the emergence of framing complex organizational structures according to a systemic perspective that involves the adoption of effective operation management tools oriented to manage critical trade-offs in time (i.e. short- vs. long-term performance) and space (i.e. aligning outputs among interacting organizational units).
With the intent to overcome the above limits, methodological support can be found in the use of simulation techniques that have proven to boost decision-making processes and operation management by deepening the understanding of how the organizational system functions and behaves by virtue of alternative strategic solutions (Sterman, 2014; Bianchi, 2016; Cosenz and Noto, 2016, 2018a; El-Haik and Al-Aomar, 2006; El-Gafy and Abdelhamid, 2008). When implementing real changes oriented to reduce value waste is too costly, simulation becomes a valuable tool to experiment and discover how complex organizational systems work and where high leverage points may lie (Davis et al., 2007; Sterman, 2014). Although there have been previous attempts to combine Lean Thinking and VSM with simulation techniques in the literature (see among others McDonald et al., 2002; El-Gafy and Abdelhamid, 2008; Solding and Gullander, 2009; Xie and Peng, 2012; Erikshammar et al., 2013; Cavdur et al., 2019; Stadnicka and Litwin, 2019), none of these focused on the potential use of simulation as methodological support to foster a strategic perspective in the implementation of Lean Thinking practices.
With the aim to fill this gap, the paper proposes to combine the Lean Thinking with System Dynamics (SD) simulation modelling.
SD is a methodological approach – developed during the 1950s by Jay Wright Forrester – to depict, model and simulate dynamic social systems characterized by complexity, i.e. in which multiple organizational variables interact aiming to produce a given set of outputs (Forrester, 1958; Sterman, 2000; Größler et al., 2008; Bianchi, 2016). Such an approach has been widely used to support strategic management in organizations at both the operational and strategic level (see among others Größler et al., 2008; Bianchi, 2016; Cosenz and Noto, 2016).
From the combination between SD and VSM originates the Dynamic Value Stream Map (DVSM) as a tool to strengthen the link between operation and strategic management through the adoption of a systemic view of organizational processes and the use of simulation for experimentation. In this perspective, the core purpose of this research is to understand how SD modelling can provide effective methodological support to Lean Thinking, thereby improving organizational process management and associated value creation.
For the above purpose, the research process begins with the review of the literature on Lean Thinking and the VSM with a focus on those extant approaches using simulation-based techniques for developing VSM. This review is combined with an analysis of SD modelling principles aimed at explaining the practical and theoretical contribution of this approach to operational and strategic management practices.
Building on this literature review, the paper proposes and illustrates the DVSM approach as a lean systemic method to support strategic management in operations. Then, following a qualitative perspective, the emerging approach is tested on a case study to offer an illustrative example of DVSM design. A case study strategy is particularly valuable to address theory-building research and, potentially, to demonstrate that existing research does not properly address the investigated propositions (Eisenhardt and Graebner, 2007). In this context, the case study research method is likely to limit potential bias and enrich the analysis (Furnari, 2015; Cosenz et al., 2020), thus offering useful insights on how to frame VSM elements into a systemic structure.
In the last section of the paper, the case study results enable to discuss the main advantages and limitations of the proposed approach in comparison with the methodological gaps found in the literature. Eventually, the paper outlines critical implications for theory and practice and concludes with future research perspectives.
2. Framing Lean Thinking principles and Value Stream Mapping
In 1990, Womack et al. published the book “The machine that changed the World”. In this book, which represents the theoretical grounds of the discipline, they theorized Lean Thinking through the exploration of the Toyota production model. Since then, Lean Thinking has been widely applied to multiple manufacturing sectors, and later on, to services.
Lean Thinking delimits an area of scientific interest which embraces a wide range of stand-alone management practices, such as total quality management (TQM), just-in-time production (JIT), 5S techniques, visual control and so on. These practices are aimed at providing opportunities for supporting or improving performance, as well as involving all organizational levels towards the achievement of common goals according to a more effective, efficient and straightforward perspective (Camacho-Miñano et al., 2013).
The Lean Thinking approach builds on five key pillars aiming to reduce value wastes in the production/supply processes. Waste is defined as “anything does not add value to the customer” (Holden, 2011, p. 265); therefore, reducing wastes is seen as the way to improve efficiency, profitability but, more importantly, to improve customer value (Bashin and Burcher, 2006). The five key pillars of Lean Thinking are (Ohno, 1988; Womack et al., 1990; Womack and Jones, 1996) as follows:
Identify value and waste from the customer perspective;
Identify and map all the activities required in the production/supply process analysed;
Make the product flow continuous – i.e. create a flow of activities to execute in sequence so that it can be offered to the customers without value waste, interruptions or delays;
Establish a pull logic – i.e. it is the customer that pulls the value from the next upstream activity, making the process exclusively demand-driven;
Pursue continuous improvements by introducing small and continuous enhancements to progressively remove non-value-added activities.
As mentioned in the introduction of this paper, the key momentum of every Lean project is related to the process mapping. This is usually carried out through the adoption of VSM (Pavnaskar et al., 2003; El-Haik and Al-Aomar, 2006). The VSM technique was presented as an innovative graphic technique to help practitioners redesign production systems (Serrano Lasa et al., 2008).
VSM is a graphical chart describing a process through the use of specific symbols identifying activities, material and information flows and other elements characterizing a specific process. The aim of the VSM is to synthetically illustrate the current status of a process “as is”, to identify value wastes and to reform the process by envisaging solutions oriented to reduce or eliminate those detected wastes (Rother and Shook, 1998; El-Haik and Al-Aomar, 2006). Figure 1 shows the VSM general framework.
Particularly, the use of VSM provides the display of the activities taking place throughout the production/supply process, the time each of them takes and those activities that add value as well as those that add no value for the customer (Serrano Lasa et al., 2008; Michael et al., 2013; Suarez-Barraza et al., 2016). Thus, it permits the identification of potential causes of waste as well as process improvements, while recognizing that not all identified processes adding no value can be eliminated from the process (Cookson et al., 2011). By means of VSM, information is analysed identifying opportunities for improvement, selecting those to be considered in the design and implementation of the future value chain maps. Subsequently, the emerging results are reviewed, and the outputs of the new map are determined (Michael et al., 2013).
According to the literature (Rother and Shook, 1998; Pavnaskar et al., 2003; Serrano Lasa et al., 2008) the main strengths of VSM are the following: (1) a graphical interface that makes it easier to frame the relationships between material and information flows; (2) the use of a wide perspective for each product/service line reflecting the production system inefficiencies; (3) a common language for the working team oriented to unify concepts and techniques into a single tool; (4) a fertile ground for starting a strategic action plan improvement.
The main limits of the VSM approach are related to the exclusive focus on single product/service processes and the difficulty to experiment with potential solutions (Solding and Gullander, 2009). In fact, these may be tested and evaluated only once effectively put in place. However, some of the undertaken solutions may be costly to implement and do not necessarily lead to the expected results due to the rise of unintended consequences related to the complexity of the system under observation (Sterman, 2000). Therefore, as suggested by the strategic management literature focusing on strategy design tools (e.g. Business Models, Balanced Scorecards), simulation models and associated scenario analysis may better support the understanding of the potential effects and implications that designed solutions involve upon the different drivers of organizational performance, before their real implementation (Bianchi and Montemaggiore, 2008; Barnabé, 2011; Bianchi, 2016; Cosenz and Noto, 2016, 2018b).
Both research and practice report several attempts to combine VSM and simulation techniques (see among others McDonald et al., 2002; El-Gafy and Abdelhamid, 2008; Solding and Gullander, 2009; Shararah et al., 2010, 2011; Xie and Peng, 2012; Abo Hamad et al., 2012; Robinson et al., 2012; Erikshammar et al., 2013; Tobail et al., 2013; Swallmeh et al., 2014; Doğan and Unutulmaz, 2016; Cavdur et al., 2019; Stadnicka and Litwin, 2019). Particularly, the simulation techniques adopted by this stream of research include discrete event simulation (DES), SD and agent-based modelling (ABM). DES has been the most adopted technique for simulating VSMs (Erikshammar et al., 2013), thus providing a valuable tool enabling to replicate the performance trend of an existing organizational system quite realistically. As for its limits, DES requires the collection of a large set of data to result in rigorous and effective analysis. As such, it is likely to neglect the qualitative aspects of the process (e.g. intangibles) which, although difficult to quantify, significantly affect the performance of complex systems (El-Gafy and Abdelhamid, 2008; Wolstenholme, 1999). This limit can be overcome by using ABM or SD modelling (El-Gafy and Abdelhamid, 2008; Xie and Peng, 2012). Both methods apply computer simulation techniques for investigating complex social systems. However, they differ in terms of basic modelling principles since ABM focuses on the “agent”, while SD on the “feedback structures” (Schieritz and Milling, 2003; Wolstenholme, 1999). According to recent research in the strategic management field, the focus on feedback loops and underlying causal relationships provides a powerful strategic perspective to understand the structure and associated performance behaviour of an organizational system (Bianchi, 2016; Cosenz and Noto, 2016, 2018b; Torres et al., 2017; Kunc and O'Brien, 2017). Applied to the operation management field, this perspective may allow decision-makers to overcome the exclusive focus on single processes, thus fostering a broader holistic view aimed to pursue a sustainable development into the entire production system (Poles, 2013; Stadnicka and Litwin, 2019). Nevertheless, previous applications of SD to VSM are mainly focussed on the analysis of manufacturing processes that neglects such a strategic perspective leading to manage trade-offs in time and space, as characterized by Bianchi (2016).
3. An overview of system dynamics modelling
SD is a methodological approach developed by Jay Wright Forrester for modelling and simulating complex physical and social systems and experimenting with the models to design strategies for management and change (Forrester, 1958). It provides a systemic perspective and a set of conceptual tools that enable one to frame the structure and behaviour of complex, non-linear, multi-loop feedback systems (Forrester, 1958; Meadows, 1980; Sterman, 2000). Complexity characterizes those organizational systems in which multiple variables interact – through linear and non-linear causal interdependencies – in order to produce outputs and results over a given period of time (Sterman, 2000).
In particular, SD is a valuable tool for analysing the dynamic tendencies of complex systems – i.e. what kind of behavioural patterns they may generate over time. The main assumption of the SD paradigm is that these patterns arise from the causal structure of the system under observation, seen as a closed boundary, i.e. embodying all main relevant variables related to the phenomenon being investigated. This perspective allows the analyst to build closed chains of causal relationships, known as “feedback loop”. SD models are thus made up of several feedback loops linked to each other that contribute to explaining and describing how and why the organizational system behaves according to certain reported trends. The underlying principle is that, if the process structure determines the system behaviour and the system behaviour determines the organization performance (Davidsen, 1991; Sterman, 2000), then the key to develop sustainable strategies to improve performance is understanding the relationship between processes, structures and behaviours and managing the leverage points (Ghaffarzadegan et al., 2011).
To represent complex and dynamic systems, SD models adopt a graphical syntax in which flow (rate) and stock (level) variables can be distinguished and combined into stock-and-flow diagrams (Größler et al., 2008). By quantifying variables and causal linkages between variables, a system of differential equations is created that can be simulated by numerical algorithms (Sterman, 2000). An SD simulation model is calibrated by comparing model output with empirical data and, in case of discrepancies or inconsistencies, refining the model and input parameters where adequate data do not exist. Once the simulation model has been developed, calibrated and tested whether it realistically behaves, inputs are modified to conduct “what if” analyses of how short- and long-term results would change in response to alternative strategy scenarios (Kunc and O'Brien, 2017; Torres et al., 2017).
The visual representation of systems offered by these models proves to be a valuable tool for understanding complex issues in organizational settings (Größler et al., 2008). Differently from other simulation approaches, SD models make explicit – even at the visual level – the structures that generate a certain system behaviour. This characteristic is particularly relevant when focusing on strategic management processes since it allows one to explore the causal dynamics related to the development and deployment of organization's strategic resources (Morecroft, 2007; Warren, 2008; Bianchi, 2016).
By virtue of its model building processes allowing scholars and professionals to deal with feedback loops, accumulation and depletion processes and delays that commonly characterize value creation and delivery in every organization, SD has been widely and successfully applied to operation management (Größler et al., 2008; Mingers and White, 2010).
In addition to the support that SD provides to operation management research and applications, it is worth to remark that SD has also been extensively used in strategic management studies (see among others Morecroft, 1984, 1999, 2007; Warren, 1999, 2008; Cosenz and Noto, 2016; Bianchi, 2016). In this field, SD proved to be a useful method to understand the process of accumulation and depletion of strategic resources, as well as to design performance indicators consistent with organizational strategies and desired goals (Kunc and Morecroft, 2009; Bianchi, 2016; Kunc and O'Brien, 2017; Torres et al., 2017).
The characteristics outlined above suggest us that SD has the potential to foster the link between operation management and strategy design and implementation as remarked by the mainstream literature.
4. Combining Value Stream Mapping and system dynamics
The DVSM combines VSM and SD modelling to foster a strategic perspective in Lean Thinking applications. To do that, DVSM leverages on the strengths of both approaches.
In particular, DVSM embraces the systemic and dynamic view of SD in order to overcome the VSM's static perspective, as well as the lack of a systemic view pointed out by Solding and Gullander (2009) and Xie and Peng (2012). The static perspective is intended as the inability to understand how potential solutions – especially when many of them are designed and implemented simultaneously – affect the analysed process in terms of value waste reduction and performance improvement in the short, medium and long term (Stadnicka and Litwin, 2019). The lack of a systemic view in the process analysis refers to the exclusive focus on single product/service processes allowed by traditional VSM practices. For example, it may happen that improving the efficiency of a specific process, although requiring a significant effort, does not produce an equivalent impact on the overall organizational performance due to the constraints existing beyond the boundaries of the analysed process (Sterman, 2000; Wolstenholme, 2006).
Thus, DVSM borrows the simulation attribute from the SD methodology to allow decision-makers to experiment with the analysed process and test potential solutions aimed at improving its performance over time (El-Gafy and Abdelhamid, 2008; Stadnicka and Litwin, 2019). Simulation is indeed an effective practice to overcome the static perspective of VSM and discover how complex systems work when real experimentation is too slow or too costly (Sterman, 2014). Although several simulation approaches may support experimentation, SD modelling simulation better comply with the aim of fostering strategic management in Lean Thinking since, as remarked by Davies et al. (2007), it is an adequate approach to investigate how the inputs to a system of interconnected causal loops, stocks and flows – that is the case of a complex operational process – produce system outcomes.
As for the lack of a systemic view, the DVSM requires modellers to widen the boundaries of the analysis (Sterman, 2002), thus including the feedback mechanisms existing between the processes and the overall system performance. The adoption of a systemic view in managing organizational performance also entails the design of key performance indicators to measure the process performance and how this contributes to pursuing organizational goals (Bianchi, 2016). Both the design of indicators to monitor the process performance and the implementation of emerging solutions form a key momentum of every Lean application (Susilawati et al., 2013; Camacho-Miñano et al., 2013). However, as many scientists claim (Meyer and Gupta, 1994; Smith, 1995; Marr, 2006; Melnyk et al., 2013), the use of performance indicators may lead to the rise of unintended consequences whenever their design is not supported by a robust methodological perspective (e.g. compliance-based vs performance-based indicators, evaluation of specific sub-system results to the detriment of others, lack of long-term orientation). In the specific case of executing actions for process improvement, unintended consequences may show up when designed indicators delimit their scope to foster sub-optimal performance or organizational myopia. These methodological shortcomings are overcome by DVSM through the adoption of a systemic view – whose scope embraces all organizational sub-systems – that, according to Bianchi (2012, 2016), is pivotal to design key performance indicators consistent with the pursuit of the global organizational strategy over time. The systemic perspective adopted by the DVSM was extensively used to support the design and implementation of performance indicators aimed at measuring organizational performance in complex systems (Sterman, 2000; Morecroft, 2007; Bianchi, 2016; Oladimeji et al., 2020). This is due to its modelling characteristics that can help improve the deficiencies of static performance measures as it can model cause and effect relationships and dynamic behaviours over time (Forrester, 1958; Sterman, 2000).
The strengths of DVSM are mainly referred to its effective visual representation which facilitates decision-makers and other key-actors to understand how the process works, the time delays between interdependent operations, the process bottlenecks, the activities that add value for the customers and those failing to do so. Due to this, DVSM models adapt SD visual representation to VSM schemas. In this combined approach, SD provides a consistent graphical representation of the elements of a process (i.e. stock and flows) when compared with “traditional” VSM. In particular, process activities can be modelled as stocks, while flows capture the accumulation and consumption processes of those resources required to carry out a given activity over time. As such, stocks and associated flows enable to depict and frame the overall production process. Namely, once an activity is completed, the resulting semi-finished product – or, more generally, the intermediate output – flows into the following activity up to the end of the process that, usually, consists in an exchange with the external environment (e.g. a final product sold to customers).
Figure 2 shows how to model process activities through DVSM modelling.
Thus, DVSM maintains the visual power of VSM and allows decision-makers and other stakeholders to understand how the process works, identify value wastes, design and test strategic solutions aimed to improve its performance over time.
In the next section, an illustrative case of DSVM is developed to explore and discuss the potential advantages and risks associated with the use of such a combined approach.
5. The dynamic Value Stream Map: an illustrative case
In this section, an illustrative case of how to apply the DVSM is developed. The case focuses on an ambulatory care process within a healthcare organization. The choice of focusing on a healthcare organization is justified by the need to experiment with the proposed tool in a complex context (Bertolini et al., 2011). This case was proposed and analysed during a training course for health professionals and managers.
The first step in the adoption of the DVSM consists in modelling the current situation of the process being analysed. This is an ambulatory care process in which patients need to receive a specialist visit. In the process “as is”, all patients arrive at the clinic for receiving cares in a close period of time. Once they get to the clinic, they got registered, one by one, by a staff member. During the registration, they fill in the administrative documentation which is then delivered to the administrative office. The second phase of the clinical process is nurse evaluation. Once they have been evaluated by the nurse, patients are conducted to the laboratory to collect their urines. These are then carried by the patient to the specific laboratory which executes the examination. Once exams are ready, patients are visited by the medical doctor. If needed, patients receive a medicine prescription which will be provided by the clinic pharmacy. After this phase, patients are discharged. During each phase, the professionals in charge need to interact with the administrative office to collect the documentation produced during the previous care phases. In the meanwhile, the accounting department prepares the documentation required to issue the invoice (on average 200 euros per treatment) which is ready once the medical doctors and pharmacy deliver the specific pieces of information.
This “as is” process is represented in the DVSM portrayed in Figure 3.
As one may notice, the VSM modelled through SD reports on the bottom part the clinical process according to a “pull logic” (i.e. the patient perspective) distinguishing the various phases through which the patients should flow. In the top side of the figure, it is reported the off-line of the process which, in this case, corresponds to the administrative process that complements the clinical one.
By simulating the process, we notice that in 150 minutes the clinic is able to manage three patients (the ones managed to finish the clinical process), over a demand of five people, collecting about 600 euros of revenues Figure 4 and 5.
Two indicators are computed to represent the process performance. These are: (1) the average time of the process, which is equal to 92 minutes and (2) the average waiting time for patients to get registered which is about 8 minutes.
The solutions proposed to improve this process were collected by the health professionals and managers during the training course. These are: planning the visits so as to reduce the waiting time for the visits; asking the patients to come with their urines already collected to eliminate one of the process phases; investing in ICTs and informatization to reduce the time related to the interaction with the administrative office during each phase.
The DVSM “To be” is reported in Figure 6.
Simulation allows us to test these solutions before their actual implementation. The number of patients completing the clinical procedure is five compared to three reported in the previous process. Consequently, the revenues collected amounts to about 1.000 euro. For what concerns the identified performance indicators, the process time is reduced from 92 minutes to 65. Moreover, the average waiting time before starting the process is now lower than 1 minute, while in the previous scenario was equal to 8 minutes Figures 7 and 8.
Through the simulation of this process, we may notice that the proposed solutions enable the improvement in terms of both end-results (i.e. number of patients processed and revenues collected) and performance indicators (i.e. average process time and average waiting time to start the process). The simulation thus supports to experiment operational results before implementing solutions in the real world.
As previously argued, SD models may also empower a strategic view of processes performance (Cosenz and Noto, 2016; Bianchi, 2016). In particular, by conceiving the simulated process as a part of a wider system (i.e. the organization) in which the achieved outputs foster the process inputs in the medium/long term, it is also possible to undertake strategic actions.
In the previous analysis, we focused on the relationship between the process efficiency and quality and the revenues, demonstrating that the proposed solutions can increase revenues through the process improvement. If we adopt a wider view, we may assume that the improvement of the performance indicators fosters the demand of patients that are more satisfied with their care experience. Through the word-of-mouth effect, this will generate a positive effect on the total revenues collected. However, an increase in the demand for the service may negatively affect the process efficiency and quality perceived by the patients if the supply is not improved accordingly. Therefore, the healthcare organization is called to re-invest part of the increase in revenues to improve the process itself. Figure 9 shows the functioning of the system. In particular, the model consists of two feedback loops. A reinforcing loop (process efficiency and quality→ service demand → revenues → resources) portrays the virtuous circle that fosters the growth of the organizational system. On the other hand, the balancing loop (process efficiency and quality → service demand) mitigates this growth accounting for the effect of the service demand on the process efficiency and quality.
Adding this insight into the DVSM may allow us to explore also strategic initiatives. For example, with the intent to consider an expected growth in the service demand, the healthcare organization may experiment to invest the revenues in an increase of the medical staff (which is in charge of the longest phase of the process).
The dotted line in Figure 10 represents the strategic relationships that close the loops described in Figure 9. The result is an increase in examined patients and, as a result, additional revenues to be reinvested in the process. Once the process is improved in order to meet the increase in care demand, future additional revenues could also be invested in hiring other staff positions, thus leveraging on the other performance driver, i.e. the average waiting time for registration. Through this model, it is possible to experiment with the amount of additional revenues to invest in the process and to test the related parameters and sensitiveness. Eventually, as Figure 10 shows, the model uses the same graphical representation of VSM, thereby maintaining its visual strength.
6. Discussion
VSM is a powerful tool to foster process innovation and operation management within an organization (Rother and Shook, 1998; Serrano Lasa et al., 2008; Karim and Arif-Uz-Zaman, 2013). In particular, it aims to provide a graphical representation of a specific organizational process forming a consistent ground to promote possible improvements.
Among the weaknesses of VSM, the literature identifies the lack of a dynamic and systemic view of the processes which may represent a barrier to the adoption of a strategic perspective for the organization. In order to overcome this limit, this paper proposes to combine the VSM with SD modelling. This last is suggested by the authors because it is characterized by four key features that make it suitable to foster a strategic view in the design and use of VSM. First, SD allows one to simulate a system behaviour and to experiment with the model potential process improvements, thus addressing the lack of a dynamic view previously mentioned. Second, SD models conceive complex systems as a closed chain of feedback loops. This pushes the business analyst to adopt a systemic view of the process so as to include all the relevant variables in the analysis. Third, SD modelling enables to link the structural characteristics of a system with its behaviours. Moreover, SD modelling representation is highly adaptable to traditional VSM visual schemas and, thus, maintains its visual strength oriented to facilitate a shared understanding of the system among decision-makers and other stakeholders.
SD makes VSM “dynamic” through the mean of simulation. As in flight-simulator games, decision-makers may simulate the outputs of proposed changes aimed to improve the processes so as to assess whether their implementation would produce the expected results over time. In fact, in complex processes, it is not possible to estimate and quantify the results of proposed improvements before their real implementation. However, in some cases, such an implementation may be too costly in terms of time and money, and thus decision-makers assume the risk that the benefits coming from one or more changes do not cover the effort of their implementation (Sterman, 2014). Simulation supports decision-makers in undertaking this kind of decisions by quantifying the expected results of potential improvement-oriented solutions. In the case reported in Section 4, the ambulatory care process analysed was initially mapped adapting SD modelling to the traditional VSM representation and simulating its performance. Through this representation and the emerging simulation, it was possible to identify potential solutions for improvement. The DVSM “as is” was then modified to experiment with these solutions, and a new DVSM “to be” was built and simulated. By comparing the simulations coming from the two models, it was possible to envisage a positive result in term of process performance measured through key performance indicators identified.
At the same time, the SD approach also fosters a systemic view of the process since it asks the analyst to “challenge the clouds” (Sterman, 2002) – i.e. to shift the boundaries of a system until all the key characteristics of the analysed environment influencing the system behaviour are analysed. Therefore, in addition to considering the elements characterizing the process performance, DVSM includes all those variables which link the process performance to the key variables of the organizational system. This supports a strategic view of the analysed process. Such a systemic view is also key to support the design of performance measures consistent with the overall organizational goals (Bianchi, 2016; Cosenz and Noto, 2016) and to assess whether the used ones may spur dysfunctional performance such as sub-optimization or myopia (Smith, 1995).
For example, in the illustrative case developed, it emerged the need to include in the analysis the effects of the adopted performance indicators on the service demand to check whether their improvement would produce poor performance results in the long run. In fact, the improved performance would possibly lead to an increase in the service demand which, in turn, would make again the process non-efficient and non-effective in order to satisfy the needs of the potential users. The DVSM was thus reviewed to include this strategic issue which was modelled by “closing the loop” between the results produced by the process performance (i.e. the outputs) and alternative use of its strategic resources (i.e. the inputs).
The DVSM approach also supports the adoption of a systemic view compared to traditional VSM since allowing analysts to map and simulate multiple organizational processes to explore how these influence each other and support the achievement of the organization's strategic goals. This feature distinguishes SD from other simulation-based approaches. In fact, as supported by Repenning (2002), the SD approach can reveal the outcomes of the interactions among multiple underlying organizational processes, especially as they unfold over time.
Eventually, SD graphical representation is highly adaptable to VSM. Although the visual characteristics of management tools may be perceived as less important than other characteristics, both operation management literature (Parry and Turner, 2006; Serrano Lasa et al., 2008; Tezel et al., 2016) and management control one (Busco and Quattrone, 2015) confirm its role in supporting processes understanding, organizational change, communication and people's motivation. Thus, maintaining the visual strength of VSM is a key feature that simulation techniques should guarantee to effectively support its improvement.
Limits of DVSM are related to the inner characteristics of SD. This is indeed a methodology aimed at comprehending the overall dynamic behaviour of a system, rather than performing a precise quantitative prediction (Meadows, 1980). When simulating specific organizational processes in which precise computation of parameters is required, this characteristic may represent a limit. In these cases, a solution could be represented by the combination of SD with other simulation techniques, such as DES. However, it is important to manage the trade-off between the additional computational effort, and the benefit in terms of model output that may be achieved.
7. Implications for practice
The implications for practice emerging from the study refer to two main areas: (1) the possibility to experiment with the models and (2) the definition of performance indicators consistent with the overall organizational strategy.
According to Grosser and Jovy (2016), SD simulation allows experimenting with multiple strategic settings limiting inaccurate management decision, identifying overlooked factors and patterns that could turn out to be relevant in the future. In complex and dynamic organizational settings, the consequence of mistakes can be fatal for business survival. More subtly, in many organizational systems, the local and distal, and short- and long-term impacts of decisions differ: what works here and now may harm the organization elsewhere and later (Repenning and Sterman, 2002; Sterman, 2014). Simulations offer a resolution to this issue since it can compress or expand time and space, allowing decision-makers to simulate months or years in the life of an organization in a few minutes. Simulation becomes the only way to discover how complex systems work and where high leverage points may lie (Sterman, 2014).
The definition of performance indicators is a complex task highly debated in the literature (Melnyk et al., 2013). On the one hand, performance measures help in guiding organizations towards desired end-results; on the other hand, poorly defined performance measure may drive towards dysfunctional performance. According to Bianchi (2012) the adoption of a systemic view through the use of SD supports decision-makers in defining measures that foster sustainable organizational performance. Practitioners may thus benefit from the adoption of DVSM when designing performance measures aimed at assessing processes performance.
8. Implications for theory
The contribution to theory resulting from this study is related to the introduction of a new combined approach, i.e. DVSM, aimed at tackling the gap between operations and organizational strategy. Both SD and Lean Thinking have in common the aim of improving thinking for change and both play important roles to foster organizational performance. Although distinct in their sphere of application, as supported by Wolstenholme (2006), they can be easily combined to achieve more than either alone. In fact, on the one hand, the adoption of Lean Thinking pursues the improvement of processes, organizational well-being and the creation of value for user; on the other hand, SD ensures that the proposed changes are aligned with the organizational strategy and become sustainable in the long-term (Poles, 2013). SD has been identified as an adequate method to support Lean Thinking in fostering a strategic approach due to its characteristics, namely simulation, systemic view, explicit link between systems structure and behaviour and effective visual representation. While in the analysis we focused on the combination between SD and a specific tool of Lean Thinking, i.e. VSM, further analysis may suggest extending such an approach to other tools and techniques proper of Lean Thinking and operation management.
The limitations of this research are mainly related to the focus on a single case study which limits the possibility of generalizing the results. In fact, although the illustrative case focused on a complex case (i.e. a healthcare ambulatory process), comparisons with other cases and other sectors were not tackled. Thus, further research perspectives may investigate other DVSM applications by analysing multiple experiences or cases from different contexts and industries (e.g. manufacturing, other services). Further researches may be aimed at combining SD with DES in VSM application to improve the simulation results and explore the trade-off between analytical efforts and corresponding benefits. Moreover, additional research perspectives can be oriented to explore how SD may foster the adoption of a strategic perspective when combined with other Lean Thinking and operation management practices.
Figures
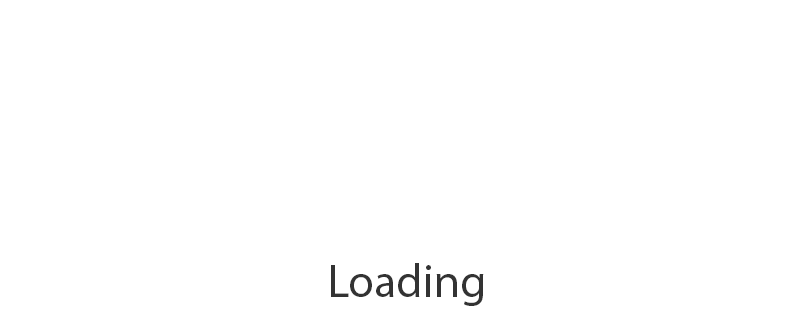
Figure 1
The VSM framework (Norton and Fearne, 2009)
References
Abo Hamad, W., Crowe, J. and Arisha, A. (2012), “Towards leaner healthcare facility: application of simulation modelling and Value Stream Mapping”, International Workshop on Innovative Simulation for Healthcare (I-WISH), Vienna.
Balocco, R., Cavallo, A., Ghezzi, A. and Berbegal-Mirabent, J. (2019), “Lean business models change process in digital entrepreneurship”, Business Process Management Journal, Vol. 25 No. 7, pp. 1520-1542.
Barnabè, F. (2011), “A ‘system dynamics-based balanced scorecard’ to support strategic decision making”, International Journal of Productivity and Performance Management, Vol. 60 No. 5, pp. 446-473.
Barnabè, F., Guercini, J. and Di Perna, M. (2019), “Assessing performance and value-creation capabilities in Lean healthcare: insights from a case study”, Public Money and Management, Vol. 39 No. 7, pp. 503-511.
Bashin, S. and Burcher, P. (2006), “Lean viewed as a philosophy”, Journal of Manufacturing Technology Management, Vol. 17 No. 1, pp. 56-72.
Bertolini, M., Bevilacqua, M., Ciarapica, F.E. and Giacchetta, G. (2011), “Business process re-engineering in healthcare management: a case study”, Business Process Management Journal, Vol. 17 No. 1, pp. 42-66.
Bianchi, C. (2012), “Enhancing performance management and sustainable organizational growth through system-dynamics modelling”, in Grösser, S.N. and Zeier, R. (Eds), Systemic Management for Intelligent Organizations, Springer, Berlin, pp. 143-161.
Bianchi, C. (2016), Dynamic Performance Management, Springer, Berlin.
Bianchi, C. and Montemaggiore, G.B. (2008), “Enhancing strategy design and planning in public utilities through ‘dynamic’ balanced scorecards: insights from a project in a city water company”, System Dynamics Review, Vol. 24 No. 2, pp. 175-213.
Bianchi, C., Winch, W. and Cosenz, F. (2018), “Experimenting lean dynamic performance management systems design in SMEs”, International Journal of Productivity and Performance Management, Vol. 67 No. 7, pp. 1234-1251.
Busco, C. and Quattrone, P. (2015), “Exploring how the balanced scorecard engages and unfolds: articulating the visual power of accounting inscriptions”, Contemporary Accounting Research, Vol. 32 No. 3, pp. 1236-1262.
Camacho-Miñano, M.D.M., Moyano-Fuentes, J. and Sacristan-Diaz, M. (2013), “What can we learn from the evolution of research on lean management assessment?”, International Journal of Production Research, Vol. 51 No. 4, pp. 1098-1116.
Cavdur, F., Yagmahan, B., Oguzcan, E., Arslan, N. and Sahan, N. (2019), “Lean service system design: a simulation-based VSM case study”, Business Process Management Journal, Vol. 25 No. 7, pp. 1802-1821.
Cookson, D., Read, C., Mukherjee, P. and Cooke, M. (2011), “Improving the quality of emergency department care by removing waste using lean Value Stream Mapping”, International Journal of Clinical Leadership, Vol. 17 No. 1, pp. 25-30.
Cosenz, F. and Noto, G. (2016), “Applying system dynamics modelling to strategic management: a literature review”, Systems Research and Behavioral Science, Vol. 33 No. 6, pp. 703-741.
Cosenz, F. and Noto, G. (2018a), “Fostering entrepreneurial learning processes through Dynamic Start-up business model simulators”, The International Journal of Management Education, Vol. 16 No. 3, pp. 468-482.
Cosenz, F. and Noto, G. (2018b), “A dynamic business modelling approach to design and experiment new business venture strategies”, Long Range Planning, Vol. 51 No. 1, pp. 127-140.
Cosenz, F., Rodrigues, V.P. and Rosati, F. (2020), “Dynamic business modeling for sustainability: exploring a system dynamics perspective to develop sustainable business models”, Business Strategy and the Environment, Vol. 29, No. 2, pp. 651-664.
Curatolo, N., Lamouri, S., Huet, J.C. and Rieutord, A. (2014), “A critical analysis of Lean approach structuring in hospitals”, Business Process Management Journal, Vol. 20 No. 3, pp. 433-454.
Davidsen, P. (1991), ‘The Structure-Behavior Graph’, System Dynamics Group, MIT Press, Cambridge, MA.
Davis, J.P., Eisenhardt, K.M. and Bingham, C.B. (2007), “Developing theory through simulation methods”, Academy of Management Review, Vol. 32 No. 2, pp. 480-499.
Doğan, N.Ö. and Unutulmaz, O. (2016), “Lean production in healthcare: a simulation-based Value Stream Mapping in the physical therapy and rehabilitation department of a public hospital”, Total Quality Management and Business Excellence, Vol. 27 Nos 1-2, pp. 64-80.
Eisenhardt, K.M. and Graebner, M.E. (2007), “Theory building from cases: opportunities and challenges”, Academy of Management Journal, Vol. 50 No. 1, pp. 25-32.
El-Gafy, M.A. and Abdelhamid, T. (2008), “Using system dynamics modeling as a lean construction work structuring tool”, Proceedings at IGLC, Vol. 16.
El-Haik, B. and Al-Aomar, R. (2006), Simulation-based Lean Six-Sigma and Design for Six-Sigma, Wiley-Interscience, Hoboken, NJ.
Erikshammar, J., Lu, W., Stehn, L. and Olofsson, T. (2013), “Discrete event simulation enhanced Value Stream Mapping: an industrialized construction case study”, Lean Construction Journal, Vol. 10, pp. 47-65.
Forrester, J.W. (1958), Industrial Dynamics, MIT Press, Cambridge.
Furnari, S. (2015), “A cognitive mapping approach to business models: representing causal structures and mechanisms”, Advances in Strategic Management, Vol. 33 No. 1, pp. 207-239.
Garnett, N., Jones, D.T. and Murray, S. (1998), “Strategic application of lean thinking”, Proceedings IGLC, Vol. 98, pp. 1-12.
Ghaffarzadegan, N., Lyneis, J. and Richardson, G.P. (2011), “How small system dynamics models can help the public policy process”, System Dynamics Review, Vol. 27 No. 1, pp. 22-44.
Groesser, S.N. and Jovy, N. (2016), “Business model analysis using computational modeling: a strategy tool for exploration and decision-making”, Journal of Management Control, Vol. 27, pp. 61-88.
Größler, A., Thun, J.H. and Milling, P. (2008), “System dynamics as a structural theory in operations management”, Production and Operations Management, Vol. 17 No. 3, pp. 373-384.
Hines, P., Holweg, M. and Rich, N. (2004), “Learning to evolve: a review of contemporary lean Thinking”, International Journal of Operations and Production Management, Vol. 24 No. 10, pp. 994-1011.
Holden, R.J. (2011), “Lean thinking in emergency departments: a critical review”, Annals of Emergency Medicine, Vol. 57 No. 3, pp. 265-278.
Julien, D.M. and Tjahjono, B. (2009), “Lean thinking implementation at a safari park”, Business Process Management Journal, Vol. 15 No. 3, pp. 321-335.
Karim, A. and Arif-Uz-Zaman, K. (2013), “A methodology for effective implementation of lean strategies and its performance evaluation in manufacturing organizations”, Business Process Management Journal, Vol. 19 No. 1, pp. 169-196.
Kunc, M. and Morecroft, J.D. (2009), “Resource-based strategies and problem structuring: using resource maps to manage resource systems”, Journal of the Operational Research Society, Vol. 60 No. 2, pp. 191-199.
Kunc, M. and O'Brien, F.A. (2017), “Exploring the development of a methodology for scenario use: combining scenario and resource mapping approaches”, Technological Forecasting and Social Change, Vol. 124, pp. 150-159.
Marr, B. (2006), Strategic Performance Management: Leveraging and Measuring Your Intangible Value Drivers, Butterworth-Heinemann, Oxford.
McDonald, T., Van Aken, E.M. and Rentes, A.F. (2002), “Utilising simulation to enhance Value Stream Mapping: a manufacturing case application”, International Journal of Logistics, Vol. 5 No. 2, pp. 213-232.
Meadows, D. (1980), “The unavoidable a priori”, in Randers, J. (Ed.), Elements of the System Dynamics Method, Pegasus Communications, Waltham, MA.
Melnyk, S.A., Bititci, U., Platts, K., Tobias, J. and Andersen, B. (2013), “Is performance measurement and management fit for the future?”, Management Accounting Research, Vol. 25 No. 2, pp. 173-186.
Meyer, M.W. and Gupta, V. (1994), “The performance paradox”, Research in Organizational Behavior, Vol. 16, pp. 309-369.
Michael, C.W., Naik, K. and McVicker, M. (2013), “Value stream mapping of the Pap test processing procedure: a lean approach to improve quality and efficiency”, American Journal of Clinical Pathology, Vol. 139 No. 5, pp. 574-583.
Mingers, J. and White, L. (2010), “A review of the recent contribution of systems thinking to operational research and management science”, European Journal of Operational Research, Vol. 207 No. 3, pp. 1147-1161.
Morecroft, J.D.W. (1984), “Strategy support models”, Strategic Management Journal, Vol. 5 No. 3, pp. 215-229.
Morecroft, J.D.W. (1999), “Visualising and rehearsing strategy”, Business Strategy Review, Vol. 10 No. 3, pp. 17-32.
Morecroft, J.D.W. (2007), Strategic Modeling and Business Dynamics, Wiley, Chichester.
Norton, A. and Fearne, A. (2009), “Sustainable Value Stream Mapping in the food industry”, Handbook of Waste Management and Co-product Recovery in Food Processing, Woodhead Publishing, pp. 3-22.
Ohno, T. (1988), The Toyota Production System: Beyond Large-Scale Production, Productivity Press, Portland, OR.
Oladimeji, O.O., Keathley-Herring, H. and Cross, J.A. (2020), “System dynamics applications in performance measurement research”, International Journal of Productivity and Performance Management, Vol. 69 No. 7, pp. 1541-1578.
Parry, G.C. and Turner, C.E. (2006), “Application of lean visual process management tools”, Production Planning and Control, Vol. 17 No. 1, pp. 77-86.
Pavnaskar, S.J., Gershenson, J.K. and Jambekar, A.B. (2003), “Classification scheme for Lean manufacturing tools”, International Journal of Production Research, Vol. 41 No. 13, pp. 3075-3090.
Poles, R. (2013), “System Dynamics modelling of a production and inventory system for remanufacturing to evaluate system improvement strategies”, International Journal of Production Economics, Vol. 144 No. 1, pp. 189-199.
Radnor, Z. and Osborne, S.P. (2013), “Lean: a failed theory for public services?”, Public Management Review, Vol. 15 No. 2, pp. 265-287.
Repenning, N.P. (2002), “A simulation-based approach to understanding the dynamics of innovation implementation”, Organization Science, Vol. 13 No. 2, pp. 109-127.
Repenning, N.P. and Sterman, J. (2002), “Capability traps and self-confirming attribution errors in the dynamics of process improvement”, Administrative Science Quarterly, Vol. 47 No. 2, pp. 265-295.
Robinson, S., Radnor, Z.J., Burgess, N. and Worthington, C. (2012), “SimLean: utilising simulation in the implementation of lean in healthcare”, European Journal of Operational Research, Vol. 219 No. 1, pp. 188-197.
Rother, M. and Shook, J. (1998), Learning to See: Value Stream Mapping to Add Value and Eliminate Muda, The Lean Enterprise Institute, MA.
Schieritz, N. and Milling, P.M. (2003), “Modeling the forest or modeling the trees A comparison of system dynamics and agent-based simulation”, Proceedings of the 2003 International System Dynamics Conference, System Dynamic Society, New York, NY.
Serrano Lasa, I., Ochoa Laburu, C. and de Castro Vila, R. (2008), “An evaluation of the Value Stream Mapping tool”, Business Process Management Journal, Vol. 14 No. 1, pp. 39-52.
Shamah, R. (2013), “A model for applying lean thinking to value creation”, International Journal of Lean Six Sigma, Vol. 4 No. 2, pp. 204-224.
Shararah, M.A., El-Kilany, K.S. and El-Sayed, A.E. (2010), “Component based modeling and simulation of Value Stream Mapping for lean production systems”, FAIM Conference, pp. 881-888.
Shararah, M.A., El-Kilany, K.S. and El-Sayed, A.E. (2011), “Value stream map simulator using extendsim”, Lecture Notes in Engineering and Computer Science: Proceedings of the World Congress on Engineering, WCE 2011, 6-8 July, 2011, London, pp. 755-758.
Smith, P. (1995), “On the unintended consequences of publishing performance data in the public sector”, International Journal of Public Administration, Vol. 18 Nos 2-3, pp. 277-310.
Solding, P. and Gullander, P. (2009), “Concepts for simulation based Value Stream Mapping”, Proceedings of the 2009 WinterSimulation Conference (WSC), Austin, TX, IEEE, pp. 2231-2237.
Stadnicka, D. and Litwin, P. (2019), “Value stream mapping and system dynamics integration for manufacturing line modelling and analysis”, International Journal of Production Economics, Vol. 208, pp. 400-411.
Sterman, J.D. (2000), Business Dynamics: System Thinking and Modelling for a Complex World, McGraw-Hill, London.
Sterman, J.D. (2002), “All models are wrong: reflections on becoming a systems scientist”, System Dynamics Review, Vol. 18 No. 4, pp. 501-531.
Sterman, J. (2014), “Interactive web‐based simulations for strategy and sustainability: the MIT sloan learningedge management flight simulators, Part I”, System Dynamics Review, Vol. 30 Nos 1-2, pp. 89-121.
Suarez-Barraza, M.F., Miguel-Davila, J.Á. and Vasquez-García, C.F. (2016), “Supply chain Value Stream Mapping: a new tool of operation management”, International Journal of Quality and Reliability Management, Vol. 33 No. 4, pp. 518-534.
Susilawati, A., Tan, J., Bell, D. and Sarwar, M. (2013), “Develop a framework of performance measurement and improvement system for lean manufacturing activity”, International Journal of Lean Thinking, Vol. 4 No. 1, pp. 51-64.
Swallmeh, E., Tobail, A., Abo-Hamad, W., Gray, J. and Arisha, A. (2014), “Integrating simulation modelling and Value Stream Mapping for leaner capacity planning of an emergency department”, Sixth International Conference on Advances in System Simulation, SIMUL 2014, Nice, pp. 256-262.
Tezel, A., Koskela, L. and Tzortzopoulos, P. (2016), “Visual management in production management: a literature synthesis”, Journal of Manufacturing Technology Management, Vol. 27 No. 6, pp. 766-799.
Tobail, A., Egan, P., Abo-Hamad, W. and Arisha, A. (2013), “Application of lean Thinking using simulation modelling in a private hospital”, Fifth International Conference on Advances in System Simulation, SIMUL 2013, Venice, pp. 22-28.
Torres, J.P., Kunc, M. and O'Brien, F. (2017), “Supporting strategy using system dynamics”, European Journal of Operational Research, Vol. 260 No. 3, pp. 1081-1094.
Warren, K. (1999), “The dynamics of strategy”, Business Strategy Review, Vol. 10 No. 3, pp. 1-16.
Warren, K. (2008), Strategic Management Dynamics, Wiley, Chichester.
Wolstenholme, E. (1999), “Qualitative vs quantitative modelling: the evolving balance”, Journal of the Operational Research Society, Vol. 50, pp. 422-428.
Wolstenholme, E. (2006), “Thoughts on the complementarity of lean thinking and system thinking for global service improvement in health and social care”, available at: http://www.symmetricpartnership.co.uk/userfiles/Documents/8Lean_Methods_and_System_Dynamics.pdf (accessed 9 June 2020).
Womack, J.P. and Jones, D.T. (1996), Lean Thinking: Banish Waste and Create Wealth in Your Corporation, Simon and Schuster, New York, NY.
Womack, J.P., Jones, D.T. and Roos, D. (1990), The Machine that Changed the World, Rawson Associates, New York, NY.
Xie, Y. and Peng, Q. (2012), “Integration of Value Stream Mapping and agent-based modeling for OR improvement”, Business Process Management Journal, Vol. 18 No. 4, pp. 585-599.
Acknowledgements
The authors would like to thanks Caterina Bianciardi and Jacopo Guercini for the preliminary discussion on the topic explored in the article. We also thank the two anonymous reviewers whose comments and suggestions helped improve this manuscript.
Corresponding author
About the authors
Guido Noto is an assistant professor of Business & Public Management at the University of Messina, Italy. He holds an International PhD in “Model Based Public Planning, Policy Design, and Management” at the University of Palermo. Before covering the current position, he was post-doc at the Management and Health Laboratory of Sant'Anna School of Advanced Studies in Pisa. His research interests are related to Performance Management in both the private and the public sector.
Federico Cosenz is an associate professor of Business & Public Management at the University of Palermo, Italy. He holds an International PhD in “Model Based Public Planning, Policy Design, and Management”. He has published on several academic and professional journals. His main research interests focus on Business Model Design, Small Business Growth Management, Business Strategy, Performance Management Systems, Simulation for Business Planning and Strategic Organizational Learning.