Abstract
Purpose
Critical infrastructures (CIs) for essential services such as water supply and electricity delivery are notoriously vulnerable to disruptions. While extant literature offers important insights into the resilience of CIs following large-scale disasters, our understanding of CI resilience to the more typical disruptions that affect CIs on a day-to-day basis remains limited. The present study investigates how the interorganizational (supply) network that uses and manages the CI can mitigate the adverse consequences of day-to-day disruptions.
Design/methodology/approach
Longitudinal archival data on 277 day-to-day disruptions within the Dutch national railway CI were collected and analyzed using generalized estimating equations.
Findings
The empirical results largely support the study’s predictions that day-to-day disruptions have greater adverse effects if they co-occur or are relatively unprecedented. The findings further show that the involved interorganizational network can enhance CI resilience to these disruptions, in particular, by increasing the overall level of cross-boundary information exchange between organizations inside the network.
Practical implications
This study helps managers to make well-informed choices regarding the target and intensity of their cross-boundary information-exchange efforts when dealing with day-to-day disruptions affecting their CI. The findings illustrate the importance of targeting cross-boundary information exchange at the complete interorganizational network responsible for the CI and to increase the intensity of such efforts when CI disruptions co-occur and/or are unprecedented.
Originality/value
This study contributes to our academic understanding of how network-level processes (i.e. cross-boundary information exchange) can be managed to ensure interorganizational (supply) networks’ resilience to day-to-day disruptions in a CI context. Subsequent research may draw from the conceptual framework advanced in the present study for examining additional supply network-level processes that can influence the effectiveness of entire supply networks. As such, the present research may assist scholars to move beyond a simple dyadic context and toward examining complete supply networks
Keywords
Citation
van den Adel, M.J., de Vries, T.A. and van Donk, D.P. (2022), "Resilience in interorganizational networks: dealing with day-to-day disruptions in critical infrastructures", Supply Chain Management, Vol. 27 No. 7, pp. 64-78. https://doi.org/10.1108/SCM-03-2021-0136
Publisher
:Emerald Publishing Limited
Copyright © 2021, Mitchell J. van den Adel, Thomas A. de Vries and Dirk Pieter van Donk.
License
Published by Emerald Publishing Limited. This article is published under the Creative Commons Attribution (CC BY 4.0) licence. Anyone may reproduce, distribute, translate and create derivative works of this article (for both commercial and noncommercial purposes), subject to full attribution to the original publication and authors. The full terms of this licence may be seen at http://creativecommons.org/licences/by/4.0/legalcode
1. Introduction
Organizations increasingly form networks around the supply, production and delivery of goods and services. Correspondingly, many scholars have shifted their attention from dyadic interorganizational relationships toward examining complete supply networks (Braziotis et al., 2013; Miemczyk et al., 2012), as reflected in contemporary research published in Supply Chain Management: An International Journal (e.g. Gremyr and Halldorsson, 2021; Huang et al., 2020; Touboulic et al., 2018). Within such supply networks, organizations critically depend on one another’s inputs and performance to realize individual firm-level goals (e.g. production schedule adherence), as well as joint network-level outcomes (e.g. environmental sustainability; Kim et al., 2011). One particularly consequential type of supply network is used for operating and maintaining critical infrastructures (CIs; Linnenluecke, 2017). CIs provide essential services such as water supply, transportation and electricity delivery, which generally are mutually interdependent and nonsubstitutable, with few or no alternative systems that can deliver the same service (De Bruijne and Van Eeten, 2007; Egan, 2007).
As is common for supply networks (Tenhiälä and Salvador, 2014), a CI is affected on a daily basis by smaller disruptions (e.g. human error, malfunctioning equipment) that interrupt service provision. Although initially small and local, these disruptions need to be contained quickly to prevent their consequences from spreading across the CI (Comfort et al., 2012; Roux-Dufort, 2007). Dealing with disruptions is, however, particularly complicated and challenging within CIs, because the involved public and private organizations often have different or even competing (commercial) interests and working methods (Boin and Lodge, 2016; van der Vegt et al., 2015). Moreover, given the complex interdependencies within these networks, any local disruption or minor mistake made by one organization in managing a disruption can reverberate throughout the CIs (Ouyang, 2014; Wu et al., 2016). For example, one rail carrier’s miscalculated schedule change resulted in canceled, delayed and over-crowded trains throughout the UK for nearly two months (Transport Committee, 2018). The success of the interorganizational (supply) network in handling disruptions effectively is reflected in the resilience of the CI. When facing disruptions, a resilient CI quickly restores or even maintains its functionality, whereas a CI that lacks resilience faces prolonged downtime and impaired functioning (Boin and McConnell, 2007; Tukamuhabwa et al., 2015).
Although extant research has provided important insights into CI resilience, key ambiguities remain in our understanding of what the responsible interorganizational network can do to minimize the adverse consequences of day-to-day CI disruptions (Linnenluecke, 2017; Zhang et al., 2018). First, most supply chain management research on network-level phenomena in general (Braziotis et al., 2013; Miemczyk et al., 2012) and network resilience specifically (Scholten et al., 2020; Tukamuhabwa et al., 2015) has focused on examining the role of a focal organization or a specific dyadic interorganizational relationship. Consequently, this research fails to capture the non-linear and complex interdependencies among all organizations that collectively form a supply network (Kim et al., 2011; Pournader et al., 2016). Second, prior research on CI resilience mostly focuses on the functioning of CIs in the aftermath of rare, high-profile disruptive events, such as Hurricane Katrina (Cigler, 2007) and the 2008 credit crisis (Corder, 2009), rather than examining what the involved interorganizational network can do to manage the more typical day-to-day disruptions that affect a CI on a recurring basis (Comfort et al., 2012; Roux-Dufort, 2007). Importantly, whereas larger disruptions are handled by centralized and dedicated organizational systems (e.g. incident command structures; Bigley and Roberts, 2001), a CI lacks such centralized support when dealing with day-to-day disruptions and involved organizations must often organize joint responses in addition to their regular daily activities (Comfort et al., 2012; Roux-Dufort, 2007). Furthermore, while large-scale disruptions typically all represent nonroutine and complicated events, day-to-day disruptions vary widely on those dimensions. The interorganizational network must, therefore, be ready to deal with day-to-day disruptions that are routine and simple, as well as those that are relatively more complicated and nonroutine (Boin and Van Eeten, 2013). Given the distinctive challenges, it is unlikely that insights into large-scale disruption management from existing research can inform organizations regarding how to deal effectively with the smaller CI disruptions that are more frequent and varied (Tenhiälä and Salvador, 2014; Tukamuhabwa et al., 2017).
Consequently, the present study sets out to provide a better understanding of how the interorganizational network using and managing a CI can enhance the day-to-day resilience of the CI. To do so, we use organizational information processing theory (OIPT; Galbraith, 1974, 1977), as an influential conceptual perspective on how organizations can manage consequential events such as disruptions that cannot be fully planned for in advance (Azadegan et al., 2020; Manhart et al., 2020). OIPT suggests that the information processing demands associated with these events typically increase when they become increasingly complicated or unfamiliar (i.e. nonroutine; Bensaou and Venkatraman, 1995; Rudolph and Repenning, 2002). When information processing demands increase, OIPT subsequently asserts that more intense information exchange between different parts within and across organizations (i.e. cross-boundary) is needed to process larger amounts of information quickly (Galbraith, 1977; Tushman and Nadler, 1978). Based on these conceptual insights, we suggest that the interorganizational network responsible for operating a CI should match its degree of cross-boundary information exchange to the characteristics of the disruption that faces the CI. Specifically, we propose that organizations inside the network can deal most effectively with CI disruptions when they align the intensity of their cross-boundary information exchange with the complicatedness and nonroutineness of the day-to-day disruptions they face.
We test our predictions using objective, longitudinal data on 277 day-to-day disruptions within the Dutch national railway system, one of the busiest and densest transportation CIs in Europe (Ramaekers et al., 2009). Our findings reveal that the benefits of cross-boundary information exchange depend on the characteristics of the smaller, more typical disruptions, supporting earlier claims that such information exchange may not be equally effective in all situations (Quick and Feldman, 2014; van der Vegt et al., 2015). Our focus on day-to-day disruptions extends both the extant CI resilience research and broader literature on disruption management in supply networks (Linnenluecke, 2017; Tukamuhabwa et al., 2017). By theorizing and collecting data beyond the dyadic level, we further contribute to the growing body of research on (service) supply networks in general (Braziotis et al., 2013; Gremyr and Halldorsson, 2021) and, in particular, to the scarce empirical research on their resilience (Pournader et al., 2016; Tukamuhabwa et al., 2015). In doing so, we also demonstrate how OIPT can be elevated beyond a single organization or a single, dyadic interorganizational relationship to develop further insights into supply network-level phenomena (e.g. sustainability; Busse et al., 2017). Practically, this study will help managers and organizational administrators to better understand when cross-boundary information exchange inside their interorganizational network could mitigate the impact of CI disruptions.
2. Theoretical framework and hypothesis development
2.1 Service supply networks, day-to-day disruptions and critical infrastructure resilience
A supply network is “a network of connected and interdependent organizations mutually and co-operatively working together to control, manage, and improve the flow of materials and information from suppliers to end users” (Aitken, 1998, p. 2; see also Braziotis et al., 2013). An interorganizational network involved in operating and managing a CI is a special type of such a (service) supply network in which different organizations coordinate their actions and combine their resources (e.g. physical infrastructure, equipment) on a daily basis to ensure effective functioning of the CI. A particularly salient task of this interorganizational network is to ensure CI resilience by dealing with disruptive events that can compromise the continuity of the CI’s services (Boin and McConnell, 2007; Linnenluecke, 2017). An important subcategory of such events are the day-to-day disruptions that represent “less dramatic but more frequent events, such as suppliers’ delivery failures, machine breakdowns, and changes to the specifications of customer orders, which nonetheless represent an important managerial concern” (Tenhiälä and Salvador, 2014, p. 439; Scholten et al., 2020; Tukamuhabwa et al., 2017).
The resilience of a supply network can generally be described as its capability to prepare for and respond to disruptions, and thereby to quickly restore its performance to the same level as before the disruption (Chowdhury and Quaddus, 2016; Pournader et al., 2016; Sawyerr and Harrison, 2020). Within CIs, such resilience can thus be directly observed in how quickly the involved interorganizational network restores the delivery of services to end users. Correspondingly, we consider a CI’s resilience to day-to-day disruptions by its recovery time – i.e. the amount of time the involved interorganizational network needs to successfully develop and implement countermeasures that fully mitigate a disruption’s impact on the overall CI (Mattsson and Jenelius, 2015; Wildavsky, 1988). A shorter recovery time indicates that the organizations have isolated the disruption’s impact quickly, effectively preventing it from completely paralyzing the CI (Boin and McConnell, 2007; Zhang et al., 2018). By contrast, a longer recovery time signifies that the organizations have failed to identify the cause of the disruption or to develop a solution. In such cases, the disruption continues to hurt functionality and can cause major problems for CIs (Christianson et al., 2008; McDaniels et al., 2008). Accordingly, we define CI resilience as “the amount of time the system [i.e. the CI] takes to recover to its pre-[disruption] level of performance after experiencing a drop off in performance” (Britt, 1988, p. 60).
2.2 Developing an information-processing perspective on critical infrastructure resilience
To ensure CI resilience to day-to-day disruptions, organizations within the involved interorganizational network face the challenging task of gathering, combining and interpreting all relevant information for making well-informed decisions on what kind of countermeasures need to be implemented (Boin and Van Eeten, 2013; Quick and Feldman, 2014; Weick and Sutcliffe, 2011). An important theoretical perspective on how organizations may effectively deal with this challenging task is offered by OIPT (Galbraith, 1974, 1977). Originally developed as an intraorganizational theory, OIPT has subsequently been extended to better elucidate a focal (buying) organization’s behavior and performance within dyadic interorganizational relationships (Bensaou and Venkatraman, 1995), and recently also within supply networks (Busse et al., 2017). We draw on this latter line of research to further extend OIPT to the network level of analysis.
Fundamentally, OIPT revolves around organizations’ “ability to handle the nonroutine, consequential events that cannot be anticipated and planned for in advance” (Galbraith, 1974, p. 30). As such, OIPT offers valuable insights into how organizations may deal with disruptions (Azadegan et al., 2020; Bode and Macdonald, 2017; Manhart et al., 2020). According to OIPT, organizations can handle “nonroutine, consequential events” by either reducing the amount of information that needs to be processed or increasing their capacity to process information. OIPT further suggests that the effectiveness of either approach depends on how complicated and unfamiliar the unexpected event is (Rudolph and Repenning, 2002; Tushman and Nadler, 1978). To deal with less complicated and more familiar events, organizations can reduce the need for information processing by establishing slack resources and capacity or lowering the interdependencies between tasks. To handle more complicated and unfamiliar events, however, these two strategies may be infeasible (Galbraith, 1974, 1977). In such cases, OIPT asserts that organizations should attempt to increase their information processing capacity by investing in formalized information systems or establishing lateral relationships between different parts of the organization (Bensaou and Venkatraman, 1995; Tushman and Nadler, 1978).
Integrating these conceptual insights from OIPT with broader resilience research, we suggest that the typical day-to-day disruptions that affect a CI are more complicated when they co-occur (Rudolph and Repenning, 2002; Zobel and Khansa, 2014), and that they are unfamiliar when they represent unprecedented events requiring nonroutine responses (Ambulkar et al., 2015; Bode et al., 2011). We further extend the intraorganizational-level perspective of OIPT by emphasizing that, within the overall interorganizational network, the members of the different organizations need to collectively engage in more intense lateral (i.e. cross-boundary) information exchange when they face co-occurring and nonroutine CI disruptions (Figure 1). We focus on cross-boundary information exchange, as opposed to other strategies recommended by OIPT (e.g. formalized information systems), because it enables real-time coordination and adjustments during disruption management, and therefore, has been identified as a primary strategy with which to enable resilience in supply networks (Bode and Wagner, 2015; Sawyerr and Harrison, 2020; Scholten and Schilder, 2015).
2.3 Disruption co-occurrence and critical infrastructure resilience
Disruption co-occurrence refers to the number of CI disruptions concurrently confronting the involved interorganizational network when resolving the focal disruption (Davis et al., 2020; Sahebjamnia et al., 2018; Zobel and Khansa, 2014). When a particular day-to-day disruption coincides with many other such disruptions within the same CI, organizations inside the network may become overwhelmed and overloaded if they lack capacity to collect the required information. Organizations may, therefore, need more time to interpret and process all relevant information for a specific disruption that coincides with other disruptions, which can subsequently delay the development of an effective countermeasure to the focal disruption (Melnyk et al., 2009; Rudolph and Repenning, 2002). Moreover, the affected organizations need time to consider and control the interdependencies between co-occurring disruptions that may affect interrelated parts of the CI (Bode and Wagner, 2015; Ouyang, 2014). When more time is needed to deal with a day-to-day disruption, its detrimental consequences will persist for a longer period and possibly spread within the CI, decreasing its resilience (Cooke and Rohleder, 2006; Wildavsky, 1988). Conversely, in the case of only one disruption, involved organizations can focus on seeking and processing information to resolve that specific disruption (Davis et al., 2020; Zhang et al., 2018). In such circumstances, organizations have no need to explore complex interdependencies among disruptions, enabling them to develop countermeasures more quickly and to ensure the CI’s resilience to the focal disruption (Bode et al., 2011; Rudolph and Repenning, 2002). Therefore, we posit:
There is a negative relationship between disruption co-occurrence and CI resilience to the focal disruption.
2.4 Disruption nonroutineness and critical infrastructure resilience
Disruption nonroutineness refers to an interorganizational network’s unfamiliarity or lack of experience with a specific type of disruption to its CI (Hult et al., 2004; McDaniels et al., 2008). As with disruption co-occurrence, we expect that more nonroutine disruptions will place greater demands on organizations inside the network, potentially overloading them and reducing resilience. A smaller, recurring disruption is nonroutine when the interorganizational network has not recently encountered it. In such cases, organizations are generally unaware of the available options for addressing the disruption, and they, therefore, experience “difficulty determining the form and the strength of a response that restores […] stability” in the CI’s operations (Bode et al., 2011, p. 839; Manhart et al., 2020). Consequently, they must devote considerable time and effort to identifying, assessing and then implementing responses to smaller disruptions of which they have little or no relevant prior experience (Christianson et al., 2008; Rinaldi et al., 2001). Again, when organizations need more time to develop countermeasures for a disruption and its effects are prolonged, the CI’s resilience decreases. By contrast, day-to-day disruptions that have occurred more routinely in the recent past are more familiar to the affected organizations, which are, thus, likely to be aware of the available options for resolving them effectively (Azadegan et al., 2020; Tushman and Nadler, 1978). Accordingly, an interorganizational network may require less time to resolve these disruptions (Ambulkar et al., 2015; Tukamuhabwa et al., 2015). Hence, we predict:
There is a negative relationship between disruption nonroutineness and CI resilience to the focal disruption.
2.5 The moderating role of cross-boundary information exchange
Cross-boundary information exchange refers to the efforts of an organization’s members to laterally approach individuals from different teams and organizations to obtain and provide access to information and expertise and to establish shared awareness (Marrone, 2010; Scholten and Schilder, 2015). Prior resilience research in supply networks has positioned such information sharing across organizations as a prerequisite for effectively resolving disruptions (Fan and Stevenson, 2018; Jüttner and Maklan, 2011; Sawyerr and Harrison, 2020). Applying the intraorganizational logic of OIPT and integrating it with this broader resilience research, we propose that cross-boundary information exchange weakens the adverse effect of disruption co-occurrence on CI resilience. We expect that, without cross-boundary information exchange, an interorganizational network will have restricted ability to handle the extensive information processing demands created by co-occurring CI disruptions (Galbraith, 1974; Tushman and Nadler, 1978). In such situations, organizations inside the network may be unable to coordinate efforts or to evaluate causes and solutions. They may subsequently lose oversight of the overall situation and specifically the focal disruption, preventing the quick restoration of functionality for the overall CI (Bode and Wagner, 2015; Pescaroli and Kelman, 2017). That is, extensive information processing demands created by disruption co-occurrence may “overwhelm information processing capacity and create a vicious cycle of stress and declining performance” (Rudolph and Repenning, 2002, p. 25).
By contrast, pursuing intense cross-boundary information exchange when confronted with co-occurring day-to-day disruptions prevents the interorganizational network from entering this vicious cycle, as organizations that exchange information and coordinate across boundaries can divide work and focus on completing specific tasks. Through such cooperation, involved organizations can gather and interpret larger quantities of information more quickly and efficiently (Bensaou and Venkatraman, 1995; Galbraith, 1974), potentially reducing the time required to resolve both the focal disruption and the co-occurring disruptions (Bode and Macdonald, 2017). More intense cross-boundary information exchange also enables the interorganizational network to integrate scattered information better and asses potential interdependencies between co-occurring disruptions (Bode and Wagner, 2015; Sawyerr and Harrison, 2020). In particular, through the sharing of relevant information across organizations inside the network, cross-boundary information exchange facilitates a clearer overview of how the causes and consequences of smaller, co-occurring disruptions may interrelate (Quick and Feldman, 2014; Weick and Sutcliffe, 2011). A more structured overview of these smaller CI disruptions helps affected organizations to coordinate disruption responses collectively, thereby reducing the duration of the disruption to the CI’s operations (Boin and Van Eeten, 2013; Craighead et al., 2007; Sawyerr and Harrison, 2020) and avoiding duplicated or opposing actions across organizations that decrease the CI’s resilience to the disruptions (Fan and Stevenson, 2018; Quick and Feldman, 2014). Hence, we postulate:
Cross-boundary information exchange during a disruption moderates the relationship between disruption co-occurrence and CI resilience to the focal disruption. This negative relationship is accentuated when cross-boundary information exchange is lower and attenuated when cross-boundary information exchange is higher.
We further predict that cross-boundary information exchange will mitigate the adverse effect of disruption nonroutineness on a CI’s resilience. Nonroutine day-to-day disruptions require the interorganizational network to “step back from the situation at hand” and develop new responses by recombining existing knowledge or gathering new information and expertise (Rudolph and Repenning, 2002, p. 25). The information and expertise needed to offset these information processing demands seldom reside within a single organization, thereby necessitating information exchange with other organizations inside the network (Galbraith, 1974, 1977). In the absence thereof, organizations may lack the vital information and expertise needed to develop effective countermeasures against nonroutine day-to-day disruptions to their CI (Christianson et al., 2008; Hult et al., 2004). Without vital information and expertise, the organizations involved may develop an ineffective response to a nonroutine disruption, inadvertently reducing the CI’s resilience by allowing the disruption to persist or even spread further within the CI (Boin and Van Eeten, 2013; Zhang et al., 2018).
By contrast, organizations that engage collectively in cross-boundary information exchange when confronted with a nonroutine CI disruption can use one another’s relevant problem-solving expertise and capabilities to restore functionality more quickly and ensure the CI’s resilience to the nonroutine disruption. Specifically, cross-boundary information exchange allows organizations to engage in joint problem-solving with all other organizations inside their interorganizational network and to analyze the disruption situation from multiple perspectives (Pournader et al., 2016; Quick and Feldman, 2014; Scholten and Schilder, 2015). Moreover, by engaging in intensive cross-boundary information exchange, members from different organizations are better able to share and combine distributed information about the potential causes of a nonroutine disruption (Hult et al., 2004; Rudolph and Repenning, 2002). Joint problem-solving and unrestricted information flow may, in turn, give the overall interorganizational network better insight into the situation and better opportunities to develop effective, well-integrated countermeasures to nonroutine day-to-day disruptions (Rinaldi et al., 2001; Zhang et al., 2018). In addition, cross-boundary information exchange may facilitate more effective implementation of countermeasures. In a CI with more intense cross-boundary information exchange, organizations may be more aware of one another’s activities and able to align their efforts more quickly (Bode et al., 2011; Marrone, 2010). Such alignment is especially important in situations that are unfamiliar for the interorganizational network, as it may help to avoid the coordination problems that frequently inhibit responses to large disruptions that are inherently nonroutine (Berthod et al., 2017; Donahue and Tuohy, 2006). We thus propose:
Cross-boundary information exchange during a disruption moderates the relationship between disruption nonroutineness and CI resilience to the disruption. This negative relationship is accentuated when cross-boundary information exchange is lower and attenuated when cross-boundary information exchange is higher.
3. Research methodology
3.1 Research setting and data collection
We collected data on disruption management within the Dutch national railway system, one of the densest and busiest transport CIs in Europe (Ramaekers et al., 2009) with over 7,000 km of rail infrastructure and more than one million daily passengers. It is maintained and operated by an interorganizational network comprising subsidiaries and divisions of several autonomous passenger carriers, cargo transporters and infrastructure repair companies. Every year, these organizations encounter more than 3,000 typical CI disruptions, such as broken-down trains, derailments, collisions, rail infrastructure failures and extreme weather conditions (e.g. heavy wind, lighting strikes). The collected data includes, for example, a collision between a passenger train and a road vehicle that blocked an important railroad crossing, and a tree struck by lightning that had subsequently fallen on and destroyed an overhead power supply line essential for providing electricity to passenger and cargo trains on parts of the railway system. Characteristically for CIs (Van Eeten et al., 2011; Mattsson and Jenelius, 2015), there is a high level of interconnectedness within the Dutch railway system that can cause even small day-to-day disruptions to escalate and spread easily. The combination of frequent disruptions and cascading consequences makes this system particularly relevant for our research purposes.
We collected objective data on all day-to-day disruptions to the Dutch railway system during one month in the winter, which is one of the busiest periods when it comes to passenger numbers. We obtained verbatim transcripts from the joint information system used by the rail organizations’ subsidiaries and divisions to exchange information and coordinate responses to disruptions. These subsidiaries and divisions are typically regarded as standalone organizational entities, because they are located across The Netherlands and responsible for their own geographical region, infrastructure and equipment. As a result, they frequently experience interorganizational dynamics in their dealings with one another and also rely primarily on the joint information system to communicate and coordinate their efforts in response to the daily disruptions affecting the railway system. Overall, the obtained data comprises 31 distinct subsidiaries and divisions spread across ten different organizations.
A disruption is registered within the joint information system upon discovery by the back-office department of the rail infrastructure manager. Any incident registered within this system represents an objective disruption to the railway system (i.e. it restricts, delays or completely halts passenger and/or cargo transportation). After the disruption is registered, the rail organizations initiate their response efforts, which are also logged within this system. When the disruption is resolved and rail services can resume, the back-office employees sign off on and close the disruption in the information system. The collected transcripts are exact excerpts from this information system and contain descriptive information about the disruption (e.g. affected trajectory, established cause, assessment of impact), as well as a verbatim and timestamped copy of the interactions among the involved subsidiaries and divisions during the disruption. We used objective information from these obtained transcripts of the joint information system to operationalize our study variables.
We used a priori screening criteria to select relevant disruptions from the data. We excluded incidents who only affected one rail organization subsidiary or division or that lasted less than 1 h, because such incidents are too small to require network-level disruption response efforts and are, therefore, not relevant within the present research. We further excluded two disruptions caused by long-term maintenance projects, regarding them as irrelevant and outside the rail organizations’ control. Our final data set comprised 277 disruptions.
3.2 Measures
CI resilience. We measured the CI’s resilience to a day-to-day disruption by calculating how much time the rail organizations needed to resolve the disruption and restore rail services to their pre-disruption level (Chowdhury and Quaddus, 2016; Habermann et al., 2015; Wildavsky, 1988). Specifically, we computed the difference between the start and end times of the disruption, as registered in the joint information system. The start time indicates when rail organizations received the first message that the railway system was disrupted; the end time indicates when the disrupted route in the railway system became fully functional again. The duration of the disruptions ranged from a little over 1 h to close to 27 h, with a mean of about 3 h. Because our outcome measure was positively skewed, we log-transformed these values as recommended by Hair et al. (2013). We reverse coded this measure, such that a low score (i.e. a long recovery time) reflects low CI resilience and a high score (i.e. a short recovery time) reflects high CI resilience.
Disruption co-occurrence. We operationalized disruption co-occurrence by counting for each disruption the number of other disruptions in the railway system that were ongoing at the same time (Davis et al., 2020; Rudolph and Repenning, 2002; Sahebjamnia et al., 2018). Specifically, using the start and end times registered in the joint information system, we summed the number of day-to-day disruptions that either started or ended during the focal disruption or lasted for its entire duration. Because the Dutch railway CI is one of Europe’s densest transportation systems, this system-wide measure is appropriate and signifies the number of other day-to-day disruptions that affected the CI while the interorganizational network worked on resolving the focal disruption. The values obtained ranged from 0 to 37 co-occurring disruptions.
Disruption nonroutineness. To measure the nonroutineness of a specific day-to-day disruption, we determined how often disruptions had occurred at the same location in the railway system in the preceding 12 months. Adopting such a frequency measure is a predominant approach for capturing an organization’s experience or familiarity with (i.e. the routineness of) a particular type of event or situation (Bode et al., 2011; Haunschild and Sullivan, 2002; Hult et al., 2004; Rudolph and Repenning, 2002). Because the effect of prior experience depreciates over time and the frequency of day-to-day disruptions in the present research setting is relatively high, we followed suggestions by Haunschild and Sullivan (2002) and others (Baum and Ingram, 1998; Bode et al., 2011) and included a “recall period” of one year when calculating disruption nonroutineness.
In the present research setting, we focus on the location of disruptions for our nonroutineness measure because the place of the CI disruption largely determines the possibilities and constraints in finding and implementing an appropriate response. Each location (i.e. rail trajectory) is characterized by, for example, the options for alternative routes and modes of transportation, the maintenance condition of the physical infrastructure and equipment and the accessibility of the physical infrastructure (e.g. rural versus urban areas). As is typical for CIs (Egan, 2007; Ouyang, 2014), each of these characteristics needs to be considered when adapting existing solutions to resolve a disruption at a particular location, regardless of whether a railway switch broke down or a train collided with another vehicle. When a particular trajectory is disrupted more frequently (i.e. lower nonroutineness), the affected parties become more experienced in dealing with this broad range of location-specific considerations, and they may even be able to develop more standardized operating procedures in case of subsequent disruptions of a similar type (e.g. location; Mattsson and Jenelius, 2015; Zhang et al., 2018).
For each disruption, the joint information system contains information on the destination station affected. Using available archival data, we computed the frequency of disruptions affecting these destination stations during the preceding 12 months. We reverse-coded this measure, such that a low score (i.e. a frequent disruption) reflects low nonroutineness, and a high score (i.e. an infrequent disruption) reflects high nonroutineness.
Cross-boundary information exchange. Prior research indicates that cross-boundary information exchange reflects the degree to which organizations’ members interact with other individuals from different groups inside or outside their organization to share information and expertise (Marrone, 2010; Scholten and Schilder, 2015). As such, capturing the frequency of external communication is a predominant approach for measuring cross-boundary information exchange (Cai et al, 2017; Marrone et al., 2007; De Vries et al., 2014). Accordingly, we measured cross-boundary information exchange as the frequency with which the rail organizations’ subsidiaries and divisions communicated with one another through the joint information system to resolve a specific day-to-day disruption. Each disruption receives its own unique section within the information system, in which only communications on resolving that specific disruption may be shared. Such communications are, therefore, not confounded by communications related to other disruptions. Within each section, communications typically occur between subsidiaries and divisions both within and between organizations. However, because each organization’s subsidiaries and divisions are dispersed geographically and operate autonomously, intra- and interorganizational communications are not perceived or administered differently within the joint information system, supporting our treatment of the subsidiaries and divisions as standalone organizational entities.
The rail organizations adhere to strict communication guidelines when using the joint information system. Any misplaced or redundant interactions are removed from the information system, preventing potential information overload. Consequently, all communication in this information system is task related and directly relevant to resolving the specific day-to-day disruption. The rail organizations are required to communicate their response efforts through the joint information system and may only use backup systems in cases of emergency (i.e. when the primary system fails). We normalized our cross-boundary information exchange measure for both the duration of a disruption and the number of involved divisions. Specifically, disruptions that last longer or involve more divisions are more likely to have a higher total number of interactions. This normalization thus allowed us to measure the intensity rather than the overall quantity of cross-boundary information exchange between all organizations within the interorganizational network. In other words, our cross-boundary information exchange measure reflects the average number of interactions per hour per division specifically directed toward resolving a disruption.
3.3 Control variables
To rule out alternative explanations for our results, we followed best practice recommendations (e.g. Helmuth et al., 2015) and selected control variables that could determine CI resilience beyond our hypothesized relationships. Specifically, prior research illustrates that more severe day-to-day disruptions may take longer to resolve (Craighead et al., 2007; Linnenluecke, 2017; Ouyang, 2014), thereby affecting the CI’s resilience. Hence, to rule out disruption severity as a potential confound, we obtained three measures reflecting disruption severity from the joint information system and included them as covariates in our analyses. The first two measures reflect the rail organizations’ a priori assessments of the expected severity of a day-to-day disruption at the moment it emerges. These assessments follow a comprehensive and standardized classification scheme and are administered by a dedicated group within the back-office department of the rail infrastructure manager.
The first a priori measure captured the anticipated societal impact of the disruption through reference to five categories: regular incidents (e.g. malfunctioning of standard equipment, bad weather), fire, accidents with casualties, hazardous substances and bomb or terrorist threats. We used regular incidents as our reference category and included dummy variables for each of the other four categories. The second a priori measure captured the disruption’s anticipated impact on the railway system, using a four-point scale, with ratings from 1 (limited impact) to 4 (severe impact). This measure, thus, reflects the rail organizations’ prediction about the potential impact of a disruption in case it is not adequately addressed. In other words, although disruptions with a system impact of rating 4 may indeed eventually cause a system breakdown, they still emerge from small and recurring deviances in the rail infrastructure, and, as such, can be considered day-to-day disruptions in origin. It is important to emphasize that, although these a priori severity classifications are largely standardized, the disruption response procedure cannot be standardized, as it will be predominantly determined by the (im)possibilities at the disruption’s location (i.e. disruption nonroutineness). A third measure reflecting disruption severity that we included as a covariate in our analyses was the number of rail subsidiaries and divisions that were involved in resolving the disruption. More subsidiaries and divisions are likely to become involved as the severity of a disruption increases, which would manifest in a direct relationship between the number of involved divisions and recovery time (i.e. CI resilience). In addition to the a priori measures, the number of involved divisions, thus, offers an appropriate ex post approximation of disruption severity.
3.4 Data analysis
The 277 day-to-day disruptions included in our final data set were distributed across 27 days, indicating that several disruptions began on the same day. Such disruptions may be affected by day-level characteristics such as the weather or number of passengers. Regular ordinary least squares regression analyses cannot statistically control for such day-level nesting and so could provide biased estimators. Following Ballinger (2004) and Hardin and Hilbe (2012), we, therefore, used generalized estimating equations (GEEs) with cluster-robust standard errors. GEEs with such specified standard errors provide maximum likelihood estimates that account for the nesting or nonindependence of multiple disruptions (Level 1, disruptions) that started the same day (Level 2, days). Our regression estimates are, as such, corrected for any differences across days (e.g. weather) that may partially explain an inherently longer or shorter duration of disruptions that began on a specific day. Adopting GEEs, thus, enabled us to control for omitted Level 2 variables and, as such, address a leading cause of endogeneity (Ketokivi and McIntosh, 2017; Lu et al., 2018). The nature of our collected data further reduces endogeneity concerns regarding reverse causality. Specifically, the archival and longitudinal data we collected enabled us to measure our dependent variable at a later point in time than our independent variables. We further explicitly tested for reverse causality and obtained no significant results. These results and more detailed information on how we alleviated endogeneity concerns are available from the first author upon request.
To correctly apply GEEs, we followed the steps recommended by Cui (2007) and Hardin and Hilbe (2012). First, we assessed which probability distribution best fitted with our dependent variable. We found that a normal (i.e. Gaussian) probability distribution best fitted our data. Second, we specified a so-called “identity link function” to indicate that our dependent variable was continuous and did not need to be transformed prior to the analyses. Third, we tested different correlation structures (e.g. autoregressive, exchangeable) to control for the day-level nesting within our data (i.e. how disruptions are affected by other disruptions that emerged during the same day). Based on model fit indices, we found that the independent correlation structure exhibited the best fit with our data (Cui, 2007). These model fit indices are available from the first author upon request.
Following Ballinger (2004) and Hardin and Hilbe (2012), we used GEE models with cluster-robust standard errors in a stepwise manner to test our hypotheses. To ease interpretation of our results, we first standardized all noncategorical predictor variables to have a mean of 0 and a standard deviation of 1 prior to the analysis. We then modeled only the intercept in Model 0, and subsequently added the study covariates in Model 1. Next, to test H1 and H2, we added the main effects of disruption co-occurrence and disruption nonroutineness in Model 2. We then entered the direct effect of cross-boundary information exchange in Model 3 prior to entering its interactions with disruption co-occurrence (H3) and disruption nonroutineness (H4) in Model 4.
To evaluate the fit of each model, we computed the corrected quasi likelihood under independence model criterion (QICC; Cui, 2007). The QICC is used to evaluate GEE model fit given a set correlation structure, where a smaller QICC indicates better model fit (Ballinger, 2004; Hardin and Hilbe, 2012). In addition to the QICC, we evaluated overall model fit based on the marginal R2 (Zheng, 2000). The marginal R2 is a simple extension for GEEs of the regular R2 statistic for ordinary least squares analyses, as it similarly denotes the amount of variance in CI resilience that is explained by the estimated model compared to the intercept-only model (Ballinger, 2004; Hardin and Hilbe, 2012).
4. Results
4.1 Descriptive statistics
Table 1 presents the descriptive statistics and bivariate correlations of our variables.The direct association of CI resilience with disruption co-occurrence is significant (r = −0.47, p < 0.01), which underlines its potential relevance as a determinant of CI recovery time after a day-to-day disruption. By contrast, the direct association of CI resilience with disruption nonroutineness is nonsignificant (r = 0.02, n.s.), which suggests a more intricate relationship with CI recovery time. Given the nested structure of the data, however, these correlations should be interpreted with caution (Hardin and Hilbe, 2012).
4.2 Hypothesis testing
Table 2 summarizes the results of our GEEs. The model fit indices in Table 2 indicate that each of our estimated models fitted significantly better than the null model (Ballinger, 2004; Hardin and Hilbe, 2012), with the exception of Model 1, which includes only our covariates. Each model further notably adds to the variance explained in CI resilience as reflected in the Δ R2marginal, with our full model explaining a total of 54% of this variance. H1 predicted a negative association between disruption co-occurrence and CI resilience to the disruption. Our results support this hypothesis (B = −0.317, SE = 0.051, p < 0.01; Table 2, Model 2). H2 predicted that disruption nonroutineness would be negatively associated with CI resilience to the disruption; however, we find no support for this hypothesis (B = 0.040, SE = 0.038, n.s.; Table 2, Model 2).
H3 posited that the relationship between disruption co-occurrence and CI resilience would be moderated by cross-boundary information exchange. Our results support this hypothesis (B = 0.141, SE = 0.027, p < 0.01; Table 2, Model 4). In the simple slope analyses depicted under (a) in Figure 2, we plotted the relationship between disruption co-occurrence and CI resilience (untransformed) at lower (−1 SD) and higher (+1 SD) levels of cross-boundary information exchange (Cohen et al., 2014). At lower levels of cross-boundary information exchange, disruption co-occurrence is significantly and negatively associated with CI resilience (B = −0.300, SE = 0.043, p < 0.01). By contrast, at higher levels of cross-boundary information exchange, the relationship between disruption co-occurrence and CI resilience is nonsignificant (B = −0.018, SE = 0.043, n.s.). These results indicate that cross-boundary information exchange attenuates the negative association between disruption co-occurrence and CI resilience.
H4 posited that the relationship between disruption nonroutineness and CI resilience would be moderated by cross-boundary information exchange. Our results support this hypothesis (B = 0.079, SE = 0.022, p < 0.01; Table 2, Model 4). In the simple slope analyses depicted under (b) in Figure 2, we plotted the relationship between disruption nonroutineness and CI resilience (untransformed) at lower (−1 SD) and higher (+1 SD) levels of cross-boundary information exchange (Cohen et al., 2014). At lower levels of cross-boundary information exchange, there is a nonsignificant association between disruption nonroutineness and CI resilience (B = −0.005, SE = 0.051, n.s.). By contrast, at higher levels of cross-boundary information exchange, the relationship between disruption nonroutineness and CI resilience is significantly positive (B = 0.153, SE = 0.039, p < 0.01). Overall, these results support H4.
4.3 Robustness checks
Following Helmuth et al. (2015), we examined whether we would continue to find support for our predictions if we excluded the three covariates from our analyses (i.e. to demonstrate robustness). We continued to find a significant relationship between disruption co-occurrence and CI resilience (H1; B = −0.312, SE = 0.054, p < 0.01). Likewise, the relationship between disruption nonroutineness and CI resilience remained nonsignificant (H2; B = 0.023, SE = 0.038, n.s.). Finally, cross-boundary information exchange continued to moderate the relationship of CI resilience with disruption co-occurrence (H3; B = 0.154, SE = 0.025, p < 0.01) and with disruption nonroutineness (H4; B = 0.061, SE = 0.021, p < 0.01). As a second robustness check, we examined the possibility of a three-way interaction between disruption co-occurrence, disruption nonroutineness and cross-boundary information exchange. The results indicate that this three-way interactive relationship was nonsignificant (B = 0.045, SE = 0.033, n.s.).
We further checked robustness by three alternative specifications of our estimated models. First, we extended the recall period used for our nonroutineness measure to two years (instead of the original and theoretically justified one year). Second, we included dummy variables for the different subsidiaries and divisions of the rail organizations to check for division-level fixed effects. Third, we adopted a first-order autoregressive (AR[1]) correlation structure to test for serial correlations between consecutive disruptions. The results of these three additional GEEs are not significantly different from those reported in Table 2, further confirming the robustness of our results. The exact statistics for these robustness checks are available from the first author upon request.
5. Discussion
5.1 Theoretical implications
This study has several theoretical implications. We contribute to CI resilience research by extending the logic of OIPT to understand how the interorganizational network using and managing a CI can minimize the adverse consequences of the typical disruptions that affect the CI on a daily basis. Our study helps to understand how the interorganizational network can effectively handle such disruptions, which fills important gaps in our current knowledge of CI resilience (Boin and Van Eeten, 2013; Zhang et al., 2018). Our study integrates insights from OIPT with those from the literature on supply network resilience to generate detailed insights into an interorganizational network’s daily management of smaller and more frequent disruptions. Specifically, it enabled us to examine how affected organizations’ joint alignment of their information sharing efforts with the characteristics of the day-to-day disruption strengthens the resilience of their shared CI. Our integrated approach to studying smaller and more recurring disruptions may be extended to research on supply network resilience beyond the specific context of CIs, which, to date, has similarly focused primarily on exceptionally large disasters (Scholten et al., 2020; Tenhiälä and Salvador, 2014), even though “most supply [networks] are much more likely to be dealing with chronic, repeated threats of disruption” (Tukamuhabwa et al., 2017, p. 487).
This study further contributes to resilience literature beyond the specific context of CIs by providing insights into the benefits of cross-boundary information exchange for the resilience of supply networks. In contrast to previous research (e.g. Ali et al., 2017; Tukamuhabwa et al., 2015), our study challenges the universal effectiveness of cross-boundary information exchange for the resilience of these networks by showing that the benefits of cross-boundary information exchange depend on the characteristics of the disruption that such a network faces. Cross-boundary information exchange is particularly effective for managing disruptions that are more complicated or nonroutine in nature. This supports Quick and Feldman’s (2014) notion that, particularly under unfavorable conditions, the benefits of cross-boundary information exchange will outweigh its possible drawbacks, such as protracted decision-making and consensus-seeking (see also Van der Vegt et al., 2015). The present study therefore contributes to resilience research by illustrating the importance for organizations to attune the information exchange within their overall network to the characteristics of external disruptions. As such, we answer recent calls in this literature to develop a better understanding of the relative effectiveness of specific mitigation strategies across different situations and disruptions (Ali et al., 2017; Ambulkar et al., 2015; Bode et al., 2011).
Moreover, we contribute to existing research by providing empirically validated insights into how different organizations within a service supply network work jointly (i.e. beyond their immediate tier) to resolve disruptions (Habermann et al., 2015; Pournader et al., 2016; Van der Vegt et al., 2015). Our theoretical framework extends information-processing arguments beyond the level of the single organization to business settings that are characterized by complex interdependencies between multiple autonomous organizations (Kim et al., 2011; Scholten and Schilder, 2015). Our corresponding network-level data and analyses subsequently help to address the “lack of empirical studies of how reliability can be upheld when it is no longer the outcome of the processes within one single organization” (Antonsen et al., 2010, p. 215; Pournader et al., 2016; Scholten et al., 2020). These advances in theory and data collection may be extended to disruption management in other (public) service networks, which are generally underexplored in resilience research, yet experience the same daily problems as more typical, goods-oriented supply networks (Eckerd and Girth, 2017; Tukamuhabwa et al., 2015). Similar to in a CI, for example, a small delay of a single truck can affect the performance of an entire distribution network of interrelated warehouses operating under a cross-docking strategy (Buijs and Wortmann, 2014). In addition, our approach may help address recent calls for research on when and how traditional supply chain management practices, such as those related to disruption management, could be adopted in public sector supply networks (Berthod et al., 2017; Fugate et al., 2019).
Beyond contributing to the resilience literature, we also illustrate how OIPT can be used as a network-level theoretical framework. We elaborate on insights from earlier applications of OIPT at the intra- and interorganizational levels by using the theory to explain the collective behavior of organizations within an interorganizational network in response to day-to-day disruptions. Specifically, we introduce and operationalize two distinct types of disruption-related determinants of information-processing demands to OIPT. We subsequently theorize and show how these demands make it necessary for organizations inside an interorganizational network to collectively engage in cross-boundary information exchange to increase their overall network’s capacity to process information. By taking the overall interorganizational network as our unit of analysis, we extend previous studies adopting OIPT to explain the behavior and decisions of specifically the focal (buying) organization within supply networks (Bode et al., 2011; Busse et al., 2017; Flynn et al., 2016). Taken together, our study may prove useful for researchers aiming to use OIPT at a network level of analysis, and it adds to the growing body of network-level supply chain management literature.
5.2 Practical implications
This study’s findings will assist both public and private managers in better handling the smaller disruptions that affect their CI on a daily basis. We show that these day-to-day disruptions to a CI can have more detrimental consequences if they co-occur with other disruptions or represent nonroutine situations. In such cases, our results suggest that managers should strive for and facilitate higher cross-boundary information exchange with other organizations inside their network to share information, develop well-integrated countermeasures and avoid duplicated or opposing operations across organizations. Our research emphasizes, however, that such cross-boundary information exchange should be at the level of the overall interorganizational network, rather than within dyadic interorganizational relationships. In particular, our findings reveal that direct communication and information exchange between all relevant organizations is paramount for increasing the CI’s day-to-day resilience. Fundamentally, when confronted by a disruption, the managers of disrupted organizations must be aware of its characteristics and collaborate with other organizations inside their network. In doing so, they will be able to resolve the disruption more swiftly and effectively, thus minimizing its impact.
5.3 Limitations and future research
Although this study has several important strengths (e.g. a unique sample of day-to-day disruptions), several limitations need to be recognized. First, our study design precludes causal inferences, as we relied on natural variance rather than manipulated study variables (as in experimental research). We mitigated potential concerns about reverse causality, however, by collecting longitudinal data and explicitly testing for this. Nevertheless, we acknowledge that future experimental or longitudinal research is needed before causal inference is justified.
A second limitation is our use of objective measures based on archival data. This measurement approach enabled us to overcome low power and other key weaknesses associated with more subjective research approaches such as surveys or observational studies (e.g. common method bias, recall and response bias; Helmuth et al., 2015). However, this approach also prevented us from capturing the fine-grained mechanisms and processes through which the disruption characteristics and cross-boundary information exchange might influence CI resilience. Future observational, experimental or case studies are needed to explore the detailed mechanisms underlying the relationships that our study illustrates.
A third potential limitation concerns our research context. The CI we examined provided a unique and highly relevant research context for this study. Specifically, the high frequency of disruptions provided a large data set for empirically testing our disruption-level hypotheses with sufficient statistical power. Nevertheless, it is unclear whether our results generalize to other types of CIs or (service) supply networks that are similarly recurrently exposed to smaller disruptions. Therefore, we encourage future studies to replicate our research in other field settings to determine whether our findings generalize to other CI contexts and (service) supply networks (e.g. manufacturing or distribution networks).
Future research could also further investigate the relationship between disruption nonroutineness and CI resilience. One possible explanation for the lack of support in our findings for this relationship is that involved organizations may differ in how much they seek to better understand the root causes of or solutions to (prior) day-to-day disruptions. When not inclined to explore the intricacies of the disruptions they face (i.e. passive disruption orientation), organizations may not benefit from prior experience (Ambulkar et al., 2015; Bode et al., 2011; Zhang et al., 2018). This potential moderating effect of disruption orientation warrants further investigation in future studies.
A final future research direction is to extend our conceptual framework to include the effectiveness of other mitigation strategies across the examined disruption characteristics, especially in relation to one another. Although cross-boundary information exchange remained effective for less adverse disruption characteristics, other mitigation strategies may be less uniformly effective and might even become counterproductive. For example, because of the associated costs and lost flexibility, redundancies in resources and capacities may not be a preferable mitigation strategy in every disruption context (Bode et al., 2011; van der Vegt et al., 2015). Therefore, we recommend future research explores in greater detail how the nature or characteristics of a disruption, rather than its mere occurrence, determine the effectiveness of a specific mitigation strategy – especially in relation to other proposed strategies.
6. Conclusion
This research used OIPT to develop an integrative framework with which to investigate the impact of smaller, recurring disruptions within a CI. We combined insights from broader resilience research and the CI literature to examine and illustrate how cross-boundary information exchange can be a decisive means for the involved interorganizational (supply) network to mitigate the detrimental consequences of day-to-day CI disruptions. When confronted with adverse disruption characteristics, such as co-occurring or nonroutine disruptions, cross-boundary information exchange was found to help CIs become more resilient by accelerating their recovery from the disruption. By contrast, cross-boundary information exchange was found to be less beneficial when disruptions represented events that are more isolated or routine. We hope this study will stimulate further research on the intricacies of cross-boundary information exchange in the context of day-to-day disruptions, and that it will help managers to implement appropriate levels of this mitigation strategy.
Figures
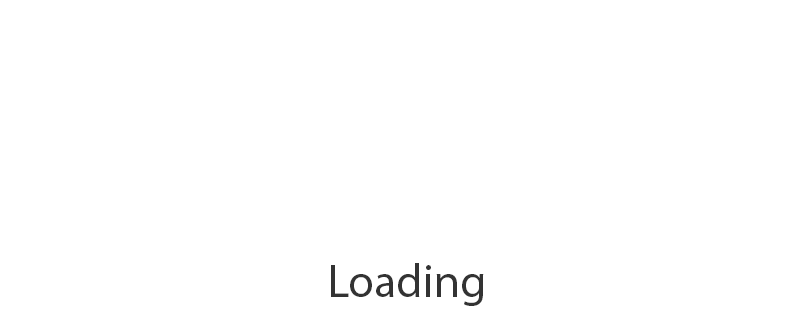
Figure 2
Simple slope analyses of the interaction effects: (a) cross-boundary information exchange as a moderator of the relationship between disruption co-occurrence and CI resilience (H3); (b) cross-boundary information exchange as a moderator of the relationship between disruption nonroutiness and CI resilience (H4)
Descriptive statistics
Variable | Mean | SD | 1 | 2 | 3 | 4 | 5 | 6 | 7 | 8 | 9 |
---|---|---|---|---|---|---|---|---|---|---|---|
1 CI resiliencea,b | −0.94 | 0.66 | |||||||||
2 Disruption co-occurrence | 5.96 | 5.86 | −0.47** | ||||||||
3 Disruption nonroutinenessb | −24.55 | 23.29 | 0.02 | 0.11 | |||||||
4 Cross-boundary information exchange | 1.04 | 0.76 | 0.53** | −0.25** | −0.22** | ||||||
5 Number of involved divisions | 2.97 | 2.14 | −0.19** | 0.02 | 0.13* | 0.03 | |||||
6 System impact | 1.99 | 0.82 | −0.02 | 0.06 | 0.02 | 0.06 | 0.17** | ||||
7 Societal impact: firec | 0.01 | 0.12 | 0.02 | −0.07 | 0.04 | 0.04 | −0.01 | 0.11 | |||
8 Societal impact: accidents with casualtiesc | 0.06 | 0.24 | 0.00 | −0.12* | 0.00 | −0.01 | 0.19** | −0.25** | 0.03 | ||
9 Societal impact: hazardous substancesc | 0.01 | 0.08 | 0.02 | −0.04 | –0.04 | 0.05 | −0.02 | −0.10 | −0.01 | −0.02 | |
10 Societal impact: bomb or terrorist threatsc | 0.01 | 0.08 | 0.00 | −0.07 | 0.03 | 0.00 | 0.20** | 0.05 | −0.01 | −0.02 | −0.01 |
N = 277 disruptions.
aLog-transformed.
bReverse-coded (resulting in a negative mean).
cDummy variable for one of the five categories of societal impact (reference category: regular incidents). * p < 0.05
**p < 0.01 (two-tailed)
Results of GEE analyses
CI resiliencea | |||||
---|---|---|---|---|---|
Model 0 | Model 1 | Model 2 | Model 3 | Model 4 | |
Independent variables | |||||
Intercept | −0.936 (0.040)** | −0.950 (0.044)** | −0.932 (0.060)** | −0.934 (0.050)** | −0.883 (0.038)** |
Disruption co−occurrence (DC) | −0.317 (0.051)** | −0.243 (0.043)** | −0.159 (0.034)** | ||
Disruption nonroutineness (DN) | 0.040 (0.038) | 0.104 (0.034)** | 0.074 (0.040) | ||
Cross-boundary information exchange (CBIE) | 0.313 (0.045)** | 0.383 (0.030)** | |||
DC × CBIE | 0.141 (0.027)** | ||||
DN × CBIE | 0.079 (0.022)** | ||||
Control variables | |||||
Number of involved divisions | −0.141 (0.037)** | −0.133 (0.030)** | −0.152 (0.028)** | −0.164 (0.028)** | |
System impact | 0.018 (0.037) | 0.026 (0.030) | 0.007 (0.034) | 0.011 (0.034) | |
Societal impact: fireb | 0.077 (0.076) | −0.128 (0.083) | −0.185 (0.163) | −0.200 (0.163) | |
Societal impact: accidents with casualtiesb | 0.147 (0.111) | −0.033 (0.112) | 0.011 (0.083) | 0.026 (0.078) | |
Societal impact: hazardous substancesb | 0.140 (0.253) | −0.018 (0.203) | −0.148 (0.081) | −0.109 (0.112) | |
Societal impact: bomb or terrorist threatsb | 0.286 (0.529) | −0.029 (0.486) | 0.084 (0.313) | 0.140 (0.325) | |
Model fit | |||||
QICCc | 120.655 | 127.730 | 105.286 | 83.255 | 78.412 |
R2marginald | 0.04 | 0.26 | 0.47 | 0.54 | |
Δ R2marginal | 0.04 | 0.22 | 0.21 | 0.07 |
N = 277 disruptions (Level 1) across 27 days (Level 2). Unstandardized regression coefficients are shown; robust standard errors are noted in parentheses.
aLog-transformed.
bReference category: regular incidents.
cA smaller QICC indicates better model fit.
dAs a measure of explained variance in the dependent variable, a larger R2marginal indicates better model fit.
**p < 0.01 (two-tailed)
References
Aitken, J.M. (1998), “Supply chain integration within the context of a supplier association: Case studies of four supplier associations”, Ph.D. thesis, Cranfield University.
Ali, A., Mahfouz, A. and Arisha, A. (2017), “Analysing supply chain resilience: integrating the constructs in a concept mapping framework via a systematic literature review, supply chain management”, Supply Chain Management: An International Journal, Vol. 22 No. 1, pp. 16-39.
Ambulkar, S., Blackhurst, J. and Grawe, S. (2015), “Firm’s resilience to supply chain disruptions: scale development and empirical examination”, Journal of Operations Management, Vols 33/34 No. 1, pp. 111-122.
Antonsen, S., Almklov, P.G., Fenstad, J. and Nybø, A. (2010), “Reliability consequences of liberalization in the electricity sector: existing research and remaining questions”, Journal of Contingencies and Crisis Management, Vol. 18 No. 4, pp. 208-219.
Azadegan, A., Parast, M.M., Lucianetti, L., Nishant, R. and Blackhurst, J. (2020), “Supply chain disruptions and business continuity: an empirical assessment”, Decision Sciences, Vol. 51 No. 1, pp. 38-73.
Ballinger, G.A. (2004), “Using generalized estimating equations for longitudinal data analysis”, Organizational Research Methods, Vol. 7 No. 2, pp. 127-150.
Baum, J.A.C. and Ingram, P. (1998), “Survival-enhancing learning in the Manhattan hotel industry, 1898–1980”, Management Science, Vol. 44 No. 7, pp. 996-1016.
Bensaou, M. and Venkatraman, N. (1995), “Configurations of interorganizational relationships: a comparison between U.S. and Japanese automakers”, Management Science, Vol. 41 No. 9, pp. 1471-1492.
Berthod, O., Grothe-Hammer, M., Müller-Seitz, G., Raab, J. and Sydow, J. (2017), “From high-reliability organizations to high-reliability networks: the dynamics of network governance in the face of emergency”, Journal of Public Administration Research and Theory, Vol. 27 No. 2, pp. 352-371.
Bigley, G.A. and Roberts, K.H. (2001), “The incident command system: high-reliability organizing for complex and volatile task environments”, Academy of Management Journal, Vol. 44 No. 6, pp. 1281-1299.
Bode, C. and Macdonald, J.R. (2017), “Stages of supply chain disruption response: direct, constraining, and mediating factors for impact mitigation”, Decision Sciences, Vol. 48 No. 5, pp. 836-874.
Bode, C. and Wagner, S.M. (2015), “Structural drivers of upstream supply chain complexity and the frequency of supply chain disruptions”, Journal of Operations Management, Vol. 36 No. 1, pp. 215-228.
Bode, C., Wagner, S.M., Petersen, K.J. and Ellram, L.M. (2011), “Understanding responses to supply chain disruptions: insights from information processing and resource dependence perspectives”, Academy of Management Journal, Vol. 54 No. 4, pp. 833-856.
Boin, A. and Lodge, M. (2016), “Designing resilient institutions for transboundary crisis management: a time for public administration”, Public Administration, Vol. 94 No. 2, pp. 289-298.
Boin, A. and McConnell, A. (2007), “Preparing for critical infrastructure breakdowns: the limits of crisis management and the need for resilience”, Journal of Contingencies and Crisis Management, Vol. 15 No. 1, pp. 50-59.
Boin, A. and Van Eeten, M.J.G. (2013), “The resilient organization: a critical appraisal”, Public Management Review, Vol. 15 No. 3, pp. 429-445.
Braziotis, C., Bourlakis, M., Rogers, H. and Tannock, J. (2013), “Supply chains and supply networks: distinctions and overlaps, supply chain management”, Supply Chain Management: An International Journal, Vol. 18 No. 6, pp. 644-652.
Britt, D.W. (1988), “Analyzing the shape of organizational adaptability in response to environmental jolts”, Clinical Sociology Review, Vol. 6 No. 1, pp. 59-75.
Buijs, P. and Wortmann, J.C. (2014), “Joint operational decision-making in collaborative transportation networks: the role of IT”, Supply Chain Management: An International Journal, Vol. 19 No. 2, pp. 200-210.
Busse, C., Meinlschmidt, J. and Foerstl, K. (2017), “Managing information processing needs in global supply chains: a prerequisite to sustainable supply chain management”, Journal of Supply Chain Management, Vol. 53 No. 1, pp. 87-113.
Cai, S., Jun, M. and Yang, Z. (2017), “The effects of boundary spanners’ personal relationships on interfirm collaboration and conflict: a study of the role of guanxi in China”, Journal of Supply Chain Management, Vol. 53 No. 3, pp. 19-40.
Chowdhury, M.H. and Quaddus, M. (2016), “Supply chain readiness, response and recovery for resilience, supply chain management”, Supply Chain Management: An International Journal, Vol. 21 No. 6, pp. 709-731.
Christianson, M.K., Farkas, M.T., Sutcliffe, K.M. and Weick, K.E. (2008), “Learning through rare events: significant interruptions at the Baltimore & Ohio railroad museum”, Organization Science, Vol. 20 No. 5, pp. 846-860.
Cigler, B.A. (2007), “The ‘big questions’ of Katrina and the 2005 great flood of new Orleans”, Public Administration Review, Vol. 67, pp. 64-76.
Cohen, J., Cohen, P., West, S.G. and Aiken, L.S. (2014), Applied Multiple Regression/Correlation Analysis for the Behavioral Sciences, 2nd ed., Psychology Press, New York, NY.
Comfort, L.K., Waugh, W.L. and Cigler, B.A. (2012), “Emergency management research and practice in public administration: emergence, evolution, expansion, and future directions”, Public Administration Review, Vol. 72 No. 4, pp. 539-548.
Cooke, D.L. and Rohleder, T.R. (2006), “Learning from incidents: from normal accidents to high reliability”, System Dynamics Review, Vol. 22 No. 3, pp. 213-239.
Corder, J.K. (2009), “The federal reserve system and the credit crisis”, Public Administration Review, Vol. 69 No. 4, pp. 623-631.
Craighead, C.W., Blackhurst, J.V., Rungtusanatham, M.J. and Handfield, R.B. (2007), “The severity of supply chain disruptions: design characteristics and mitigation capabilities”, Decision Sciences, Vol. 38 No. 1, pp. 131-156.
Cui, J. (2007), “QIC program and model selection in GEE analyses”, The Stata Journal: Promoting Communications on Statistics and Stata, Vol. 7 No. 2, pp. 209-220.
Davis, Z., Zobel, C.W., Khansa, L. and Glick, R.E. (2020), “Emergency department resilience to disaster-level overcrowding: a component resilience framework for analysis and predictive modeling”, Journal of Operations Management, Vol. 66 Nos 1/2, pp. 54-66.
De Bruijne, M. and Van Eeten, M. (2007), “Systems that should have failed: critical infrastructure protection in an institutionally fragmented environment”, Journal of Contingencies and Crisis Management, Vol. 15 No. 1, pp. 18-29.
de Vries, T.A., Walter, F., van Der Vegt, G.S. and Essens, P.J.M.D. (2014), “Antecedents of individuals’ interteam coordination: broad functional experiences as a mixed blessing”, Academy of Management Journal, Vol. 57 No. 5, pp. 1334-1359.
Donahue, A.K. and Tuohy, R.V. (2006), “Lessons we don’t learn: a study of the lessons of disasters, why we repeat them, and how we can learn them”, Homeland Security Affairs, Vol. 2 No. 2, pp. 1-28.
Eckerd, A. and Girth, A.M. (2017), “Designing the buyer–supplier contract for risk management: assessing complexity and mission criticality”, Journal of Supply Chain Management, Vol. 53 No. 3, pp. 60-75.
Egan, M.J. (2007), “Anticipating future vulnerability: defining characteristics of increasingly critical infrastructure-like systems”, Journal of Contingencies and Crisis Management, Vol. 15 No. 1, pp. 4-17.
Fan, Y. and Stevenson, M. (2018), “Reading on and between the lines: risk identification in collaborative and adversarial buyer–supplier relationships, supply chain management”, Supply Chain Management: An International Journal, Vol. 23 No. 4, pp. 351-376.
Flynn, B.B., Koufteros, X. and Lu, G. (2016), “On theory in supply chain uncertainty and its implications for supply chain integration”, Journal of Supply Chain Management, Vol. 52 No. 3, pp. 3-27.
Fugate, B., Pagell, M. and Flynn, B.B. (2019), “From the editors: introduction to the emerging discourse incubator on the topic of research at the intersection of supply chain management and public policy and government regulation”, Journal of Supply Chain Management, Vol. 55 No. 2, pp. 3-5.
Galbraith, J.R. (1974), “Organization design: an information processing view”, Interfaces, Vol. 4 No. 3, pp. 28-36.
Galbraith, J.R. (1977), Organization Design, Addison-Wesley, Reading, MA.
Gremyr, I. and Halldorsson, A. (2021), “Guest editorial – Advancing service supply chains: conceptualisation and research directions, supply chain management”, Supply Chain Management: An International Journal, Vol. 26 No. 3, pp. 297-306.
Habermann, M., Blackhurst, J. and Metcalf, A.Y. (2015), “Keep your friends close? Supply chain design and disruption risk”, Decision Sciences, Vol. 46 No. 3, pp. 491-526.
Hair, J.F., Black, W.C., Babin, B.J. and Anderson, R.E. (2013), Multivariate Data Analysis, 7th ed., Prentice-Hall, NJ.
Hardin, J.W. and Hilbe, J.M. (2012), Generalized Estimating Equations, 2nd ed., Chapman & Hall/CRC, Boca Raton, FL.
Haunschild, P.R. and Sullivan, B.N. (2002), “Learning from complexity: effects of prior accidents and incidents on airlines, learning”, Administrative Science Quarterly, Vol. 47 No. 4, pp. 609-643.
Helmuth, C.A., Craighead, C.W., Connelly, B.L., Collier, D.Y. and Hanna, J.B. (2015), “Supply chain management research: key elements of study design and statistical testing”, Journal of Operations Management, Vol. 36 No. 1, pp. 178-186.
Huang, Y., Han, W. and Macbeth, D.K. (2020), “The complexity of collaboration in supply chain networks, supply chain management”, Supply Chain Management: An International Journal, Vol. 25 No. 3, pp. 393-410.
Hult, G.T.M., Ketchen, D.J. and Slater, S.F. (2004), “Information processing, knowledge development, and strategic supply chain performance”, Academy of Management Journal, Vol. 47 No. 2, pp. 241-253.
Jüttner, U. and Maklan, S. (2011), “Supply chain resilience in the global financial crisis: an empirical study”, Supply Chain Management: An International Journal, Vol. 16 No. 4, pp. 246-259.
Ketokivi, M. and McIntosh, C.N. (2017), “Addressing the endogeneity dilemma in operations management research: theoretical, empirical, and pragmatic considerations”, Journal of Operations Management, Vol. 52 No. 1, pp. 1-14.
Kim, Y., Choi, T.Y., Yan, T. and Dooley, K. (2011), “Structural investigation of supply networks: a social network analysis approach”, Journal of Operations Management, Vol. 29 No. 3, pp. 194-211.
Linnenluecke, M.K. (2017), “Resilience in business and management research: a review of influential publications and a research agenda”, International Journal of Management Reviews, Vol. 19 No. 1, pp. 4-30.
Lu, G., Ding, X., Peng, D.X. and Chuang, H.H.-C. (2018), “Addressing endogeneity in operations management research: recent developments, common problems, and directions for future research”, Journal of Operations Management, Vol. 64 No. 1, pp. 53-64.
McDaniels, T., Chang, S., Cole, D., Mikawoz, J. and Longstaff, H. (2008), “Fostering resilience to extreme events within infrastructure systems: characterizing decision contexts for mitigation and adaptation”, Global Environmental Change, Vol. 18 No. 2, pp. 310-318.
Manhart, P., Summers, J.K. and Blackhurst, J. (2020), “A Meta-analytic review of supply chain risk management: assessing buffering and bridging strategies and firm performance”, Journal of Supply Chain Management, Vol. 56 No. 3, pp. 66-87.
Marrone, J.A. (2010), “Team boundary spanning: a multilevel review of past research and proposals for the future”, Journal of Management, Vol. 36 No. 4, pp. 911-940.
Marrone, J.A., Tesluk, P.E. and Carson, J.B. (2007), “A multilevel investigation of antecedents and consequences of team member boundary-spanning behavior”, Academy of Management Journal, Vol. 50 No. 6, pp. 1423-1439.
Mattsson, L.G. and Jenelius, E. (2015), “Vulnerability and resilience of transport systems – a discussion of recent research”, Transportation Research Part A: Policy and Practice, Vol. 81, pp. 16-34.
Melnyk, S.A., Rodrigues, A. and Ragatz, G.L. (2009), “Using simulation to investigate supply chain disruptions”, in Zsidisin, G.A. and Ritchie, B. (Eds), Supply Chain Risk: A Handbook of Assessment, Management, and Performance, Springer, Boston, MA, pp. 103-122.
Miemczyk, J., Johnsen, T.E. and Macquet, M. (2012), “Sustainable purchasing and supply management: a structured literature review of definitions and measures at the dyad, chain and network levels”, Supply Chain Management: An International Journal, Vol. 17 No. 5, pp. 478-496.
Ouyang, M. (2014), “Review on modeling and simulation of interdependent critical infrastructure systems”, Reliability Engineering & System Safety, Vol. 121, pp. 43-60.
Pescaroli, G. and Kelman, I. (2017), “How critical infrastructure orients international relief in cascading disasters”, Journal of Contingencies and Crisis Management, Vol. 25 No. 2, pp. 56-67.
Pournader, M., Rotaru, K., Kach, A.P. and Hajiagha, S.H.R. (2016), “An analytical model for system-wide and tier-specific assessment of resilience to supply chain risks, supply chain management”, Supply Chain Management: An International Journal, Vol. 21 No. 5, pp. 589-609.
Quick, K.S. and Feldman, M.S. (2014), “Boundaries as junctures: collaborative boundary work for building efficient resilience”, Journal of Public Administration Research and Theory, Vol. 24 No. 3, pp. 673-695.
Ramaekers, P., Wit, T. and Pouwels, M. (2009), Hoe Druk is Het Nu Werkelijk Op Het Nederlandse Spoor? Het Nederlandse Spoorgebruik in Vergelijking Met de Rest Van de EU-27, Centraal Bureau voor de statistiek (Statistics Netherlands), The Hague.
Rinaldi, S.M., Peerenboom, J.P. and Kelly, T.K. (2001), “Identifying, understanding, and analyzing critical infrastructure interdependencies”, IEEE Control Systems Magazine, Vol. 21 No. 6, pp. 11-25.
Roux-Dufort, C. (2007), “Is crisis management (only) a management of exceptions?”, Journal of Contingencies and Crisis Management, Vol. 15 No. 2, pp. 105-114.
Rudolph, J.W. and Repenning, N.P. (2002), “Disaster dynamics: understanding the role of quantity in organizational collapse”, Administrative Science Quarterly, Vol. 47 No. 1, pp. 1-30.
Sahebjamnia, N., Torabi, S.A. and Mansouri, S.A. (2018), “Building organizational resilience in the face of multiple disruptions”, International Journal of Production Economics, Vol. 197, pp. 63-83.
Sawyerr, E. and Harrison, C. (2020), “Developing resilient supply chains: lessons from high-reliability organisations”, Supply Chain Management: An International Journal, Vol. 25 No. 1, pp. 77-100.
Scholten, K. and Schilder, S. (2015), “The role of collaboration in supply chain resilience”, Supply Chain Management: An International Journal, Vol. 20 No. 4, pp. 471-484.
Scholten, K., Stevenson, M. and Van Donk, D.P. (2020), “Dealing with the unpredictable: supply chain resilience”, International Journal of Operations & Production Management, Vol. 40 No. 1, pp. 1-10.
Tenhiälä, A. and Salvador, F. (2014), “Looking inside glitch mitigation capability: the effect of intraorganizational communication channels”, Decision Sciences, Vol. 45 No. 3, pp. 437-466.
Touboulic, A., Matthews, L. and Marques, L. (2018), “On the road to carbon reduction in a food supply network: a complex adaptive systems perspective”, Supply Chain Management: An International Journal, Vol. 23 No. 4, pp. 313-335.
Transport Committee (2018), “Rail timetable changes: May 2018”, Seventh Report of Session 2017-19, House of Commons, London.
Tukamuhabwa, B.R., Stevenson, M. and Busby, J. (2017), “Supply chain resilience in a developing country context: a case study on the interconnectedness of threats, strategies and outcomes”, Supply Chain Management: An International Journal, Vol. 22 No. 6, pp. 486-505.
Tukamuhabwa, B.R., Stevenson, M., Busby, J. and Zorzini, M. (2015), “Supply chain resilience: definition, review and theoretical foundations for further study”, International Journal of Production Research, Vol. 53 No. 18, pp. 5592-5623.
Tushman, M.L. and Nadler, D.A. (1978), “Information processing as an integrating concept in organizational design”, Academy of Management Review, Vol. 3 No. 3, pp. 613-624.
van der Vegt, G.S., Essens, P., Wahlstrom, M. and George, G. (2015), “Managing risk and resilience”, Academy of Management Journal, Vol. 58 No. 4, pp. 971-980.
Van Eeten, M., Nieuwenhuijs, A., Luiijf, E., Klaver, M. and Cruz, E. (2011), “The state and the threat of cascading failure across critical infrastructures: the implications of empirical evidence from media incident reports”, Public Administration, Vol. 89 No. 2, pp. 381-400.
Weick, K.E. and Sutcliffe, K.M. (2011), Managing the Unexpected: Resilient Performance in an Age of Uncertainty, 2nd ed., John Wiley & Sons, San Francisco.
Wildavsky, A.B. (1988), Searching for Safety, Transaction Publishers, New Brunswick, NJ.
Wu, B., Tang, A. and Wu, J. (2016), “Modeling cascading failures in interdependent infrastructures under terrorist attacks”, Reliability Engineering & System Safety, Vol. 147, pp. 1-8.
Zhang, F., Welch, E.W. and Miao, Q. (2018), “Public organization adaptation to extreme events: mediating role of risk perception”, Journal of Public Administration Research and Theory, Vol. 28 No. 3, pp. 371-387.
Zheng, B. (2000), “Summarizing the goodness of fit of generalized linear models for longitudinal data”, Statistics in Medicine, Vol. 19 No. 10, pp. 1265-1275.
Zobel, C.W. and Khansa, L. (2014), “Characterizing multi-event disaster resilience”, Computers & Operations Research, Vol. 42, pp. 83-94.