Abstract
Purpose
This research proposes to organise and distil this massive amount of data, making it easier to understand. Using data mining, machine learning techniques and visual approaches, researchers and managers can extract valuable insights (on guests' preferences) and convert them into strategic thinking based on exploration and predictive analysis. Consequently, this research aims to assist hotel managers in making informed decisions, thus improving the overall guest experience and increasing competitiveness.
Design/methodology/approach
This research employs natural language processing techniques, data visualisation proposals and machine learning methodologies to analyse unstructured guest service experience content. In particular, this research (1) applies data mining to evaluate the role and significance of critical terms and semantic structures in hotel assessments; (2) identifies salient tokens to depict guests' narratives based on term frequency and the information quantity they convey; and (3) tackles the challenge of managing extensive document repositories through automated identification of latent topics in reviews by using machine learning methods for semantic grouping and pattern visualisation.
Findings
This study’s findings (1) aim to identify critical features and topics that guests highlight during their hotel stays, (2) visually explore the relationships between these features and differences among diverse types of travellers through online hotel reviews and (3) determine predictive power. Their implications are crucial for the hospitality domain, as they provide real-time insights into guests' perceptions and business performance and are essential for making informed decisions and staying competitive.
Originality/value
This research seeks to minimise the cognitive processing costs of the enormous amount of content published by the user through a better organisation of hotel service reviews and their visualisation. Likewise, this research aims to propose a methodology and method available to tourism organisations to obtain truly useable knowledge in the design of the hotel offer and its value propositions.
Keywords
Citation
Sánchez-Franco, M.J. and Rey-Tienda, S. (2023), "The role of user-generated content in tourism decision-making: an exemplary study of Andalusia, Spain", Management Decision, Vol. ahead-of-print No. ahead-of-print. https://doi.org/10.1108/MD-06-2023-0966
Publisher
:Emerald Publishing Limited
Copyright © 2023, Manuel J. Sánchez-Franco and Sierra Rey-Tienda
License
Published by Emerald Publishing Limited. This article is published under the Creative Commons Attribution (CC BY 4.0) licence. Anyone may reproduce, distribute, translate and create derivative works of this article (for both commercial and non-commercial purposes), subject to full attribution to the original publication and authors. The full terms of this licence may be seen at http://creativecommons.org/licences/by/4.0/legalcode
1. Introduction
Tourism in Andalusia (Spain) is relevant due to its impact on regional production and employment. Tourism is, in fact, its first industry, representing 6.5% of its GDP in 2021 (Δ 35.8 % and Δ 49.8% of tourist flow compared to 2020). Furthermore, according to the Hotel Occupancy Survey (National Statistics Institute, 2022), Andalusia recorded 5,682,276 hotel overnight stays in September 2022 (1,961,146 travellers), notably advancing from 4,696,783 overnight stays in September 2021 (1,579,073 travellers). The key to tourism in Andalusia is also contextualised in the growing use of information and communication technologies (ICTs) to consult, book or purchase tourism services, mainly hotel services. Five years ago, in 2017, 61.2% of tourists reported using ICT to consult, make a reservation or purchase services during their trip to Andalusia, up 4% compared to the previous year (Balance of the Year of Tourism in Andalusia, 2017). Therefore, its use represents a critical opportunity for the sector. For instance, ICTs increase the possibility of cost reduction by eliminating the intermediation relationship and the availability of new (and ubiquitous) communication channels between tourists and organisations. Furthermore, ICTs transmit diversity, wealth, quality, safety, complementarity and differentiation. According to the Andalusia Horizon 2020 General Plan for Sustainable Tourism in Andalusia, this development has changed how tourists make their decisions due to the exchange of opinions and experiences through applications, social networks and other spaces on the Internet.
In this sense, the hospitality domain is evolving towards other models based on the publication and consultation of user-generated content (UGC) in online booking systems (Raguseo et al., 2017; Sparks et al., 2016; Lyu et al., 2022). Guests seek advice before booking a hotel. They consult reviews published on information-mediation platforms (e.g. Booking, TripAdvisor, Expedia and Yelp, among others) and assess the ratings of other guests about their stays in hotel establishments. Online reviews are spontaneous, enlightening and even passionate, easily accessible from anywhere and at any time (Alarcón-Urbistondo et al., 2023; Guo et al., 2016; Zhu et al., 2020). Reviews are memories or cognitive reconstructions of a trip or stay that appear to reduce the potential risk of purchase noticed by other users (Sparks et al., 2016). Although shared content that recreates guest experiences can be distorted, the information is perceived as credible.
Consequently, our research seeks to extend the published research on information-mediation platforms by applying an interpretation framework based on the discipline of relationship marketing. Our analysis proposes to identify critical terms and topics inferred from the unstructured content generated by the guests on the services demanded by the guests of hotel establishments. Therefore, our methodology uses a multifaceted approach, merging both qualitative and quantitative research paradigms. It uses an automated tracking system for data collection, where hotel, review and guest data are recorded from specified locations. Our analysis of these data includes structured and unstructured elements, with an emphasis on user-generated reviews as a key source of information.
Our study delves into the complex analytical environment of tourism in Andalusia, focussing primarily on the intricate interplay of features that affect the hospitality industry and guest satisfaction by understanding needs, providing reliable service and building trust (relationship quality, hereinafter, RQ; cf. classic texts such as those of Gundlach et al., 1995; Morgan and Hunt, 1994; Parasuraman and Grewal, 2000). Our study aims to examine the role of ICTs in shaping consumer behaviour and their consequent influence on hotel services and demand. Our research further investigates the correlation between variables associated with guests, e.g. traveller type and length of stay, and the narratives produced by the guests, with a substantial reliance on data visualisation as an analytical tool.
Moreover, our research employs the graphical visualisation of results. Data visualisation is a fundamental tool for analysing and understanding guest reviews. It enables users to effectively and quickly interpret data, helping decision-making and identifying patterns and trends. Visual representation of data is also more accessible to the human brain. Specialised visualisation tools are necessary to effectively explore and understand data, assuming that traditional data analysis methods can be inefficient and impractical for handling large datasets. However, there are potential limitations in using data visualisation. In particular, the reliance on data visualisation may lead to the oversimplification of complex data, resulting in biased or incomplete conclusions. To avoid these mistakes, our research starts by defining its objectives before collecting and preparing its data for visualisation. In addition, our approach concludes the importance of choosing the appropriate chart type for the data and message and ensures that the visualisation is legible and easy to understand.
In summary, our research seeks (1) to minimise the cognitive processing costs of the enormous amount of content published by the user through a better organisation of hotel service reviews and their visualisation, and (2) to propose a methodology and method available to tourism organisations to obtain truly useable knowledge in the design of the hotel offer and its value propositions. Our selected statistical analysis also includes various algorithms to identify the semantic structures behind UGC. These include Scattertext for highlighting the most salient terms, word shifts graphs for visualising text comparisons and Non-Negative Matrix Factorisation (NMF) for topic modelling. Finally, our research validates results with various statistical measures, including the Matthews correlation coefficient (MCC) and SHapley Additive exPlanations (SHAP). The Research Method section provides details of the data gathering, data mining and findings on hotel stays. Finally, the Discussion section presents future research lines and theoretical and managerial implications.
2. Theoretical framework
The RQ refers to the general assessment of the strength of a relationship and is often associated with relationship satisfaction, trust and commitment. RQ is conceived as a suitable approach to explain and predict a relationship's success and is based here on commitment theory in business relationships (cf. Gundlach et al., 1995; Morgan and Hunt, 1994; Parasuraman and Grewal, 2000). In the context of the hotel industry, RQ is defined as the extent to which a hospitality relationship is able to fulfil the needs of guests; that is, high relational quality can lead to increased guest satisfaction as it often involves understanding and meeting the guest's needs and expectations, providing reliable and consistent service, and building a sense of commitment between the hotel and the guest (Mody et al., 2019). In this regard, commitment theory posits that the more committed a guest is to a hotel, the more likely they are to continue doing business with the hotel, for example, repeat bookings, positive reviews related to hospitality features and word-of-mouth recommendations.
In particular, in a relational context, when comparing the results of hospitality services with guests' expectations, satisfaction could be conceptualised as the guest's sensation of pleasure or disappointment resulting from a stay. Satisfaction, which is related to the service provider's performance, is a key measure of a hotel's effectiveness at outperforming other hospitality services. According to a cognitive approach, satisfaction is also formulated as the affective response to the congruence between the result and the standard of comparison (the disconfirmation of expectations model, Oliver, 1997, 2010). Following the atmospheric proposal, our study could identify intangible features (accommodation ambience, among others) and tangible features (accommodation amenities, for example) that influence guests' pleasure, arousal and willingness to return. For example, Belarmino et al. (2019) conclude that room facilities are a dominant topic for hotel guests. Amenities attract guests who prefer the feeling of being home instead of staying in a conventional hotel. “Hotel amenities play a significant role in guests' decision-making processes and service experience […], including satisfaction” (Yu et al., 2022, p. 3168), which can even justify higher prices.
Thus, the expectations of guests, the characteristics-based evaluation, the emotional evaluation and sensory attributes play a crucial role in generating satisfaction (Bagozzi et al., 1999; Baker et al., 1992; Lazos and Steenkamp, 2005; Mudie et al., 2003; Oliver, 2010; Rodríguez and San Martín, 2008; Yu and Dean, 2001). And precisely, our research on the UGC helps hosts and hotel managers identify the reasons of guests and provide better services to improve guests' experience, hospitality service reputation, willingness to return and even willingness to accept a higher price. Firstly, the literature on hotel guest satisfaction and dissatisfaction highlights key findings with important implications for hotel managers (e.g. Sann et al., 2022). Accessibility or standardisation are critical factors for short stays, i.e. being within walking distance of major attractions such as the city centre and transportation hubs and having lower risks associated with the hotel. The proximity allows guests to save time and effort on the commute, thus enhancing their overall travel experience. Following Sánchez-Franco and Aramendia-Muneta (2023), “guests emphasise drivers such as location and accessibility (e.g. walking distance from major attractions such as the city centre, the transportation hub, or the beach), lower risks–through standardisation, regulations, and reputation (…)”.
A hotel located near a metro station provides guests with the convenience of exploring different parts of the city with ease. Easy access to transportation options (for instance, public transport or car parking) thus becomes an essential factor in a guest's decision-making process. Moreover, location can be a determinant of perceived safety and security–hotels in more central or high-footfall areas are often seen as safer, a factor of particular importance for families. Although service differentiation is the key to standing out in the competitive hospitality market, location greatly improves the value of these differentiated services. “Location and accessibility (…) help customers find the hotel easily, provide a good view of the surroundings and save time for customers seeking to visit nearby places of interest” (Xu and Li, 2016, p. 61;, cf. also Sim et al., 2006). Accessibility is, therefore, a crucial factor in a guest's decision-making process, particularly when choosing a hotel for short or family trips (Poon and Huang, 2017). The proximity to major attractions or hot spots not only allows guests to explore the surrounding area, but, when combined with differentiated services, also provides a comprehensive and convenient travel experience.
Moreover, standardisation precisely refers to the consistent quality and services provided by hotels, e.g. in-room services, airport shuttle services and free parking. Traditional hotels generally operate within established frameworks to deliver uniform service quality. In contrast, peer-to-peer (P2P) accommodation platforms such as Airbnb can provide distinctive and tailored guest experiences, yet frequently lack the standardisation and oversight characteristic of the conventional hospitality sector. This potential absence of conventions and controls may engender risks for hosts. The personalised experiences offered by P2P accommodation platforms contrast with the regulated standards of traditional hotels. Whereas Airbnb listings provide unique, tailored stays for guests, they lack the regulations and standardisation that hotels reliably offer. Hotels adhere to safety standards and regulations, ensuring guests have a consistent, risk-averse experience. In sum, while P2P platforms prioritise uniqueness, hotels focus on consistent standards which provide guests with security and peace of mind.
Hence, standardised facilities and services offer a sense of familiarity and assurance to guests, facilitating their exploration of the surroundings. For example, knowing that a hotel provides a reliable shuttle service to key points of interest can encourage guests to explore the local area. Similarly, a hotel that offers comprehensive business services, for example, meeting rooms or conference facilities, can be particularly valuable for business travellers. If this hotel is also located in the city centre or near major business districts, it can provide added convenience for these guests, thus improving the overall value proposition. In contrast, if it is also located near a beach or a natural park, the overall relaxation experience for the guests can be significantly enhanced due to the serene surroundings. Therefore, the value of these differentiated services can be significantly improved when combined with a strategic location.
Secondly, hotels design their services to create unique value propositions for their guests. In addition, providing a local editorial perspective, local insider tips and practical information on neighbourhoods can facilitate an authentic local experience for guests. Furthermore, guests place a high value on property characteristics –e.g. open spaces, increased safety, car parking or gym services- and a variety of amenities and services –e.g. wellness facilities, dining options, business services amenities, Internet access, minibars, TV streaming services, hair dryers or coffee makers (Radojevic et al., 2015). Hotels that offer car parking can also be perceived as more secure and provide additional convenience for guests.
Thirdly, hotels differentiate their services to ensure survival (Benítez-Aurioles, 2019). As Sánchez-Franco and Aramendia-Muneta (2023) suggest, hotels cultivate a traditional delivery-focused paradigm that stresses service quality and facilities (Chu and Choi, 2000; Dann et al., 2019; Kandampully and Suhartanto, 2000). Hotels offer housekeeping services, guest loyalty programmes (related to money saving) and facilities to make them less vulnerable to competition (see also Festila and Müller, 2017; Young et al., 2017). Furthermore, hotels prioritise the quality of interactions between guests and employees, which is crucial for guest satisfaction (Osman et al., 2019; Parasuraman et al., 1988). As Xu and Li (2016, p. 63) conclude, “staff performance seemed to be among the most influential factors in determining customer satisfaction […] that can strengthen the customer's relationship with the hotels”. The staff is easily accessible and encourages engaging guest interaction. In addition, hotels create opportunities for social and recreational activities or events that encourage guests to engage with each other and build a sense of community. On the one hand, quality interactions between guests and employees can significantly improve guest satisfaction. On the other hand, hotels create a more pleasant and satisfying experience for their guests, leading to greater customer loyalty. In sum, a hotel employee who goes above and beyond to assist a guest, such as providing personalised recommendations for local attractions or promptly addressing any issues, can create a positive impression on the guest and lead to increased satisfaction and a higher likelihood of the guest returning or recommending the hotel to others.
Accordingly, our research proposes precisely a service-orientated method to explore the latent qualities extracted from the experience narrated by the tourist (e.g. location of the establishment, service quality and perceived value, staff, sleep and comfort, amenities and related services, cleanliness, hotel atmosphere, among others). The emergence of UGC precisely reshapes how customers share their experiences and make decisions, creating an untapped source of data that offers nuanced insights into guest preferences and expectations. Following Sánchez-Franco et al. (2019), “UGC plays an increasingly important role in consumer attitudes and purchase intentions, particularly in relation to travel services (Litvin et al., 2008; Liu and Park, 2015; Marchiori and Cantoni, 2015; Wu et al., 2017)”. Our study, in this sense, emphasises that the spontaneous and multifaceted nature of UGC can supplement traditional survey-based research, revealing hidden dimensions of the guest experience that could have remained uncovered. Furthermore, our research assesses the fulfilment of expectations, predictions, goals and desires in the context of the relationship. In this regard, UGC tends to be more empathetic than other one-dimensional metrics. UGC highlights the utilitarian, affective, social and symbolic aspects of consumer experiences in their natural setting, without interference from researchers (cf. Sánchez-Franco et al., 2016). In this context, the media systems dependency theory suggests that consumers who rely heavily on a particular medium (e.g. community-based online services) are more susceptible to attitudinal and behavioural changes stemming from that community (cf. Ball-Rokeach, 1985). Therefore, this analysis can provide a more precise, dynamic and detailed understanding of the determinants of guest satisfaction, allowing hotels to refine and calibrate their services and formulate value propositions that precisely meet guest needs.
As Zhu et al. (2020) conclude, reviews are multifaceted and incorporate richer content that a single scalar value cannot fully capture (Archak et al., 2011). Structured questionnaires sometimes introduce various response biases resulting from the question's wording. A J-shaped distribution characterises the scores, which tend to be positive (Zervas et al., 2021), perhaps motivated by fear of retaliation (Dolnicar, 2018). Additionally, traditional questionnaire-based research requires an arduous effort for data acquisition. The questionnaires are not regularly updated compared to the dynamism of the tourism sector and require excessive input from respondents. In contrast, data from information mediation platforms are accessible to the researcher. Free and natural opinions are labelled thematically, spatially and temporally. They tend to contain less bias due to influence. And they ultimately offer a vital and abundant opportunity for scientific studies on tourism once the researcher removes the noise they have. In sum, UGC presents a way to spread information dissemination and inform travel decision-making. By sharing their travel experiences through content, images and videos, customers enhance the amount of data available data for future potential travellers, encompassing new markets, topics and sensitive matters. The up-to-date and easily accessible customer feedback provided through UGC serves as a modern form of digital word-of-mouth (eWOM) (Mitsopoulou et al., 2023). Xu et al. (2023) demonstrate the indirect influence of UGC on travellers' intentions to revisit a destination, as well as on word-of-mouth (WOM) transmission through perceived image and satisfaction experienced with that destination. Furthermore, empirical findings confirm the importance of considering the UGC as a key contemporary source for destination image formation. To sum up, the influence of UGC on consumers' attitudes towards a brand and their purchase intention has been widely recognised in the recent literature (Chevalier and Mayzlin, 2006; Godes and Mayzlin, 2004; Martins Gonçalves et al., 2018).
By applying data mining and machine learning techniques, our study cleans and extracts the essential elements of the original text (topics and thematic communities) and generates a simplified and understandable version of the text concerning the guest profile and influencing the enquiry, booking, purchase and repurchase of tourism services (cf. Litvin et al., 2008; Veloso and Gomez-Suarez, 2023; Vermeulen and Seegers, 2009). Furthermore, as Sánchez-Franco et al. (2022) point out, user-created online reviews play a crucial role in building hotels' reputation (through eWOM, cf. Chong et al., 2018; Hennig-Thurau et al., 2004; Jalilvand and Samiei, 2012). Analysing natural and unstructured narratives attracts users and keeps them loyal (e.g. Gretzel and Yoo, 2008; Park et al., 2007; Sánchez-Franco et al., 2018; Ye et al., 2011). In conclusion, adopting a customer-focused, data-driven paradigm can markedly augment a hotel's competitive advantage and financial performance. As society advances further into the digital era, leveraging the utility of UGC and sophisticated analytics will become increasingly instrumental for the prosperity of hotels.
3. Research objectives
Our study aims to employ data mining techniques to quantitatively assess the influence and relevance of key terms and semantic structures in hotel evaluations. This approach allows us to uncover potentially hidden, yet impactful, aspects of guest feedback, a significant step towards enhancing the guest experience and hotel offerings. As a prerequisite to elucidating our methodology, our research outlines the following structured research objectives:
Data Preprocessing: Our aim involves the preprocessing of data, intending to sanitise and streamline the structure of our review corpus. This process provides a clean dataset for further exploration and analysis, allowing for more accurate interpretations.
Key-Term Extraction: A sub-aim lies in the retrieval, filtering and extraction of critical terms that characterise each narrative or published opinion. This extraction is carried out on both the frequency of occurrence of a term and the amount of information it represents, allowing an in-depth understanding of prevalent themes.
Handling Extensive Document Archives: Our study uses pattern discovery and visualisation techniques based on machine learning methods, thereby reducing the complexity of the large data set. Our study also proposes to manage the extensive archive of document reviews through the automatic revelation of latent topics.
Contribution of Semantic Structures: Our primary sub-aim here is to apply data mining techniques to evaluate the contribution and significance of semantic structures in hotel appraisals, providing a more nuanced understanding of guest feedback.
Identifying Relevant Topics: The secondary sub-aim involves identifying the essential topics (by NMF topic modelling) associated with guests' experiences.
Topic Importance: Our tertiary sub-aim centres on the identification of the importance of each topic. This process is achieved by applying XGBoost, using SHAP values.
Semantic Grouping: The present study seeks to facilitate and accelerate the interpretation of semantic structures by clustering topics using the K-Prototypes algorithm. This results in a more intuitive understanding of the topics, allowing faster insight and more informed decision-making.
Proposing an Automated Review Evaluation System: Our final objective is to propose the development of an automatic review evaluation system. This system would predict the quality of reciprocally beneficial relationships with hotel establishments, which would reduce the time spent searching and sorting through the available documentation. This proposition could lead to significant improvements in how hotels manage and respond to guest feedback, enabling them to enhance the guest experience more efficiently.
4. Research method
4.1 Data collection
Andalusia is selected as a geographical, cultural, social, economic and political area of analysis because of its prestigious diversity in the tourism sector analysed, that is, the hotel sector. Andalusia offers a catalogue of hotel establishments based on sun and beach, business and congress and cultural, urban and rural areas. In particular, our research analyses structured and essentially unstructured data published on online content infomediation platforms (in our case, Booking.com) and focuses on the cities of Seville, Cordoba and Granada, three cities with significant points of cultural interest (museums, monuments and other assets of cultural interest). Seville, Cordoba and Granada account for 33% of tourists visiting the Andalusian region (Hotel Occupancy Survey, INE, September 2022).
4.2 Retrieving and extracting information from central recommender systems
An automated tracking system is run for data collection to record data related to the hotel, the review and the guest (reviewer) in a specific location indicated. The procedure is summarised in the following steps:
The proposed methodology employs a Python-based web scraping approach to systematically collect and structure hotel review data across multiple locations from Booking.com. Initially, the list of study locations is defined (Step 1). For each location, the programme automatically extracts hotel names exceeding three stars from Booking.com using request queries (Step 2). Subsequently, the software iterates through each hotel on Booking.com, gathering embedded structured data such as rating and date, along with unstructured review titles and texts via additional queries (Step 3). Reviews are stored as dictionaries containing the location, hotel name and other variables. The dictionaries are then used to construct Pandas data frames for analysis. The entire scrape-structure-store process is repeated for each location (Step 4), enabling the automated aggregation of multisite structured and unstructured Booking.com hotel review data systematically. It is stressed that no individualised analyses are performed and the data collection is for purely academic purposes.
The proposed approach thus provides an efficient and scalable means of compiling large corpora of textual data for text analytics and modelling. The methodology is generalisable across review domains and websites. Retrieval and extraction aim to (1) retrieve and extract structured data associated with user reviews from hotel establishments, (2) retrieve and extract the set of features of reviews written in natural language to obtain a new set of nonredundant features and (3) produce structured data patterns that make data analysis feasible. The metadata are city and hotel, type (group or friends, solo, couple or traveller with children, family) and length of stay, among others.
Once the data are downloaded between 1 and 3 September 2022, our research individualises the reviews into positive and negative
reviews (see Figure 1) and filters the English narratives with at least 80 characters, avoiding excessively concise reviews. The final number equals 23,545 reviews (14,317 positive and 9,228 negative) once normalised and cleaned (see Section 3.3. Data cleansing) between September 2019 and August 2022.
Finally, our study assesses the quality of the online review. Several published studies have explored the influence of review quality on guest evaluations from different aspects, e.g. review length, review structure, readability and writing style. In particular, Forman et al. (2008) reveal that the readability of the review has a positive effect on its usefulness and spelling errors have a negative impact. The Flesch Reading Ease metric, calculated using the textstat 0.7.3 Python package, assigns readability scores on a scale from 1 to 100, with higher values denoting greater legibility. Scores ranging between 70 and 80 correspond to an eighth-grade reading level, indicating that such texts should be reasonably comprehensible for the average adult reader. The negative reviews published on Booking.com and analysed in our study, reach a Flesch Reading Ease index equal to 75.22 (fairly easy to read). On the contrary, the value of positive reviews drops to 70.26 (fairly easy to read). Moreover, there are no appreciable differences depending on the type of traveller (values around 72 points). There are also no significant differences according to the traveller's origin (values around 70–73 points) or the length of stay (values around 72 points).
4.3 Data cleansing
Since the representation of the reviews may correspond to a high-dimensional space, methods must be applied to clean and structure the input text and identify a simplified subset of the corpus features that can represent it in subsequent analysis. For this purpose, a normalisation process (cf. Cotelo et al., 2015) based on the automatic transformation of the documents is carried out to eliminate errors and expressions typical of the jargon used in the field of social networks (e.g. abbreviations, words with repeated letters or errors, textual emoticons, ASCII art, stop words, among others). In addition, the conversion of different forms to a lower number (lemmatisation) is carried out, among other normalisation tasks.
5. Exploratory data analysis: results
Our research applies specific algorithms to extract underlying patterns from the data, thereby gaining knowledge and understanding of the described phenomenon (cf. Rygielski et al., 2002). This section focuses on applying algorithms for the identification and differentiation of the various semantic structures (posted by hotel guests) analysed from the processing of the content posted in the hotels and, consequently, their contribution to the levels of relational quality detected through sentiment analysis. This phase is subdivided into three subtasks:
Subtask 1 focuses on visualising the most characteristic words in a category compared to others (using Scattertext 0.1.9, Kessler, 2017). It extracts a scored list of the most prominent sentences from a review by applying the PyTextRank 3.2.4 package–a modified version from Mihalcea and Tarau (2004) – and Phrasemachine (Scattertext 0.1.9) to identify noun phrases.
Subtask 2 visualises pairwise comparisons between texts using word shift graphs, that is, a method for identifying which words contribute to the difference between the texts being compared (Shifterator 0.3.0, Gallagher et al., 2021).
Subtask 3 focuses on modelling topics and their contribution to class prediction (relatively positive or relatively negative review).
5.1 Subtask 1: visualisation of the most characteristic words of a category in comparison to other categories
Initially, our research creates a scatter text graph that shows which words are associated with relatively positive (SAT) versus relatively negative categories (DISSAT) when guests describe their hotel stays. For this purpose, our study uses the Scattertext package (Kessler, 2017). Scattertext is a Python package used for generating interactive visualisations of text data, particularly for analysing and comparing the usage of words across different categories of text. Scattertext helps perform sentiment analysis, topic modelling and text classification tasks. Researchers can use it in different types of text data, e.g. customer reviews, news articles and social media posts.
In particular, Scattertext allows identifying words and phrases that are disproportionately frequent in one text category while also providing a way to compare the overall usage of words across different categories. Scattertext here identifies the most characteristic words in two texts based on the frequency with which each word appears in one text compared to the other, having eliminated adjectives and adverbs in our case. Figure 2 shows the visualisation of word usage between positive comments (SATISF) and negative comments (DISSAT) written by guests. Our study establishes the RankDifference()to determine the word scores in creating the scatterplot. Illustratively, the word “sight”, located at the top left of Figure 2, has a score of 0.73127 with 373 mentions in SATISF (y-axis) and 18 in DISSAT (x-axis). Its graphical coordinates are (6, 103); 6 (for 25,000 terms) is the DISSAT coordinate, and 103 is the SATISF coordinate. Also, it shows 26 per 1,000 documents (SAT frequency) and 2 per 1,000 documents (DISSAT frequency).
The words on the x- or y-axes show high precision, i.e. high discriminative power regardless of their frequency. The closer a point (word) is to the top of Figure 2, the more frequently the word is used in positive reviews (SATISF). The further a point (word) is to the right, the more frequently the word is used in negative reviews (DISSAT). Very common words in both text types are shown in the upper right-hand corner and rare words (rarely used in both review types) are shown in the lower left-hand corner. In this sense, what is relevant is in the upper left and lower right corners. Namely:
In the upper left corner (Figure 2), words such as sight, heart, attraction, cordoba [Córdoba], distance or tapa, among others, are used frequently in positive reviews and rarely in negative reviews.
Words frequently used in negative reviews and words infrequently used in positive reviews occupy the bottom right corner of Figure 2. These keywords include hear, smell, toilet, lack, corridor, wall, or phone.
The most characteristic (common) terms of both sets of documents (stop words) tend to appear in the upper right corner (Figure 2). In this sense, the words commonly used in both types and with little differentiation are bed, breakfast, staff, pool, park, or bathroom, among others.
In summary, Figure 2 performs a thorough lexical analysis, distinguishing words most frequently featured in positive and negative hotel reviews. Terms such as sight, heart, attraction and distance emerge as salient features in positive reviews, emphasising the importance of the location of the hotel and the tourist attractions (cultural and gastronomic) of the city in the satisfaction of the guest. On the contrary, terms such as hear, smell, toilet and lack are linked to the hotel's primary service and comfort, highlighting noise, odours and sleeping comfort problems, i.e. comfort-related issues such as noise pollution and cleanliness. While tourist attractions may serve as potent marketing instruments, a lapse in essential comforts can substantially detract from a guest's overall experience.
Furthermore, proportional changes are easy to interpret, but simplistic in extracting exciting differences between two texts (Gallagher et al., 2021). Scattertext allows the use of the singular value decomposition (SVD) technique with three factors, and our analysis proposes the relative positions of key terms after removing adjectives and adverbs. In Figure 3, our study represents the first two singular values (the result of SVD decomposition), locating each term on the x-axis (first singular value) and the y-axis (second singular value). SVD partly confirms the above results, which show words associated with the core services of a hotel, namely, the room and its services related to sleep and the bathroom, and representative of negative reviews (DISSAT). In contrast, terms about attractions and their distance from the hotel, views and amenities of the recommended areas of the destination (e.g. restaurants) are associated with positive reviews (SATISF).
In this regard, Figure 3 elevates the discussion through the SVD technique, validating and refining the insights gained from Figure 2. It becomes evident that shortcomings in core hotel services, for example, the room itself and its ancillary features, predominantly shape negative reviews. However, terms associated with location, scenic views and nearby attractions play a positive role. For management, our results require a bifurcated strategy: (a) enhancing basic comforts for a satisfactory stay and (b) promoting the hotel's locational advantages of the hotel as unique selling propositions.
Furthermore, our study extracts specific phrases for each category using Phrasemachine (Scattertext 0.1.9), which allows a better contextualisation of the distinctive cues between classes. For example, in Figure 4, in the case of positive reviews, the expressions “excellent location’ (0.91486), ‘friendly staff’ (0.86630), a spacious room (0.84879) or ‘perfect location’ (0.84710) achieve the highest scores. On the other hand, the distinctive expressions of the negative reviews are the following: didn't [did not] work (−0.85441), room door (−0.82114), room window (−0.81220), noisy night (−0.77065) or double bed (−0.75652).
Therefore, Figure 4 uses Phrasemachine to delve into specific phrasal patterns indicative of guest sentiment. Phrases related to an excellent location and friendly staff emerge as leading indicators of positive guest experiences. In contrast, “didn't work” or “noisy night” are revealing of negative experiences. These findings imply that micro-interactions and amenities are not mere experiential details; they wield the capability to delineate the entire guest experience and, then, require judicious management.
The consolidation of findings from Figures 2–4 explains that both external and internal elements critically contribute to the architecture of guest reviews. While aspects such as scenic views and local attractions can be instrumental as marketing levers, basic characteristics relating to guest comfort are critical. Hospitality management would be well advised to formulate a balanced strategy that not only capitalises on the unique selling points concerning location but also addresses the fundamental mechanics of guest comfort and service.
5.2 Subtask 2: pairwise comparisons between texts using word shifts graphs
Using Shifterator 0.3.0 (Gallagher et al., 2021), the estimated benchmark scores distinguish between different regimes of interest in word scores. In particular, the Shifterator makes it possible to identify which words explain the most variation between texts (reference and comparison categories) and visualise pairwise comparisons using word shifts. Furthermore, it allows us to know the score of each word in terms of its use in each text and, likewise, to know qualitatively whether the word is relatively positive or negative. In summary, our study quantifies which words contribute to the differences between two texts and how they do so. By making these lexical shifts transparent and quantifiable, word shift graphs enable more grounded statistical analyses and enhance our understanding of how language varies across textual contexts.
In particular, our study constructs several word shift graphs with horizontal bar charts that provide word-level explanations of how and why two texts in each category differ. Our study previously constructed a dictionary of words and each word is assigned a weight or score using weighted logarithmic odds values (above the 60th percentile), identifying 7,216 keywords with their scores from most dissatisfied (negative values) to most satisfied (positive values). It also avoids overlapping terms between polarities (positive and negative) according to their context of use. The weighted log-odds method is described in Monroe et al. (2008). It is a relevant approach for text analysis in that it accurately measures how word usage differs (and scores) in a comparative set of documents, in our case, “relatively positive/satisfactory” or “relatively negative/unsatisfactory” reviews.
A brief interpretative guide to the word shift graphs (Gallagher et al., 2021) showing the top fifty words contributing to the difference in satisfaction versus dissatisfaction between the categories compared is set out below (Gallagher et al., 2021):
A relatively positive word (+) is used more frequently (↑) in the second text (COMP) (less in the first text, REF).
A relatively positive word (+) is used less frequently (↓) in the second text (more in the first text).
A relatively negative word (−) is used more frequently (↑) in the second text (less in the first text).
A relatively negative word (−) is used less frequently (↓) in the second text (more in the first text).
If the contribution of a word is positive, δτ > 0 (that is, + or − ↓), the bar points to the right, and if negative, δτ < 0 (i.e. + or − ↑), the bar points to the left.
In summary, four different types of contribution (+−↑↓) are indicated by bars. A relatively positive and more frequent (compared to) word is characterised by a bright yellow bar on the right (+↑), while a relatively negative and more frequent (compared to) word is characterised by a bright blue bar on the left (−↑). For our word shift graphs, our study sets a reference value of 0 (the centre of our dictionary scale) and applies a stop lens to dictionary words between −5 and 5. The graphs feature diagnostic plots of cumulative contribution and text size in the lower left and right corners, respectively. Specifically, the point at which the cumulative curve intersects the horizontal line signifies the proportion of the word shift difference accounted for by the most contributing terms (see Gallagher et al., 2021). Therefore, it is essential to consult it to determine the weight to be given to the interpretation based on the word-shift graph. The second diagram shows the relative size of the text in each corpus, measured by the number of tokens (here, words) used.
5.2.1 Analysis by type of traveller
In the following, our study discusses the main results achieved by the type of traveller (Figure 5). The couple category is used as a reference category (REF) due to its highest number of reviews and valences are based on weighted logarithmic odds values or the strength of the link between words and the valence of reviews.
The analysis of guest reviews reveals that those travelling as a family tend to have more negative experiences than those travelling as a couple due to the higher use of relatively negatively loaded words in the narratives of reviews (from families), such as room, floor, time, water, pay, or smell. In contrast, the couple category employs more positive words, for example, location, walk, view, terrace, or city. On the other hand, guests travelling as a family tend to use relatively positive words that partially offset the negativity noted earlier; e.g. staff, breakfast, or shop -associated with the hotel's quality of service and amenities.
The relative total of each type of contribution (positive or negative) is shown at the top of the word shifts graph, which allows for a clear comparison between the different sentiments expressed by guests travelling as a family and as a couple.
The analysis of guest reviews reveals that those travelling in groups have more favourable experiences than guests travelling as a couple due to the increased use of positive words, such as staff, location, amaze, or rooftop, associated with the quality of service provided. In addition, guests travelling in groups tend to use fewer negative words (e.g. bite, room, night, hear, or window) and more negative words (e.g. water, book, air-conditioning, pay, or tell) related to room amenities or service issues.
Travelling alone has more negative experiences than travelling as a couple. This difference in sentiment is likely due to the greater use of relatively negatively loaded words in review narratives, e.g. room, hear, noise, people, or window. Likewise, the lower use of positive words, such as location, breakfast, staff, view, or city, is also worth noting. It could suggest that solo travellers highlight negative problems with the comfort of their accommodation and nearby amenities.
Finally, looking at the point where the cumulative curve intersects the horizontal cut-off line (see cumulative contribution diagram in the bottom left corner), the first ten words explain around 50% of the difference between the two texts.
5.2.2 Analysis by length of stay
Our study also discusses the main results achieved by the type of traveller (Figure 6). The reference category (REF) is a guest whose stay is equal to one night.
Guests staying two nights or more tend to use more negative words, such as water, floor, window, or door, suggesting complaints about the room's amenities or service. One possible explanation may be that guests who stay longer tend to be more critical of their hotel stay, foster higher expectations, and, as a result, likely question issues related to the amenities and services the hotel provides associated with water, floor, window or door.
Hotels should, therefore, strive to balance addressing negative issues and emphasising positive aspects to ensure that guests staying for longer periods have a pleasant stay, providing excellent service, being attentive to guests' needs and offering a variety of amenities. Additionally, hotels should consider that guests staying longer may have higher expectations and adjust their services accordingly.
The relative total of each type of contribution is shown at the top of the word shift graph, allowing a clear comparison between the different sentiments expressed by guests staying for different periods. In general, hotels should strive to balance addressing negative issues and highlighting positive aspects to ensure that guests staying longer periods have a pleasant stay. Finally, looking at the point where the cumulative curve intersects the horizontal cut-off line, the first ten words explain around 50% of the difference between the texts.
According to previous results, our methodology allows for the quantification of the cumulative contribution of words, offering a valuable parameter for interpretation. It is useful in customer experience management, allowing the development of targeted hospitality strategies and specialised in-stay experiences. Additionally, it helps to allocate focus and resources to areas that need improvement, as identified by significant terms in negative reviews.
In particular, sentiment allocation by traveller is a notable feature of our method. Figure 5 provides additional information on the experiences of varying types of travellers and lengths of stay. Therefore, our method serves as a diagnostic tool that highlights problem areas; also suggests targeted solutions for hotel management, enriching the base for tactical and strategic decision making. In fact, our observed trends in guest reviews in different travel categories (families, couples, groups and solo travellers) reveal intricate dynamics of expectations, experiences and expressed sentiments. Below are key interpretations for each type (COMP) compared to couples (REF):
Families: The greater prevalence of negatively loaded words in family reviews might suggest that families are more critical or have higher expectations regarding room quality and stay (e.g. such as room, floor, time, water, pay, or smell, among others). Dissatisfaction could be rooted in the challenges associated with accommodating multiple people with varying needs, which could lead to increased focus on shortcomings. However, the use of positive words related to service quality (e.g. staff, breakfast, or shop, among others) partially offsets this negativity, indicating that family-friendly services could mitigate some of the perceived shortcomings.
Groups: Interestingly, the groups display favourable experiences. It suggests a social amplification of positive sentiment. Groups focus less on negative issues and find social interaction to compensate for any service-related or amenity-based shortcomings. The groups seem to have general criticism related to room amenities or specific service issues, showing aligned expectations as a collective.
Solo travellers: Negative experiences among solo travellers could be interpreted in various ways. Focussing on noise, windows, or floor, among others, could suggest an emphasis on privacy and personal space, which could be lacking. Solo travellers may feel less distracted by social interactions, leading to greater awareness of any shortcomings in their accommodation.
In conclusion, our research underscores the need for specific segmented customer experience management strategies. They offer valuable quantitative and qualitative insights that can greatly inform hotel management decisions. By adapting services and preemptively addressing customer needs based on these insights, significant improvements in satisfaction scores and fiscal viability can be expected.
5.3 Subtask 3: modelling of topics
Visualisation-based analyses provide a meaningful and interpretable summary of how individual terms contribute to cross-text variation and are helpful in knowledge extraction. However, identifying the rational and experiential (latent) topics in the corpus, and their visualisations, is also a fundamental approach to understanding the proper context of guests' opinions.
In this regard, topic modelling is a valuable tool in natural language processing and text mining, which groups similar words to identify patterns in a collection of text documents and latent topics in the corpus, even when they are not explicitly mentioned. In this regard, the generated topics are interpretable, and the terms associated with each topic highlight the meaning of each. Therefore, the results could be used for text classification, document summation, or building recommendation systems.
Next, our research estimates the relationships between terms and documents through a text-mining algorithm to discover hidden semantic structures (topics) in our dataset.
In particular, our research analyses natural and unstructured narratives using machine learning algorithms based on text summarisation and the application of NMF (cf. Lee and Seung, 1999). Our study applies topic analysis to normalised reviews using the NMF method implemented in sklearn (scikit-learn developers, 2020). NMF factors high-dimensional vectors (in our case, the TF-IDF matrix of M documents and N terms or words) into a lower-dimensional representation. The lower-dimensional vectors are non-negative, and their coefficients are also non-negative. In essence, from a matrix of documents (revisions) by words (A), the NMF application generates two matrices, i.e. (1) the matrix W (topics × terms) and (2) the coefficient matrix H (documents x topics). Our analysis excludes terms that appear in less than 100 documents (min_df) or more than 95% (max_df) of the documents. NMF shows higher coherence levels than Latent Dirichlet allocation (LDA). Coherence levels measure the similarity of meaning between the critical terms in topic i. Coherence helps to differentiate between topics that are interpretable and topics that are merely artefacts resulting from the applied statistical technique. Using the coherence score c_v (more accurate than u_mass), our study runs the model for a different number of topics (from 10 to 100 topics) and selects the number of topics with the highest coherence score. Following this procedure, the recommended number of topics is equal to 25 – a number from which coherence starts to decrease. Table 1 provides a word-by-word description of each topic and an illustrative example of a narrative for each topic.
Moreover, selecting a relatively small set of high-probability words per topic is advisable, since reviews tend to focus on key aspects of a particular stay. Rather than extracting extensive vocabulary, limiting to approximately 10 words per theme retains interpretability whilst minimising extraneous content. Although dependent on the dataset, this constrained lexicon likely encapsulates the essence of each topic without redundancy. In Table 1, topics and associated terms should be manually examined to validate consistency and relevance.
Similarly, our study applies an XGBoost classification algorithm, trained with the H-matrix of documents per topic and previously transformed by the natural logarithm of the values. Eighty per cent of the documents allow us to train the model. The remaining 20% will enable us to validate the model's performance and ensure that the model reliably predicts future observations. Our analysis uses StratifiedKFold and GridSearchCV from scikit-learn to select the best parameters for the XGBoost function. StratifiedKFold is a variation of KFold that returns stratified folds which preserve the percentage of samples for each class, with five-folds. GridSearchCV allows testing a range of parameters. Once both functions are applied, the best hyperparameters are: {‘colsample_bytree’: 0.4, ‘gamma’: 0.5, ‘max_depth’: 8, ‘min_child_weight’: 5, ‘subsample’: 0.8}.
Finally, our analysis further estimates the confusion matrix (Table 2) and the Matthews correlation coefficient (MCC) to validate the research model. The MCC is a statistical measure of the quality of binary (two-class) classifications. It takes into account true and false positives and negatives and is generally regarded as a balanced measure which can be used even if the classes are of very different sizes. In our analysis, the MCC value equals 0.70.
Second, our study identifies key characteristics related to hotel stays using the SHAP values for each topic for each document (SHapley Additive exPlanations, hereafter SHAP; see Lundberg and Lee, 2017). The SHAP values describe how each topic in our model contributes to increasing (or decreasing) positive or negative levels. Here, SHapley Additive exPlanations (SHAP) systematically uncover the crucial elements that govern guest satisfaction during hotel stays. Consequently, to clarify the hierarchy of influential variables, Figure 7 has been designed to reveal the factors that contribute positively or negatively to the overall guest experience.
In particular, Figure 7b allows us to visualise the importance of characteristics and their impact on the prediction by plotting summary charts. That is.
The Y-axis indicates the feature names in importance order from top to bottom.
The X-axis represents the SHAP value, which indicates the degree of change in model outputs.
The colour of each point on the graph represents the value of the corresponding feature, with red indicating high values and blue indicating low values.
Each point represents a row of data from the original data set.
For example, Topic 0 emerges as the most vital, including a range of factors including the quality of staff interactions and aesthetic appeal of the facilities. Topic 0 increases the predicted output, that is, guest satisfaction. On the contrary, variables such as Topic 6 and Topic 18, which deal with noise and comfort issues, as well as the effectiveness of problem resolution, are found to harm guest satisfaction.
In this regard, the topics with the highest (to lowest) degree of importance are the following:
Topic 0 (staff and facility aesthetics) (+) is related to elements of a hotel experience, including staff, language, furnishings, decoration, amenities, temporary stays, membership, ownership, location and design.
Topic 6 (noise and comfort concerns) (−) highlights the various equipment, services and characteristics provided in a hotel room to make the stay more comfortable and convenient for guests.
Topic 18 (guest concerns and resolutions) (−) is related to problems the guest may experience during their stay, e.g. maintenance work being done at the hotel.
Topic 1 (walking distance to attractions) (+) focuses on walking and exploring a specific location, such as an attraction or sightseeing spot, as well as nearby places such as plazas or shops.
Topic 7 (centrally located to explore city) (+) concentrates on exploring a city and its central area, such as the city centre or heart and how to reach it by walking or taking, for example, a taxi.
Topic 17 (charming old town and location) (+) focuses on the city and its characteristics, accessibility and visits.
Topic 24 (aesthetically pleasing design) (+) is related to the design and ambience of a hotel, precisely the outdoor spaces and the property's aesthetic appeal.
Topic 12 (overall guest experience and attributes) (+) highlights luxury and exclusivity and the experiences while staying in it.
Finally, to better understand guests in terms of topics and structural features, our analysis applies the K-Prototype approach to classifying similar guests into the same group. The variables used in the clustering for mixed data (numerical and categorical) are those shown in the table accompanying Table 3. In addition to the length of stay, type of traveller, or type of review, our study also includes the eight topics with the highest contribution to prediction (see Figure 7a); the scores are normalised. Our analysis also balances and intersects the different classes of the categorical variables to avoid over-dimensioning the classes.
Eight clusters are proposed using the elbow method. The elbow method calculates the total variance of the clusters (cost) for a number from 2 to n. As the number of groups increases, the total variance of the groups should decrease. The elbow method proposes that the number of groups in which additional groups do not produce a significant decrease in total variance is the number of groups to extract (here, 8 groups or clusters).
The UMAP (Uniform Manifold Approximation and Projection) dimensionality reduction technique is used to represent the data in 2 dimensions (Figure 8). Three steps are taken to obtain the embeddings: (1) the Yeo-Johnson transformation is applied for numerical variables and one hot encode for categorical variables; (2) UMAP is applied separately to each type of variable and (3) the obtained embeddings are combined. In particular, Figure 8 visually shows the quality of the clusters or segments. The spatial distance between the data points serves as an initial indicator of similarity or dissimilarity in the original high-dimensional feature space. Clustering phenomena within the map often point towards inherent groupings or classes present in the data, while point density offers insights into the prevalence of certain characteristics–not likely to be a spurious group. The topological aspects of UMAP maps thus provide additional layers of data interpretation, particularly in understanding gradual transitions between data clusters or groups.
In our research, although K-Prototypes clustering provides distinctive clusters, the clusters or segments are distributed with clear boundaries and specific clusters appear in different areas of the scatterplot. This is probably a consequence of suboptimal embeddings.
In summary, the analytical techniques adopted in our study provide a comprehensive yet nuanced understanding of the guest experience within hotels. SHAP identifies the pivotal factors that contribute to both positive and negative aspects of customer experience, thus guiding managers in crafting more targeted service strategies. Simultaneously, UMAP and K-Prototype facilitate the creation and visualisation of guest clusters, enabling service customisation at a deeper level. Our collective insights are indispensable for hotel managers seeking excellence in service, ultimately contributing to increased profitability.
In addition, our analysis calculates the mean value of each topic per cluster to check whether the mean allows one to evaluate the topics' significance in each cluster. Then the variance of the means between the clusters is calculated for each topic. This allows for the selection of the main topics per cluster. Figure 9 shows the differences per group. Values are scaled between 0 and 1 for ease of visualisation. The extracted clusters provide information on the various aspects of hotel stays that are most important to guests and can help hotel management improve guest satisfaction. That is.
The first group comprises guests travelling alone and hotels staying between 2 and 3 nights. The critical topic associated with this group is topic 6, which is related to the various equipment, services and characteristics provided in a hotel room to make the stay more comfortable and convenient for guests. Specifically, this group expresses concern about sleep comfort and noise during the night.
The second group consists of guests travelling as a couple and staying between 2 and 3 nights. The narratives in this cluster tend to be positive, with the most relevant topic being topic 1, which relates to the location and surroundings of the hotel, specifically the hotel's proximity to various tourist attractions, places of interest and shopping areas. To a lesser extent, topic 7 -related to the location and accessibility of the hotel in a city or urban area-is also relevant. Additionally, topic 0 -on the hotel facilities and services–is also discussed.
To sum up, the second group comprises the various features of hotel amenities, services and characteristics, including the hotel's proximity to points of interest, accessibility, ease of reaching the hotel by foot or public transportation, staff fluency in multiple languages, front desk service, decor and facilities, as well as the overall guest experience during their stay.
The third group of guest experiences comprises guests travelling in groups and staying for one night. The overall sentiment expressed is positive, and the most relevant topic discussed is topic 0 related to the hotel's administration. Topic 0 covers various aspects of the hotel, such as the staff, their level of fluency in different languages, the front desk, the decor, the facilities, the visit, the members, the property, the site and the decoration.
The fourth group is composed of guests travelling as a family and guests booking an overnight stay. The narratives in this group are primarily negative, describing issues related to topic 18. Topic 18 refers to problems that guests may experience during their stay, e.g. maintenance work at the hotel. In addition, topic 6 relates to the various equipment, services and features provided in rooms to make the stay more comfortable and convenient. Topic 6 is discussed to a lesser extent.
In particular, guest reviews indicate various issues related to the physical characteristics of the hotel room, such as the discomfort associated with the bed, lack of adequate air conditioning, outside noise or that of other guests, and issues with flooring, walls and windows. These factors can negatively impact the overall experience and sleep comfort. Although these physical characteristics of the hotel have previously been highlighted for stays of more than one night (group 1), in this case, they are also relevant for guests travelling as a family (with children).
The fifth group comprises solo guests who comment on positive experiences during their stay -for one night. The most relevant topic discussed is topic 7. Topic 7 comprises issues such as the hotel's proximity to the city centre, a location that allows exploring the heart of the city easily, the ease of reaching the hotel on foot or by public transportation, or the short distance to the hotel by taxi. In summary, the fifth group values the ease of reaching the city's main attractions and enjoying the city centre without transport problems.
The sixth group consists of guests travelling as a family for 2 or 3 nights. The most relevant topic discussed is topic 17 associated with the location, characteristics and accessibility of a hotel in a town; for instance, the hotel's proximity to the city centre, its ability to locate and visit charming sites, the hotel's architectural design, the courtyard, the charm of the visit, the central location, the ease of access and the proximity to any points of interest in the town, such as a bridge or a cathedral.
Therefore, the sixth group values exploring attractive city sites and having a comfortable stay with easy access to amenities and points of interest in the area.
The seventh group is identified as guests travelling as a family for one night and reporting mainly negative aspects. However, no specific topic stands out in their reviews.
The eighth group is made up of families staying overnight. They narrate positive aspects that revolve around topic 12, which relates to the luxury and exclusivity of a hotel and the guest's experience while staying in it. This topic encompasses various aspects, such as being amazed by the hotel's experience, the rooftop, related to an outdoor area on the top of a building, often used as a recreational space or for events, the design, the property, the staff, the construction, the stunningness, the suite and the visit. For example, a family orders suite rooms to reduce lodging costs. Also, topic 0 is discussed.
In summary, hotel managers must pay attention to the aesthetic and functional elements, the physical characteristics and maintenance of the establishment, the level of service provided by the personnel, the quality of the building and its infrastructure, the level of surprise and satisfaction generated, the unique rooms and amenities offered, and the overall guest experience. Therefore, this group of guests appears to value the luxury and exclusivity of the hotel, specifically the hotel design and rooftop experience, which left them amazed. They also praise the hotel staff and the hotel maintenance level. The hotel suite and the visit also stood out as positive aspects.
6. Conclusions
Our study underscores the paramount importance of considering a holistic and customer-centric approach to improve guest satisfaction in the hotel industry. Drawing on commitment theory, our research identifies relational quality, location and accessibility, service differentiation and standardisation as key facets that significantly impact guest satisfaction. This understanding offers valuable information for hotel managers to craft services that resonate with guest expectations and foster trust and commitment.
Our research presents a comprehensive method for analysing guest reviews using advanced data mining and machine learning techniques. It (1) aims to identify key characteristics and themes that guests highlight during their hotel stays, (2) visually explores the relationships between these characteristics and differences between different types of travellers through online hotel reviews and (3) determines predictive power. Its implications are crucial for the hospitality domain, as they provide real-time insights into guests' perceptions and business performance and are essential for making informed decisions and staying competitive. Furthermore, as stated in the Andalusian Regional Government Strategic Tourism Marketing Plan (2020), obtaining this information promptly is critical to the success of hotel establishments.
However, with the abundance of online reviews available, it can be overwhelming for managers to analyse them effectively. Our method thus helps to organise and distil this massive amount of data, making it easier to understand. Furthermore, using data mining, machine learning techniques and visual approaches, researchers and managers can extract valuable insights (on guests' preferences) and convert them into strategic thinking based on exploration and predictive analysis. In summary, by providing practical implications for guest perceptions, our study suggests that different types of guests present differences in hotel key factors. Consequently, it aims to help hotel managers in making informed decisions, thus improving the overall guest experience and increasing competitiveness.
7. Implications
Our work pursues an exploratory purpose (Rigdon et al., 2017). It designs computational experiments and analyses complex and uncertain systems. As a result, it helps (1) to gain and extend knowledge and understanding of the phenomenon described and (2) to better pinpoint the problem to be investigated, that is, the organisation of the information provided by the data, detection of patterns of behaviour and determination of topics (or semantic structures) and their relationships with the phenomenon in question. Therefore, the proposed research encourages further theory-building by applying an inductive reasoning perspective (Henseler, 2018).
Based on the designed method, our study develops a prototype proposal in the tourism domain that visualises the advantages of analysing UGC. In particular, topic modelling, prediction, classification and visualising natural language processing results are employed in our research to facilitate the preprocessing of guest online reviews and the subsequent analysis of data from various academic disciplines and research areas. Therefore, our key implication lies in proposing an approach (a) that can find and model latent knowledge that researchers find difficult to observe by exploring large volumes of data and (b) that helps in the decision-making process in the hotel sector. In this context, integrating multiple data sources (structured and unstructured) based on guest feedback and their subsequent compression and value provision recommends ways of doing that are not possible with traditional single-discipline approaches. Moreover, as Rong et al. (2012) point out, data mining emerges precisely as a valuable method to help business managers achieve the goals of efficient user relationship management.
In short, the field of information and communication technologies and tourism generates growing academic, technical and business opportunities and challenges when approached together. For example, the application of data mining to the tourism sector, particularly to hotel establishments, increases the chance of meeting demand with less information asymmetry. Furthermore, increased hospitality competitiveness creates satisfaction and loyalty-specific objectives to improve international positioning and provide stability to the destination (tourists who repeat and recommend their stay; see Campón-Cerro et al., 2015a, b; Hernández Mogollón et al., 2013). Therefore, the level of relational quality in their accommodation in Andalusia is a determining factor in creating, maintaining and intensifying the expected loyalty. Nevertheless, although published assessments and perceptions of quality and excellence are based on their experience (during their stay), it is necessary to go deeper into the printed text and analyse its latent structure from a demand perspective to ensure a satisfactory and emotionally rewarding visit.
Consequently, our research contributes to the literature by revealing the vital features of hospitality experiences and hotels perceived (by guests) and how to improve guest experiences. From the logic of sufficiency, this question is in-depth using a vast amount of natural and unstructured UGC to provide insights that may not be obtained through conventional methods. Therefore, our research proposes an integration framework for the content provided by guest comments concerning accommodation to extract which hospitality characteristics are equally or differentially relevant. Our study contributes to the literature on hospitality services, offering insight for practice and allowing the design of guest (dis)satisfaction policies and a more fine-grained understanding of hospitality services. Furthermore, the results reported in this investigation contribute to the debate about whether hotels and other alternative tourist accommodations compete concerning the main accommodation conditions.
The main implications of our study are thus as follows. First, hotel managers should improve the services (improving rooms and rest). In particular, the hotel should take preventive measures against uncomfortable issues by reducing noise in rooms and corridors and increasing circulation to reduce odours. Second, hotel maintenance should be a priority, ensuring that the hotel is clean, tidy and in good condition. Likewise, hotel rooms' equipment, services and features are crucial for guest comfort and convenience and to avoid guest complaints. Third, guests highlight the hotel's location and the surrounding interests, its proximity to tourist attractions, places of interest and shopping areas. Therefore, the hotel should highlight gastronomic and cultural offers close to the hotel (stressing these offers). Fourth, the design and ambience of the hotel, hedonism and exclusivity, e.g. outdoor spaces and aesthetic facilities, are crucial to guests. In particular, our findings also suggest that hotels should (1) address (negative) issues related to family rooms, such as room size, odours and water quality, and (2) highlight the location and nearby amenities (neighbourhood) for guests travelling alone. Similarly, managers should adjust promotional efforts to meet guests' needs, for example, by providing excellent services by friendly, helpful and committed staff (responsible for taking reservations, cleaning rooms, planning parties and maintaining the building) or amenities to families (e.g. a good breakfast and stores) and compensating for negative experiences. Fifth, managers must pay attention to the features and facilities related to the comfort of rooms for guests travelling alone (e.g. room size, noise levels and window quality, that is, sleep). Finally, guests travelling in groups (compared to couples) use positive words (in their narratives) such as staff, location, amaze or rooftop. In contrast, they tend to use more negative words, such as water, book, air conditioning, pay, etc., which may indicate problems with the hotel's amenities or in-room facilities.
In summary, online platforms represent a two-way channel for producing and consuming information and co-creating experiences. Our project analyses the usefulness of data mining techniques as an intelligent tool to enhance the image of hotel establishments and, by extension, of Andalusia as an international tourist destination. Guest reviews or comments expressed in natural language allow customers to describe their experiences with hotel services. In other words, our study does not address the analysis of quantitative variables collected by designing a structured questionnaire. In contrast, it identifies performance issues that are subtle (and even hidden), challenging to diagnose and damaging to the hotel's reputation if not delved into by various disciplines with consistent teams. By natural language processing techniques and topic extraction (unsupervised learning model), our analysis confirms with higher precision and richness of data the results of the published literature on the components contributing to improving relational quality levels. Therefore, our results should be even more reliable and valid than the statistical results discussed in the work based solely on customer ratings (customer satisfaction) and perceptions obtained from satisfaction surveys on small samples of customers.
Finally, future research should further investigate the bias towards mostly positive reviews using a scale based, for example, on stars awarded. In addition, it should include a more significant number of guest descriptor variables, such as cultural script or demographic characteristics (e.g. age or income). Such variables may affect their stay evaluations. Research should also study other destinations with different personalities and backgrounds and seasonality patterns of tourists or tourist attractions to generalise the conclusions. Furthermore, Sainaghi and Baggio (2020) acknowledged that one of the main drawbacks is the complexity of differentiating the segments of business travellers on the one hand and leisure guests on the other. Future studies should focus on hotels located in the city centre and around transport hubs.
Accordingly, a significant limitation is also the potential for bias in big data. For example, data collected from online sources may be biased towards specific demographics, for instance, those who are more likely to be active on social networks. Therefore, data collection methods can introduce bias, such as self-selection or nonresponse bias, leading to inaccurate or unfair conclusions. In summary, it is essential to carefully consider the sources and methods of data collection and apply appropriate techniques to account for potential biases in the data, use a diverse set of data sources and be transparent about the limitations of the data to ensure that insights and conclusions are reliable and valid.
Figures
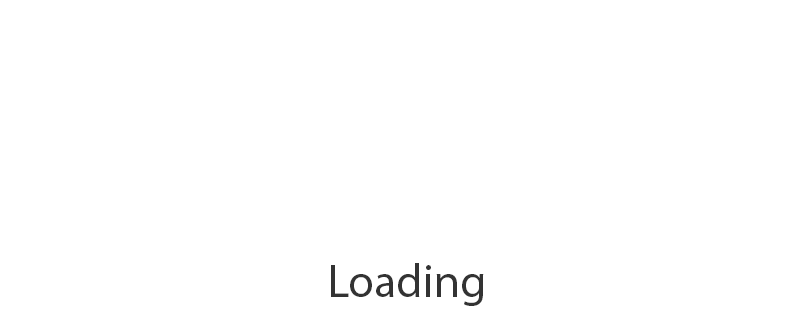
Figure 1
Example of positive and negative
reviews on Booking.com
NMF: words per topic and illustrative example of a narrative per topic
Topic | Label | Terms | Description | Example |
---|---|---|---|---|
Topic 0 | staff and facility aesthetics | staff, speak, desk, decor, facility, visit, member, property, site, decorate | Topic 0 is concerned with the robustness and aesthetic appeal of the hotel's facilities and physical properties. It is highlighted by keywords such as ‘staff’, ‘desk’, ‘decor’, and ‘facility’, illustrating the importance of efficient, well-versed personnel, enchanting decoration and comprehensively equipped facilities for an enthralling guest experience | Awesome location, very cheap for a stylish casona with the purest Spanish vibe. The staff was very helpful |
Topic 1 | walking distance to attractions | walk, distance, attraction, min, site, sight, place, alcazar [Alcazar], plaza, shop | The emphasis lies in the proximity of the hotel to notable attractions and places of interest. ‘Walk’, ‘distance’, ‘attraction’ and ‘site’ are prime words that denote the significance of accessible tourism sites, shopping plazas and historical edifices, facilitating an immersive cultural exploration for guests | This place is an outstanding location. So close to everything within walking distance. It was awesome! Nothing fancy, rooms pretty clean and nice personal. We will be back and recommend it! |
Topic 2 | parking facilities | park, car, garage, drive, street, space, access, nearby, euro, pay | Topic 2 is imbued with the challenges and conveniences of parking facilities at the hotel. Keywords such as ‘park’, 'car’, ‘garage’, and ‘street’ underscore the imperative of accessible and secure parking, further reinforcing its significance in enhancing the overall guest experience | Parking. If you put the hotel address on GPS, it takes you to the back side of the hotel, away from the hotel parking. The parking is located underground, and the path to the parking was so narrow that we could only park 1 of the two cars we took. We had to find another parking place for the 2nd car. The path to the parking is wide enough only for small cars |
Topic 3 | hotel swimming and relaxation | pool, swim, rooftop, relax, sun, heat, roof, towel, facility, gym | The focus here is on the recreational facilities offered by the hotel, particularly on swimming and relaxation. ‘Pool’, ‘swim’, ‘rooftop’, and ‘relax’ indicate the presence of well-appointed leisure amenities such as rooftop pools and sun lounges which contribute to an indulgent stay | The surroundings of the plunge pool are not hugely attractive. It could do with some living plants. Also, there is no safety box for belongings |
Topic 4 | sleep comfort and bedding | bed, pillow, sleep, mattress, sheet, request, decor, towel, book, air-conditioning | The critical elements of comfort, indicated by ‘bed’, ‘pillow’, ‘sleep’, and ‘mattress’, are accentuated in Topic 4. The need for optimum sleep comfort, facilitated by quality bedding and effective air conditioning, is paramount to ensuring a restful and rejuvenating stay for guests | Exceptional value for money. The bed was so comfortable-large room and free non-alcoholic minibar when registering for the club |
Topic 5 | breakfast and dining options | breakfast, buffet, food, option, selection, egg, variety, fruit, include, serve | Topic 5 explores the dining options offered by the hotel, with an emphasis on the quality and variety of breakfast items. ‘Breakfast’, ‘buffet’, ‘food’, and ‘option’ denote the importance of a diverse and enticing breakfast menu in creating a favourable first impression and guest experience | Breakfast was a box of pre-packed items. A lot of waste and non-recyclable packaging, though it came in a recyclable container |
Topic 6 | noise and comfort concerns | window, noise, street, hear, floor, door, people, sleep, air-conditioning, wall | Topic 6 brings to light concerns regarding noise and privacy. Words like ‘window’, ‘noise’, ‘street’, and ‘door’ underline the necessity of effective soundproofing measures to maintain an atmosphere of serenity, enabling guests to unwind undisturbed | Unfortunately, windows are inferior quality or are not appropriately sealed, and you can hear every noise from the street. It was impossible to sleep until three o'clock |
Topic 7 | centrally located to explore city | city, centre, locate, explore, heart, walk, foot, min, reach, taxi | The location appears to be a key aspect of Topic 7. ‘City’, ‘centre’, ‘locate’, and ‘explore’ suggest that the hotel's strategic positioning in the heart of the city enables guests to easily explore urban attractions, whether on foot or by taxi | The clean room and overall size of the room were great. The location is perfect in the city centre, next to big stores |
Topic 8 | panoramic views | view, rooftop, balcony, alhambra [Alhambra], river, stun, city, window, window, floor, terrace | Highlighting ‘view’, ‘rooftop’, ‘balcony’, and ‘alhambra’, Topic 8 emphasises the significance of breathtaking vistas in enhancing the hotel experience. Panoramic views, whether from a balcony, rooftop, or window, can provide an immersive sensory experience for guests | The location is excellent, and you can view the cathedral from the room. The room is quite large. However, I asked for a queen room, but it came out to be a twin room |
Topic 9 | bathroom sanitation | bathroom, bedroom, door, smell, space, towel, sink, glass, toilet, floor | Topic 9 deals with the hotel's sanitary facilities, specifically bathrooms. ‘Bathroom’, ‘bedroom’, ‘door’, and ‘smell’ underline the importance of well-maintained and clean restrooms equipped with modern amenities for guest comfort | They were very kind, and the big room was extremely clean. The bathroom was big and worked perfectly fine |
Topic 10 | nearby gastronomic experiences | restaurant, shop, food, nearby, cafe, dinner, eat, attraction, tapa, site | Topic 10 emphasises the importance of gastronomic experiences. The words ‘restaurant’, ‘shop’, ‘food’, and ‘nearby’ indicate that access to a variety of dining options, local shops, and cultural attractions can greatly enhance the guest's experience | Very stylish hotel–the H10 chain is one of our favourites-excellent value for money. Great bars and restaurants are nearby in the perfect location–close to Alameda Square |
Topic 11 | check-in and reception service | reception, lady, check-in, desk, book, check, check, hour, guy, arrive, wait | Words like ‘reception’, ‘lady’, ‘check-in’, and ‘desk’ spotlight the importance of smooth check-in/check-out procedures and courteous front desk service in creating positive first and last impressions, thus affecting overall guest satisfaction | The hotel was ideally situated in a central location. Our room was comfortable and provided everything that we needed. In addition, the team in reception were welcoming and helpful |
Topic 12 | overall guest experience and attributes | amaze, experience, rooftop, design, property, staff, build, stun, suite, visit | Topic 12 concentrates on the overall guest experience. ‘Amaze’, ‘experience’, ‘rooftop’, and ‘design’ signify the role of impressive design, stunning rooftops, and exceptional hospitality in crafting unforgettable hotel stays | Everything! This was our second stay at the hotel, and it's the perfect place to celebrate a special occasion. The staff was so kind and looked after us very well. THank you for a fantastic stay |
Topic 13 | comfortable public spaces | area, sit, lobby, seat, lounge, pool, dine, relax, space, courtyard | Underlined by ‘area’, ‘sit’, ‘lobby’, and ‘seat’, Topic 13 explores the significance of comfortable public spaces, such as lobbies, seating areas, and dining spaces, where guests can relax, socialise, or dine | Nice room, very lovely general installations. The hotel is in an exquisite old house with a pleasant outdoor area. Excellent bar waiters! |
Topic 14 | hot beverage facilities | coffee, facility, tea, tea, machine, kettle, water, drink, cup, fridge, expect | Topic 14 deals with the provision of hot beverage facilities in the hotel. ‘Coffee’, ‘facility’, ‘tea’, and ‘machine’ indicate that the availability of these facilities is a valued convenience for guests, contributing to a sense of home away from home | Facilities for making tea and coffee restaurant area in the hotel were of poor quality or not provided/available-otherwise, clean, modern rooms with good facilities |
Topic 15 | rooftop and terrace leisure | terrace, roof, drink, relax, sun, enjoy, floor, sit, overlook, jacuzzi | Topic 15, featuring words like ‘terrace’, ‘roof’, ‘drink’, and ‘relax’, accentuates the importance of leisure spaces. Terraces and rooftops that provide stunning vistas and serve as relaxation hubs can dramatically enrich the guest experience | A very interesting blend between tradition and modernity. Good to relax in the sauna/jacuzzi at the end of the day. Great spacious room with huge terrasse … |
Topic 16 | nightly comfort and air-conditioning | night, sleep, air-condition, book, turn, wake, expect, problem, change, heat | Highlighted by ‘night’, ‘sleep’, ‘air-condition’, and ‘book’, Topic 16 refers to the quality of sleep and the role of well-maintained air conditioning in ensuring a comfortable and restful night's sleep for guests | The only thing we didn't like was the, we suspect, illegal construction trash hauling going on at 3a both nights during our stay. Absolutely nothing the hotel could do about this, though |
Topic 17 | charming old town and location | town, locate, build, heart, courtyard, charm, visit, centre, access, bridge | With ‘town’, ‘locate’, ‘build’, and ‘heart’, Topic 17 underlines the allure of a hotel located in a charming town centre, offering ease of access to local attractions and the enchantment of heritage architecture | Friendly staff. Perfect breakfast. The lovely restored building is excellently located in the old town |
Topic 18 | guest concerns and resolutions | bite, feel, issue, lack, expect, people, smell, wall, floor, tire | Topic 18, encapsulated by the terms ‘bite’, ‘feel’, ‘issue’, and ‘lack’, addresses potential concerns or problems guests might encounter during their stay. It emphasises the importance of promptly resolving these issues to ensure that guests can unwind undisturbed | The housekeeping team was a bit loud in the mornings. Also, due to the season-opening, repairs were done in the hotel, paint jobs, people moving around etc. |
Topic 19 | shower amenities | shower, water, bath, floor, pressure, temperature, bottle, toilet, shampoo, break | Topic 19 explores sanitary facilities, specifically shower amenities. ‘Shower’, ‘water’, ‘bath’, and ‘floor’ highlight the importance of well-maintained and fully-functional shower facilities, clean bathrooms, and adequate water pressure and temperature for guest satisfaction | Some bath amenities were missing, like a shower cap and cotton pads-the cleanliness of the breakfast restaurant floor |
Topic 20 | service and customer relations | service, food, customer, order, drink, experience, offer, wait, menu, valet | The service aspect of the hotel industry is highlighted here. ‘Service’, ‘food’, ‘customer’, and ‘order’ suggest the crucial role of efficient and high-quality customer service in creating a delightful dining experience and a positive overall hotel experience | Very nice looking hotel with excellent service. A special shout out to Lucía, who we thought went out of her way to give us unbelievable service. She's a huge credit to this hotel, and we wish her the best of luck |
Topic 21 | convenient transportation access | alhambra [Alhambra], bus, station, train, visit, taxi, airport, min, palace, entrance | Keywords such as ‘alhambra’, ‘bus’ ‘station’, and ‘train’ underscore the significance of convenient transportation options and proximity to major tourist attractions such as the Alhambra, contributing to the overall guest experience | Charming hotel–perfect location!!! Very safe for solo travellers, convenient to bus stops (to go to Alhambra or train station), and everywhere else is walkable |
Topic 22 | flexible booking policies | day, time, book, leave, check-in, change, feel, arrive, spend, checkout | Topic 22 relates to time management during the hotel stay. ‘Day’, ‘time’, ‘book’, and ‘leave’ emphasise the importance of flexible booking and check-in/check-out policies in ensuring a hassle-free and enjoyable guest experience | 4 pm check-in is far too late, and on some days, my room wasn't cleaned until 3 pm, the hottest part of the day when I needed to be inside |
Topic 23 | value for money | price, book, pay, include, expect, euro, charge, offer, compare, rate | The terms ‘price’, ‘book’, ‘pay’, and ‘include’ in Topic 23 focus on the financial aspect of the hotel stay. The transparency and competitiveness of pricing and inclusion of value-added services are vital in influencing booking decisions and guest satisfaction | It was not a 3-star hotel. At best, it was a one-star hostel with 4-star pricing. Noisy, with small dingy rooms just about adequately clean |
Topic 24 | aesthetically pleasing design | love, feel, courtyard, design, decor, property, enjoy, patio, style, balcony | Topic 24, featuring ‘love’, ‘feel’, ‘courtyard’, and ‘design’, underscores the impact of aesthetically pleasing interiors and outdoor spaces on the overall guest experience. A hotel's design and decor can enhance the ambience, making guests feel more at home and appreciated | We loved this place very much. We loved the decor and the friendliness of the staff. We loved the comfortable room overlooking the courtyard. The breakfast was luxurious. And the value for money was amazing |
Source(s): Table created by the authors
Confusion matrix
Actual value/prediction | 0 | 1 |
---|---|---|
0 | 2.588 | 318 |
1 | 360 | 1.443 |
Note(s): 0: a positive review; 1: a negative review
Source(s): Table created by the authors
Summary: mode (categorical variables) and means (numerical variables)
Cluster | Total (*) | Noches | Viajero | Satisf | Topic 0 | Topic 6 | Topic 18 | Topic 1 | Topic 7 | Topic 17 | Topic 24 | Topic 12 |
---|---|---|---|---|---|---|---|---|---|---|---|---|
1 | 898 | 2–3 | solo | negative | 0.105 | 0.264 | 0.110 | 0.110 | 0.100 | 0.102 | 0.110 | 0.100 |
2 | 488 | 2–3 | couples | positive | 0.132 | 0.098 | 0.090 | 0.249 | 0.145 | 0.102 | 0.097 | 0.087 |
3 | 871 | 1 | groups | positive | 0.259 | 0.119 | 0.102 | 0.100 | 0.097 | 0.101 | 0.123 | 0.098 |
4 | 320 | 1 | families | negative | 0.102 | 0.138 | 0.289 | 0.099 | 0.095 | 0.095 | 0.094 | 0.086 |
5 | 363 | 1 | solo | positive | 0.140 | 0.116 | 0.091 | 0.088 | 0.267 | 0.112 | 0.099 | 0.088 |
6 | 356 | 2–3 | families | positive | 0.126 | 0.136 | 0.089 | 0.124 | 0.088 | 0.248 | 0.102 | 0.087 |
7 | 1,308 | 1 | families | negative | 0.122 | 0.130 | 0.126 | 0.125 | 0.120 | 0.124 | 0.132 | 0.121 |
8 | 208 | 1 | families | positive | 0.138 | 0.093 | 0.086 | 0.096 | 0.098 | 0.096 | 0.108 | 0.285 |
Note(s): * Class sizes have been balanced and intersected for analysis to avoid over-dimensioning the classes
Source(s): Table created by the authors
Reports
Balance of the Year of Tourism in Andalusia 2017, Junta de Andalucía.
Hotel Occupancy Survey 2022, Andalusian Institute of Statistics.
General Plan for Sustainable Tourism in Andalusia Horizon 2020, Junta de Andalucía.
Strategic Tourism Marketing Plan 2020, Junta de Andalucía.
References
Alarcón-Urbistondo, P., Rojas-de-Gracia, M.M. and Casado-Molina, A. (2023), “Proposal for employing user-generated content as a data source for measuring tourism destination image”, Journal of Hospitality and Tourism Research, Vol. 47 No. 4, pp. 643-664, doi: 10.1177/10963480211012756.
Archak, N., Ghose, A. and Ipeirotis, P.G. (2011), “Deriving the pricing power of product features by mining consumer reviews”, Management Science, Vol. 57 No. 8, pp. 1485-1509, doi: 10.1287/mnsc.1110.1370.
Bagozzi, R.P., Gopinath, M. and Nyer, P.U. (1999), “The role of emotions in marketing”, Journal of the Academy of Marketing Science, Vol. 27 No. 2, pp. 184-206, doi: 10.1177/0092070399272005.
Baker, J., Levy, M. and Evans, J.R. (1992), “An experimental approach to making retail store environmental decisions”, Journal of Retailing, Vol. 68 No. 4, p. 445, available at: https://www.proquest.com/scholarly-journals/experimental-approach-making-retail-store/docview/228646607/se-2
Ball‐Rokeach, S.J. (1985), “The origins of individual media‐system dependency: a sociological framework”, Communication Research, Vol. 12 No. 4, pp. 485-510, doi: 10.1177/009365085012004003.
Belarmino, A., Whalen, E., Koh, Y. and Bowen, J.T. (2019), “Comparing guests' key attributes of peer-to-peer accommodations and hotels: mixed-methods approach”, Current Issues in Tourism, Vol. 22 No. 1, pp. 1-7, doi: 10.1080/13683500.2017.1293623.
Benítez-Aurioles, B. (2019), “Is Airbnb bad for hotels?”, Current Issues in Tourism, Vol. 25 No. 19, pp. 3076-3079, doi: 10.1080/13683500.2019.1646226.
Campón-Cerro, A.M., Alves, H.B. and Hernández-Mogollón, J.M. (2015a), “Attachment as a factor in generating satisfaction with, and loyalty to, rural tourism destinations”, Tourism and Management Studies, Vol. 11 No. 1, pp. 70-76, available at: http://www.redalyc.org/articulo.oa?id=388743883008
Campón-Cerro, A.M., Hernández-Mogollón, J.M. and Alves, H.B. (2015b), “Sustainable improvement of competitiveness in rural tourism destinations: the quest for tourist loyalty in Spain”, Journal of Destination Marketing and Management, Vol. 6, pp. 252-266, doi: 10.1016/j.jdmm.2016.04.005.
Chevalier, J.A. and Mayzlin, D. (2006), “The effect of word of mouth on sales: online book reviews”, Journal of Marketing Research, Vol. 43 No. 3, pp. 345-354, doi: 10.1509/jmkr.43.3.345.
Chong, A.Y.L., Khong, K.W., Ma, T., McCabe, S. and Wang, Y. (2018), “Analyzing key influences of tourists' acceptance of online reviews in travel decisions”, Internet Research, Vol. 28 No. 3, pp. 564-586, doi: 10.1108/intr-05-2017-0212.
Chu, R.K.S. and Choi, T. (2000), “An importance-performance analysis of hotel selection factors in the Hong Kong hotel industry: a comparison of business and leisure travelers”, Tourism Management, Vol. 21 No. 4, pp. 363-377, doi: 10.1016/s0261-5177(99)00070-9.
Cotelo, J.M., Cruz, F.L., Troyano, J.A. and Ortega, F.J. (2015), “A modular approach for lexical normalisation applied to Spanish tweets”, Expert Systems with Applications, Vol. 42 No. 10, pp. 4743-4754, doi: 10.1016/j.eswa.2015.02.003.
Dann, D., Teubner, T. and Weinhardt, C. (2019), “Poster child and Guinea pig – insights from a structured literature review on Airbnb”, International Journal of Contemporary Hospitality Management, Vol. 31 No. 1, pp. 427-473, doi: 10.1108/ijchm-03-2018-0186.
Dolnicar, S. (2018), Peer-to-Peer Accommodation Networks: Pushing the Boundaries, Goodfellow, Oxford.
Festila, M. and Müller, S.D. (2017), “The impact of technology-mediated consumption on identity: the case of Airbnb”, Proceedings of the Annual Hawaii International Conference on System Sciences, pp. 54-63, doi: 10.24251/hicss.2017.007.
Forman, C., Ghose, A. and Wiesenfeld, B. (2008), “Examining the relationship between reviews and sales: the role of reviewer identity disclosure in electronicmarkets”, Information Systems Research, Vol. 19 No. 3, pp. 291-313, doi: 10.1287/isre.1080.0193.
Gallagher, R.J., Frank, M.R., Mitchell, L., Schwartz, A.J., Reagan, A.J., Danforth, Ch.M. and Dodds, P.S. (2021), “Generalized word shift graphs: a method for visualizing and explaining pairwise comparisons between texts”, EPJ Data Science, Vol. 10 No. 4, doi: 10.1140/epjds/s13688-021-00260-3.
Godes, D. and Mayzlin, D. (2004), “Using online conversations to study word‐of‐mouth communication”, Marketing Science, Vol. 23 No. 4, pp. 545-560, doi: 10.1287/mksc.1040.0071.
Gretzel, U. and Yoo, K.H. (2008), “Use and impact of online travel reviews”, in O'Connor, P., Höpken, W. and Gretzel, U. (Eds), Information and Communication Technologies in Tourism 2008, Springer, Vienna, pp. 35-46, doi: 10.1007/978-3-211-77280-5_4.
Gundlach, G.T., Achrol, R.S. and Mentzer, J.T. (1995), “The structure of commitment in exchange”, Journal of Marketing, Vol. 59 No. 1, pp. 78-92, doi: 10.1177/002224299505900107.
Guo, Y., Barnes, S.J. and Jia, Q. (2016), “Mining meaning from online ratings and reviews: tourist satisfaction analysis using Latent Dirichlet allocation”, Tourism Management, Vol. 59, pp. 467-483, doi: 10.1016/j.tourman.2016.09.009.
Hennig-Thurau, T., Gwinner, K.P., Walsh, G. and Gremler, D.D. (2004), “Electronic word-of-mouth via consumer-opinion platforms: what motivates consumers to articulate themselves on the Internet?”, Journal of Interactive Marketing, Vol. 18, pp. 38-52, doi: 10.1002/dir.10073.
Henseler, J. (2018), “Partial least squares path modeling: Quo vadis?”, Quality and Quantity, Vol. 52 No. 1, pp. 1-8, doi: 10.1007/s11135-018-0689-6.
Hernández Mogollón, J.M., Campon-Cerro, A.M. and Alves, H. (2013), “Authenticity in environmental high-quality destinations: a relevant factor for green tourism demand”, Environmental Engineering and Management Journal, Vol. 12 No. 10, pp. 1961-1970, doi: 10.30638/eemj.2013.245.
Jalilvand, M.R. and Samiei, N. (2012), “The impact of electronic word of mouth on a tourism destination choice: testing the theory of planned behavior”, Internet Research, Vol. 22 No. 5, pp. 591-612, doi: 10.1108/10662241211271563.
Kandampully, J. and Suhartanto, D. (2000), “Customer loyalty in the hotel industry: the role of customer satisfaction and image”, International Journal of Contemporary Hospitality Management, Vol. 12 No. 6, pp. 346-351, doi: 10.1108/09596110010342559.
Kessler, J. (2017), “Scattertext: a browser-based tool for visualising how corpora differ”, Proceedings of the 55th Annual Meeting of the Association for Computational Linguistics-System Demonstrations, Vancouver, Canada, July 30-August 4, pp. 85-90, doi: 10.18653/v1/p17-4015.
Lazos, F.J.M. and Steenkamp, J.B.E.M. (2005), “Emotions in consumer behaviour. A hierarchical approach”, Journal of Business Research, Vol. 58 No. 10, pp. 1437-1445, doi: 10.1016/j.jbusres.2003.09.013.
Lee, D.D. and Seung, H.S. (1999), “Learning the parts of objects by non-negative matrix factorisation”, Nature, Vol. 401, pp. 788-791, doi: 10.1038/44565.
Litvin, S.W., Goldsmith, R.E. and Pan, B. (2008), “Electronic word-of-mouth in hospitality and tourism management”, Tourism Management, Vol. 29 No. 3, pp. 458-468, doi: 10.1016/j.tourman.2007.05.011.
Liu, Z. and Park, S. (2015), “What makes a useful online review? Implication for travel product websites”, Tourism Management, Vol. 47, pp. 140-151, doi: 10.1016/j.tourman.2014.09.020.
Lundberg, S. and Lee, S.-I. (2017), “A unified approach to interpreting model predictions”, Advances in Neural Information Processing Systems, Vol. 30, NIPS 2017, doi: 10.48550/arXiv.1705.07874.
Lyu, J., Khan, A., Bibi, S., Chan, J.H. and Qi, X. (2022), “Big data in action: an overview of big data studies in tourism and hospitality literature”, Journal of Hospitality and Tourism Management, Vol. 51, pp. 346-360, doi: 10.1016/j.jhtm.2022.03.014.
Marchiori, E. and Cantoni, L. (2015), “The role of prior experience in the perception of a tourism destination in user-generated content”, Journal of Destination Marketing and Management, Vol. 4, pp. 194-201, doi: 10.1016/j.jdmm.2015.06.001.
Martins Gonçalves, H., Miranda Silva, G. and Gomes Martins, T. (2018), “Motivations for posting online reviews in the hotel industry”, Psychology and Marketing, Vol. 35 No. 11, pp. 807-817, doi: 10.1002/mar.21136.
Mihalcea, R. and Tarau, P. (2004), “TextRank: bringing order into text”, Proceedings of the 2004 Conference on Empirical Methods in Natural Language Processing (EMNLP), Barcelona, Association for Computational Linguistics, pp. 404-411, available at: https://aclanthology.org/W04-3252.pdf
Mitsopoulou, E., Moustaka, E., Kamariotou, M.I. and Kitsios, F.C. (2023), “User-generated content and social media platforms in digital marketing: determinants of perceived value and trust in travel information”, Operations Research in the Age of Digital Transformation and Business Analytics: BALCOR 2020, Springer International Publishing, Cham, pp. 235-241, Thessaloniki, Greece, September 30-October 3, 2020, doi: 10.1007/978-3-031-24294-6_25.
Mody, M., Suess, C. and Lehto, X. (2019), “Going back to its roots: can hospitableness provide hotels competitive advantage over the sharing economy?”, International Journal of Hospitality Management, Vol. 76, pp. 286-298, Part A, doi: 10.1016/j.ijhm.2018.05.017.
Monroe, B.L., Colaresi, M.P. and Quinn, K.M. (2008), “Fightin' words: lexical feature selection and evaluation for identifying the content of political conflict”, Political Analysis, Vol. 16 No. 4, pp. 372-403, doi: 10.1093/pan/mpn018.
Morgan, R.M. and Hunt, S.D. (1994), “The commitment-trust theory of relationship marketing”, Journal of Marketing, Vol. 58 No. 3, pp. 20-38, doi: 10.1177/002224299405800302.
Mudie, P., Cottam, A. and Raeside, R. (2003), “An exploratory study of consumption emotion in services”, The Service Industries Journal, Vol. 23 No. 5, pp. 84-106, doi: 10.1080/02642060308565625.
National Statistics Institute (2022), “INE (January 20, 2023)”, available at: https://www.ine.es/en/
Oliver, R. (1997), Satisfaction: A Behavioural Perspective on the Customer, McGraw-Hill, New York.
Oliver, R. (2010), “Customer satisfaction”, in Sheth, J.N. and Malhotra, N.K. (Eds) Wiley International Encyclopedia of Marketing, John Wiley & Sons, New Jersey, pp. 1-5.
Osman, H., D'Acunto, D. and Johns, N. (2019), “Home and away: why do consumers shy away from reporting negative experiences in the peer-to-peer realms?”, Psychology and Marketing, Vol. 36 No. 12, pp. 1162-1175, doi: 10.1002/mar.21264.
Parasuraman, A. and Grewal, D. (2000), “The impact of technology on the quality-value-loyalty chain: a research agenda”, Journal of the Academy of Marketing Science, Vol. 28 No. 1, pp. 168-174, doi: 10.1177/0092070300281015.
Parasuraman, A., Zeithaml, V.A. and Berry, L.L. (1988), “SERVQUAL: a multiple item scale for measuring, consumer perceptions of service quality”, Journal of Retailing, Vol. 64 No. 1, pp. 12-40, available at: https://psycnet.apa.org/record/1989-10632-001
Park, D.H., Lee, J. and Han, I. (2007), “The effect of online consumer reviews on consumer purchasing intention: the moderating role of involvement”, International Journal of Electronic Commerce, Vol. 11 No. 4, pp. 125-148, doi: 10.2753/jec1086-4415110405.
Poon, K.Y. and Huang, W.J. (2017), “Past experience, traveler personality and tripographics on intention to use Airbnb”, International Journal of Contemporary Hospitality Management, Vol. 29 No. 9, pp. 2425-2443, doi: 10.1108/ijchm-10-2016-0599.
Radojevic, T., Stanisic, N. and Stanic, N. (2015), “Ensuring positive feedback: factors that influence customer satisfaction in the contemporary hospitality industry”, Tourism Management, Vol. 51, pp. 13-21, doi: 10.1016/j.tourman.2015.04.002.
Raguseo, E., Neirotti, P. and Paolucci, E. (2017), “How small hotels can drive value their way in infomediation. The case of 'Italian hotels vs OTAs and TripAdvisor”, Information and Management, Vol. 54 No. 6, pp. 745-756, doi: 10.1016/j.im.2016.12.002.
Rigdon, E.E., Sarstedt, M. and Ringle, C.M. (2017), “On comparing results from CB-SEM and PLS- SEM: five perspectives and five recommendations”, Marketing ZFP, Vol. 39 No. 3, pp. 4-16, doi: 10.15358/0344-1369-2017-3-4.
Rodríguez, I. and San Martín, H. (2008), “Tourist satisfaction. A cognitive – affective model”, Annals of Tourism Research, Vol. 35 No. 2, pp. 551-573, doi: 10.1016/j.annals.2008.02.006.
Rong, J., Vu, H.Q., Law, R. and Li, G. (2012), “A behavioral analysis of web sharers and browsers in Hong Kong using targeted association rule mining”, Tourism Management, Vol. 33 No. 4, pp. 731-740, doi: 10.1016/j.tourman.2011.08.006.
Rygielski, C., Wang, J.-C. and Yen, D.C. (2002), “Data mining techniques for customer relationship management”, Technology in Society, Vol. 24, pp. 483-502, doi: 10.1016/s0160-791x(02)00038-6.
Sánchez-Franco, M.J. and Aramendia-Muneta, M.E. (2023), “Why do guests stay at Airbnb versus hotels? An empirical analysis of necessary and sufficient conditions”, Journal of Innovation and Knowledge, Vol. 8 No. 3, 100380, doi: 10.1016/j.jik.2023.100380.
Sánchez-Franco, M.J., Navarro-García, A. and Rondán-Cataluña, F.J. (2016), “Online customer service reviews in urban hotels: a data mining approach”, Psychology and Marketing, Vol. 33 No. 12, pp. 1174-1186, doi: 10.1002/mar.20955.
Sánchez-Franco, M.J., Navarro-García, A. and Rondán-Cataluña, F.J. (2019), “A naive Bayes strategy for classifying customer satisfaction: a study based on online reviews of hospitality services”, Journal of Business Research, Vol. 101, August, pp. 499-506.
Sánchez-Franco, M.J., Roldán, J.L. and Cepeda, G. (2018), “Understanding relationship quality in hospitality services: a study based on text analytics and Partial Least Squares”, Internet Research, Vol. 29 No. 3, pp. 478-503, doi: 10.1108/intr-12-2017-0531.
Sánchez-Franco, M.J., Troyano, J.A., Cruz, F.L. and Alonso-Dos-Santos, M. (2022), “Exploring the generation of online content by the user and its predictive influence on Relational quality. Application to the Andalusian hotel sector. SEPLN-PD 2022”, Annual Conference of the Spanish Association for Natural Language Processing 2022: Projects and Demonstrations, September 21-23, 2022, A Coruña, available at: https://hdl.handle.net/11441/140553
Sainaghi, R. and Baggio, R. (2020), “Substitution threat between Airbnb and hotels: Myth or reality?”, Annals of Tourism Research, Vol. 83, 102959, doi: 10.1016/j.annals.2020.102959.
Sann, R., Lai, P.-C., Liaw, S.-Y. and Chen, C.-T. (2022), “Predicting online complaining behavior in the hospitality industry: application of big data analytics to online reviews”, Sustainability, Vol. 14 No. 3, p. 1800, doi: 10.3390/su14031800.
scikit-learn developers (2020), “Sklearn.Decomposition.NMF-scikit-learn 0.23.2 documentation”, available at: https://scikit-learn.org/stable/modules/generated/sklearn.decomposition.NMF.html
Sim, J., Mak, B. and Jones, D. (2006), “A model of customer satisfaction and retention for hotels”, Journal of Quality Assurance in Hospitality and Tourism, Vol. 7 No. 3, pp. 1-23, doi: 10.1300/J162v07n03_01.
Sparks, B.A., Kam Fung So, K. and Bradley, G.L. (2016), “Responding to negative online reviews: the effects of hotel responses on customer inferences of trust and concern”, Tourism Management, Vol. 53, pp. 74-85, doi: 10.1016/j.tourman.2015.09.011.
Veloso, M. and Gomez-Suarez, M. (2023), “The influential role of hotel-generated content on social media”, Journal of Hospitality and Tourism Technology, Vol. 14 No. 2, pp. 245-257, doi: 10.1108/jhtt-08-2021-0241.
Vermeulen, I.E. and Seegers, D. (2009), “Tried and tested: the impact of online hotel reviews on consumer consideration”, Tourism Management, Vol. 30 No. 1, pp. 123-127, doi: 10.1016/j.tourman.2008.04.008.
Wu, L., Shen, H., Fan, A. and Mattila, A.S. (2017), “The impact of language style on consumers reactions to online reviews”, Tourism Management, Vol. 59, pp. 590-596, doi: 10.1016/j.tourman.2016.09.006.
Xu, X. and Li, Y. (2016), “The antecedents of customer satisfaction and dissatisfaction toward various types of hotels: a text mining approach”, International Journal of Hospitality Management, Vol. 55, pp. 57-69, doi: 10.1016/j.ijhm.2016.03.003.
Xu, H., Cheung, L.T., Lovett, J., Duan, X., Pei, Q. and Liang, D. (2023), “Understanding the influence of user-generated content on tourist loyalty behavior at a World Heritage cultural site”, Tourist Recreation Investigation, Vol. 48 No. 2, pp. 173-187, doi: 10.1080/02508281.2021.1913022.
Ye, Q., Law, R., Gu, B. and Chen, W. (2011), “The influence of user-generated content on traveler behavior: an empirical investigation on the effects of eWord-of-Mouth to hotel online bookings”, Computers in Human Behavior, Vol. 27 No. 2, pp. 634-639, doi: 10.1016/j.chb.2010.04.014.
Young, C.A., Corsun, D.L. and Xie, K.L. (2017), “Travelers' preferences for peer-to-peer (P2P) accommodations and hotels”, International Journal of Culture, Tourism, and Hospitality Research, Vol. 11 No. 4, pp. 465-482, doi: 10.1108/ijcthr-09-2016-0093.
Yu, Y. and Dean, A. (2001), “The contribution of emotional satisfaction to consumer loyalty”, International Journal of Service Industry Management, Vol. 12 No. 3, pp. 234-250, doi: 10.1108/09564230110393239.
Yu, M., Cheng, M., Yu, Z., Tan, J. and Li, Z. (2022), “Investigating Airbnb listings' amenities relative to hotels”, Current Issues in Tourism, Vol. 25 No. 19, pp. 3168-3185, doi: 10.1080/13683500.2020.1733497.
Zervas, G., Proserpio, D. and Byers, J.W. (2021), “A first look at online reputation on Airbnb, where every stay is above average”, Marketing Letters, Vol. 32 No. 1, pp. 1-16, doi: 10.1007/s11002-020-09546-4.
Zhu, L., Lin, Y. and Cheng, M. (2020), “Sentiment and guest satisfaction with peer-to-peer accommodation: when are online ratings more trustworthy?”, International Journal of Hospitality Management, Vol. 86 No. 3688, 102369, doi: 10.1016/j.ijhm.2019.102369.
Further reading
Garbarino, E. and Johnson, M.S. (1999), “The different roles of satisfaction, trust, and commitment in customer relationships”, Journal of Marketing, Vol. 63 No. 2, pp. 70-87, doi: 10.2307/1251946.
Hennig-Thurau, T. and Klee, A. (1997), “The impact of customer satisfaction and relationship quality on customer retention: a critical reassessment and model development”, Psychology and Marketing, Vol. 14 No. 8, pp. 737-764.
Xiang, Z., Schwartz, Z., Gerdes, J. and Uysal, M. (2015), “What can big data and text analytics tell us about hotel guest experience and satisfaction?”, International Journal of Hospitality Management, Vol. 44, pp. 120-130.
Acknowledgements
The authors are grateful to the Junta de Andalucía for funding the research (Project I + D + i FEDER Andalucía, 2014–2020, US-1380960). The authors thank Jason Kessler (creator of Scattertext) for his expertise and assistance throughout all aspects of Scattertext and for his help in understanding its functionality.
The authors are grateful to the Junta de Andalucía for funding the research (Project I+D+i FEDER Andalucía, 2014–2020, US-1380960). The authors thank Jason Kessler (creator of Scattertext) for his expertise and assistance throughout all aspects of Scattertext and for his help in understanding its functionality.
Corresponding author
About the authors
Manuel J. Sánchez-Franco, PhD, is Full Professor of e-Business Management and Social Communication at the Department of Business Administration and Marketing at the University of Sevilla (Spain) and collaborates on research projects with companies and public administrations. His research interests are in the areas of consumer behaviour and tourism research. He has published books, book chapters and research papers in top-ranked journals.
María de la Sierra Rey-Tienda is a double degree graduate in Business Administration and Law, a research fellow at the Department of Business Management at Loyola University of Andalusia and collaborates at the “Metropol Parasol” Chair about Sustainable Management and Innovative Marketing of Singular Sites in Urban Areas at University of Seville (Spain). Her research interests are in the areas of organisational culture, sustainable and smart mobility, and tourism. She has published research papers in indexed journals.